Non-Uniform Low-Light Image Enhancement Via Non-Local Similarity Decomposition Model
SIGNAL PROCESSING-IMAGE COMMUNICATION(2021)
摘要
Many low-light image enhancement methods ignore the characteristic of non-uniform low-light images, thereby causing the enhanced results to lose their naturalness. In this paper, a non-local similarity decomposition model based on the Retinex theory is proposed to obtain high-quality enhanced results for non-uniform low-light images. For the reflectance layer, we explore a weighted L1-norm regularization according to the similarity measure of non-local patches. The similarity measure contains color, contrast and texture information, which are the intrinsic features of images. For the illumination layer, we introduce a weight matrix to constrain its gradient based on the edge information of non-uniform low-light images. By applying these constraints, it is more precise to estimate the reflectance and illumination layers simultaneously. Finally, the decomposed illumination is further adjusted. Furthermore, an alternating direction method of multipliers and preconditioned conjugate gradient are utilized to accelerate the solution of the proposed model. Experimental results demonstrate that our method is better for enhancing non-uniform low-light image both in subjective and objective assessments.
更多查看译文
关键词
Non-uniform low-light image enhancement, Similarity measure, Non-local similarity decomposition, Edge information, Reflectance and illumination
AI 理解论文
溯源树
样例
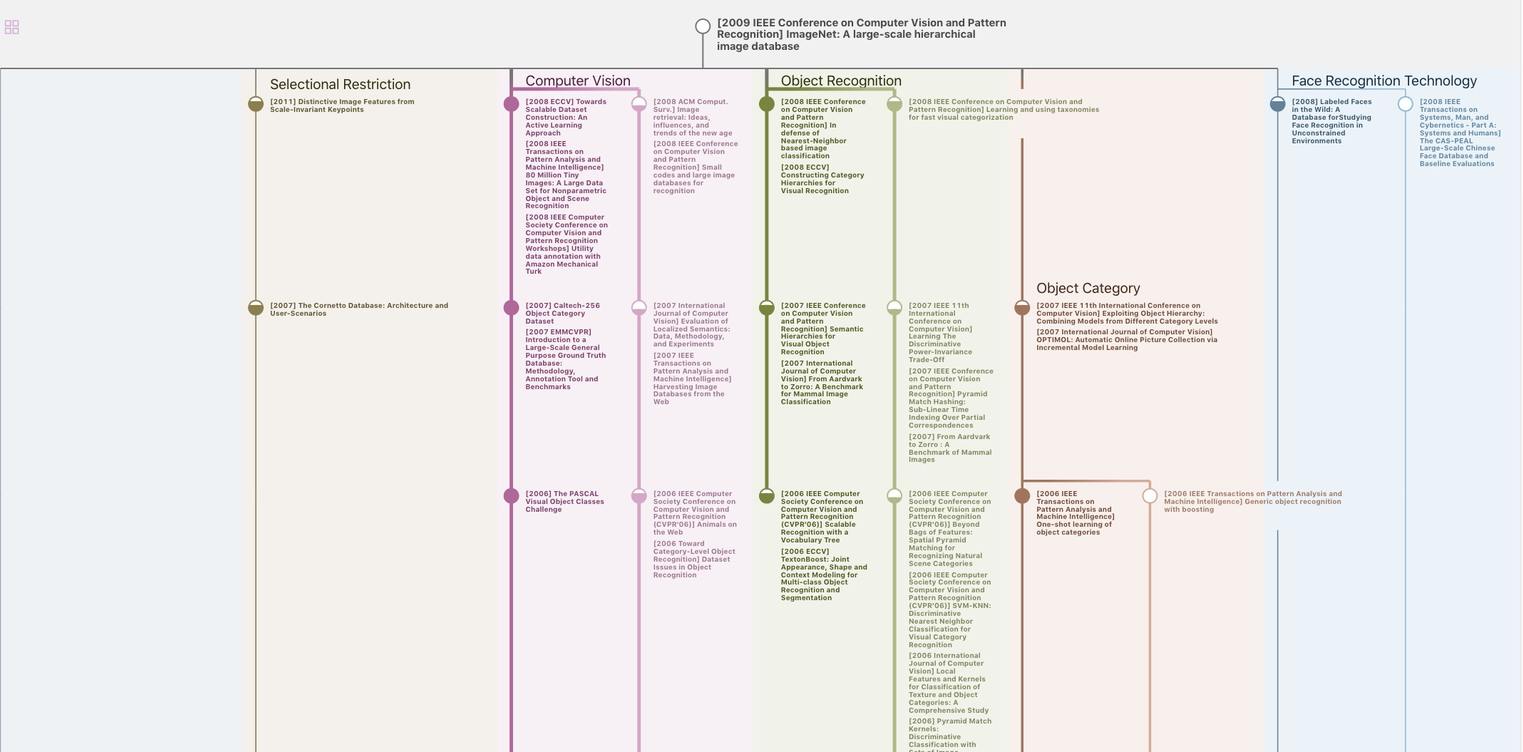
生成溯源树,研究论文发展脉络
Chat Paper
正在生成论文摘要