Prediction Of Nitration Sites Based On Fcbf Method And Stacking Ensem-Ble Model
CURRENT PROTEOMICS(2021)
摘要
Background: Nitration is an important Post-Translational Modification (PTM) occur-ring on the tyrosine residues of proteins. The occurrence of protein tyrosine nitration under disease conditions is inevitable and represents a shift from the signal transducing physiological actions of -NO to oxidative and potentially pathogenic pathways. Abnormal protein nitration modification can lead to serious human diseases, including neurodegenerative diseases, acute respiratory distress, or-gan transplant rejection and lung cancer. Objective: It is necessary and important to identify the nitration sites in protein sequences. Predict-ing which tyrosine residues in the protein sequence are nitrated and which are not is of great signifi-cance for the study of nitration mechanism and related diseases. Methods: In this study, a prediction model of nitration sites based on the over-under sampling strat-egy and the FCBF method was proposed by stacking ensemble learning and fusing multiple fea-tures. Firstly, the protein sequence sample was encoded by 2701-dimensional fusion features (PseAAC, PSSM, AAIndex, CKSAAP, Disorder). Secondly, the ranked feature set was generated by the FCBF method according to the symmetric uncertainty metric. Thirdly, in the process of mod-el training, the over-and under-sampling technique was used to tackle the imbalanced dataset. Fi-nally, the Incremental Feature Selection (IFS) method was adopted to extract an optimal classifier based on 10-fold cross-validation. Results and Conclusion: Results show that the model has significant performance advantages in in-dicators such as MCC, Recall and F1-score, no matter in what way the comparison was conducted with other classifiers on the independent test set, or made by cross-validation with single-type fea-ture or with fusion-features on the training set. By integrating the FCBF feature ranking methods, over-and under-sampling technique and a stacking model composed of multiple base classifiers, an effective prediction model for nitration PTM sites was built, which can achieve a better recall rate when the ratio of positive and negative samples is highly imbalanced.
更多查看译文
关键词
PTM (post-translational modification), nitration site, FCBF (fast correlation-based filter), stacking model, over- and under- sampling, Incremental Feature Selection (IFS)
AI 理解论文
溯源树
样例
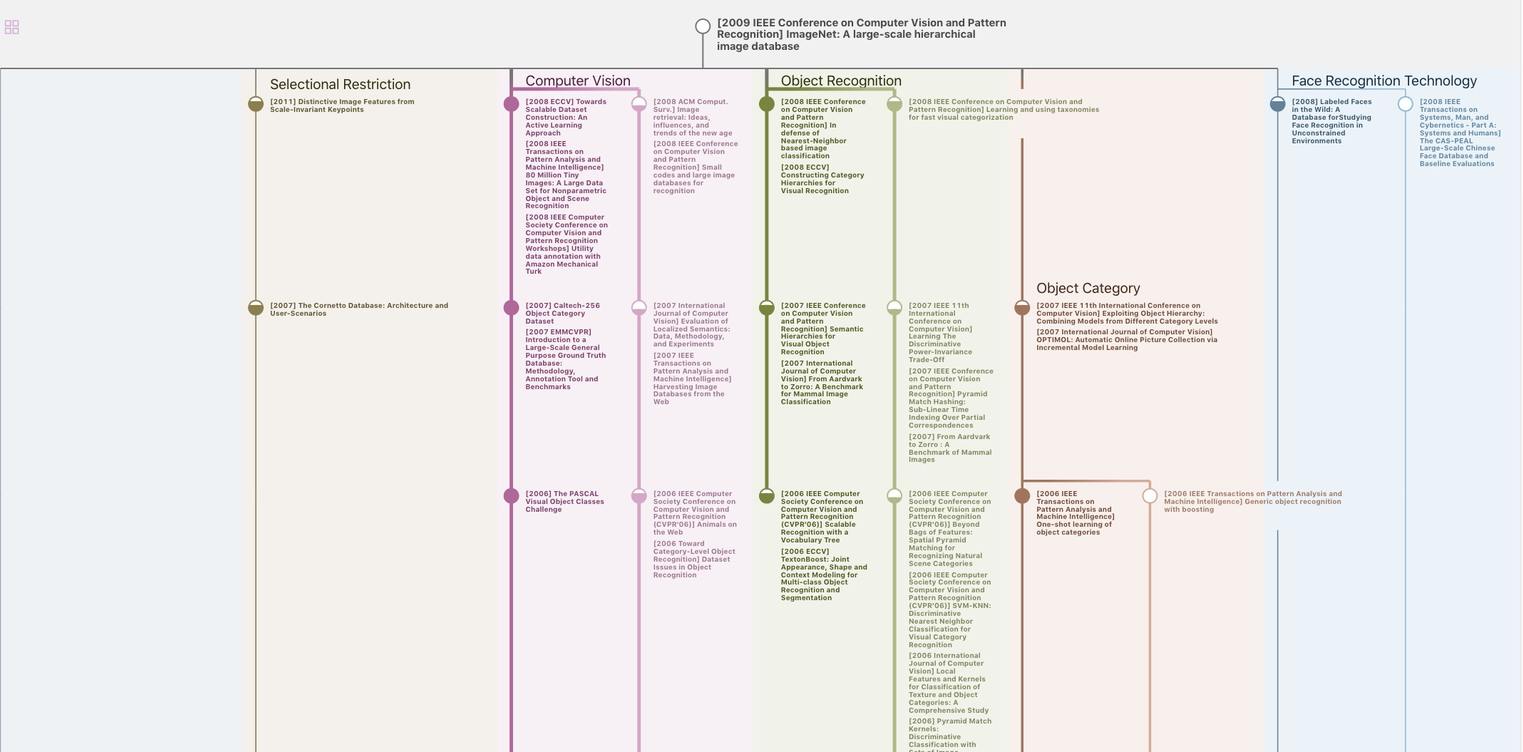
生成溯源树,研究论文发展脉络
Chat Paper
正在生成论文摘要