Estimation Of Significant Wave Heights From Ascat Scatterometer Data Via Deep Learning Network
REMOTE SENSING(2021)
摘要
Sea state estimation from wide-swath and frequent-revisit scatterometers, which are providing ocean winds in the routine, is an attractive challenge. In this study, state-of-the-art deep learning technology is successfully adopted to develop an algorithm for deriving significant wave height from Advanced Scatterometer (ASCAT) aboard MetOp-A. By collocating three years (2016-2018) of ASCAT measurements and WaveWatch III sea state hindcasts at a global scale, huge amount data points (>8 million) were employed to train the multi-hidden-layer deep learning model, which has been established to map the inputs of thirteen sea state related ASCAT observables into the wave heights. The ASCAT significant wave height estimates were validated against hindcast dataset independent on training, showing good consistency in terms of root mean square error of 0.5 m under moderate sea condition (1.0-5.0 m). Additionally, reasonable agreement is also found between ASCAT derived wave heights and buoy observations from National Data Buoy Center for the proposed algorithm. Results are further discussed with respect to sea state maturity, radar incidence angle along with the limitations of the model. Our work demonstrates the capability of scatterometers for monitoring sea state, thus would advance the use of scatterometers, which were originally designed for winds, in studies of ocean waves.
更多查看译文
关键词
Advanced Scatterometer (ASCAT), significant wave height, WaveWatch III, deep learning, multi-hidden-layer neural network
AI 理解论文
溯源树
样例
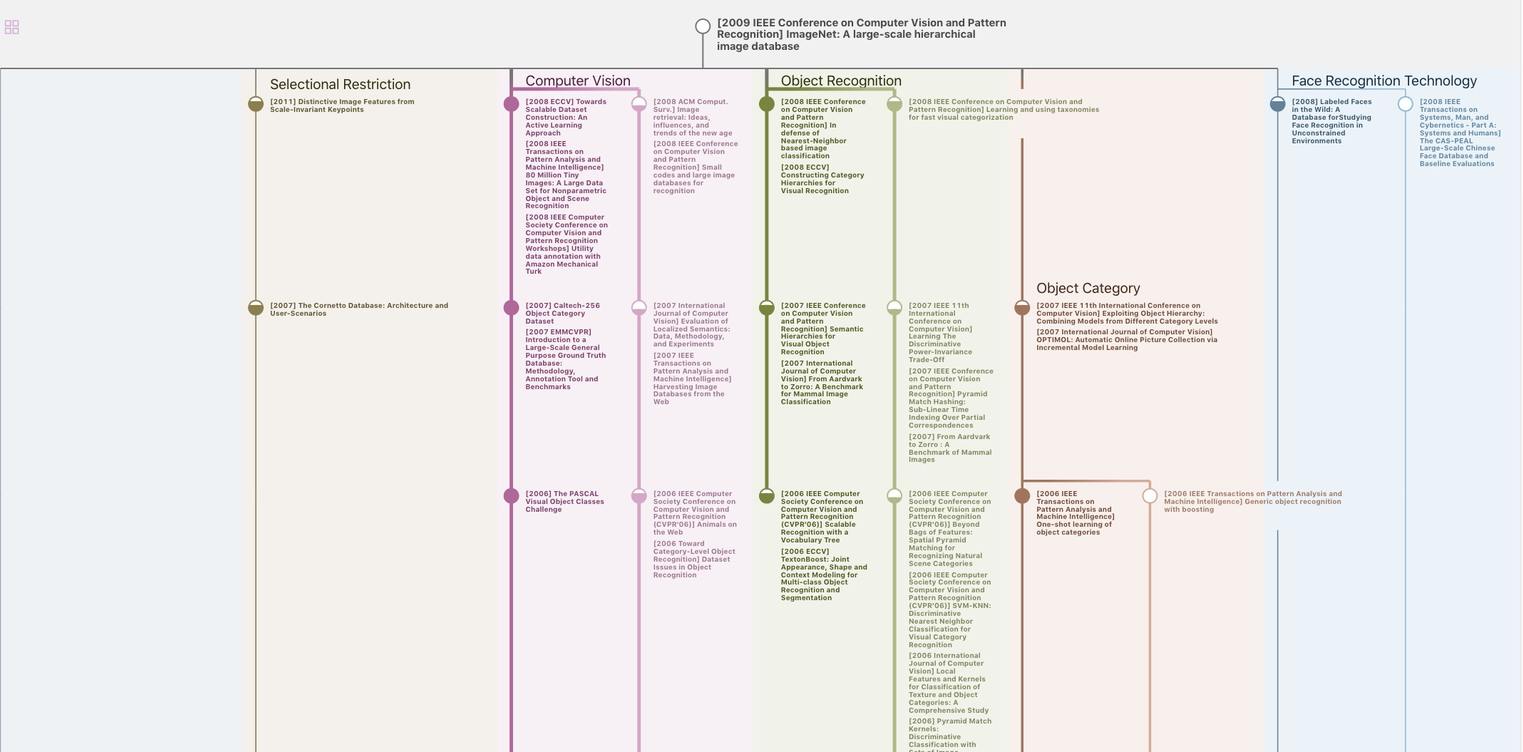
生成溯源树,研究论文发展脉络
Chat Paper
正在生成论文摘要