High-Order Triplet Crf-Pcanet For Unsupervised Segmentation Of Sar Image
IGARSS 2020 - 2020 IEEE INTERNATIONAL GEOSCIENCE AND REMOTE SENSING SYMPOSIUM(2020)
摘要
In this paper, we combine the modeling power of conditional random fields (CRF) model with the representation-learning ability of principal component analysis network (PCANet), and propose a high-order triplet CRF model, named as HOTCRF-PCANet, for unsupervised synthetic aperture radar (SAR) image segmentation. HOTCRF-PCANet introduces an auxiliary field to explicitly regulate label interactions of complex SAR image. In the label and auxiliary fields, HOTCRF-PCANet defines a discrete quadrilateral pairwise Markov fields (DQPMF) model, and thus constructs a high-order DQPMF potential to model the high-order label interactions in an unsupervised way. Additionally, HOTCRF-PCANet uses a product-of-expert (POE) potential to enforce the regions' labeling consistency for pixels within the weak-structured region. Moreover, HOTCRF-PCANet modifies PCANet into an unsupervised mode, i.e. UPCANet, automatically learns rich features of SAR image and constructs an UPCANet-based unary potential to predict the local class probability. The effectiveness of HOTCRF-PCANet is demonstrated by the application to the unsupervised segmentation of simulated and real SAR images.
更多查看译文
关键词
Nonstationary SAR image, Unsupervised segmentation, High-order label interactions, Deep Learning, Conditional random fields
AI 理解论文
溯源树
样例
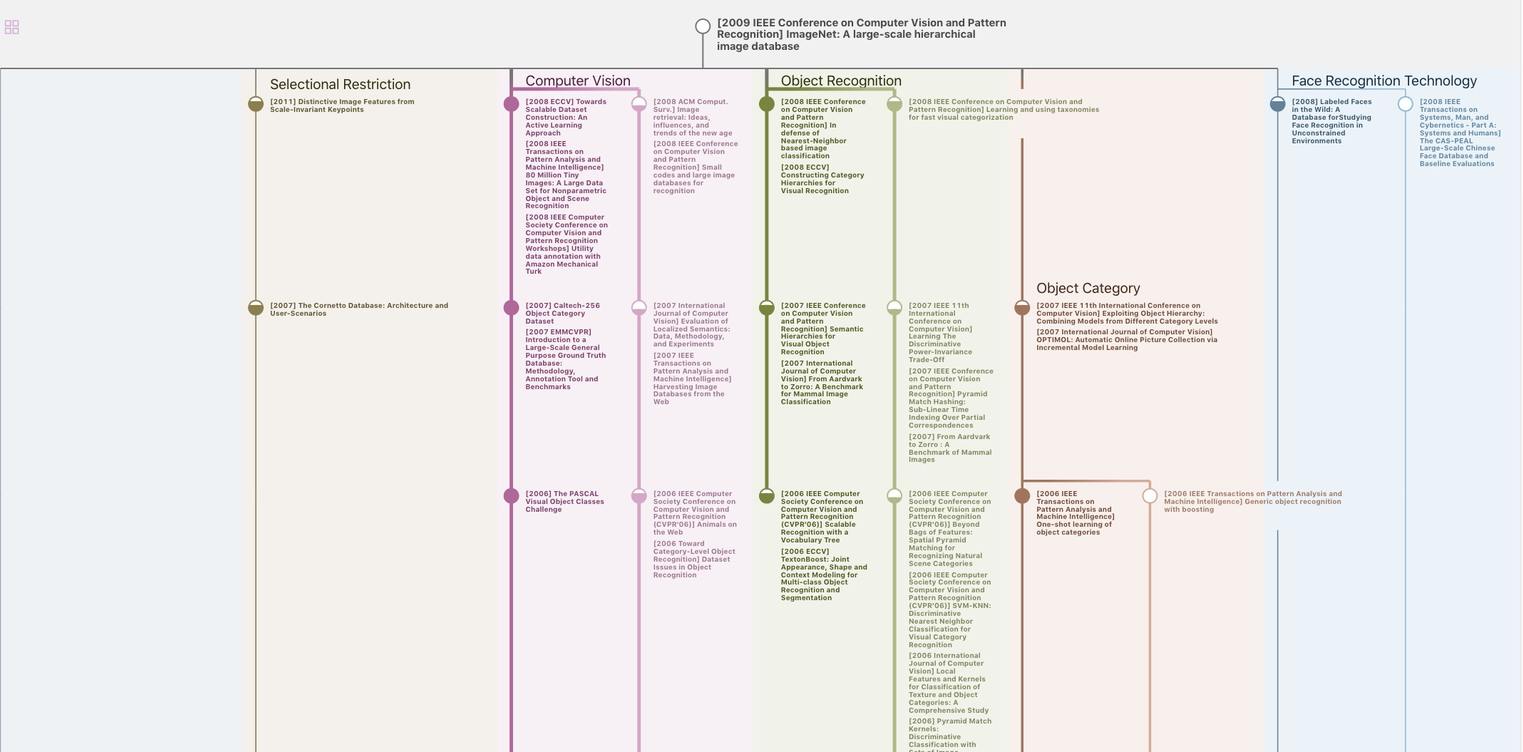
生成溯源树,研究论文发展脉络
Chat Paper
正在生成论文摘要