Introducing The Hidden Neural Markov Chain Framework
ICAART: PROCEEDINGS OF THE 13TH INTERNATIONAL CONFERENCE ON AGENTS AND ARTIFICIAL INTELLIGENCE - VOL 2(2021)
摘要
Nowadays, neural network models achieve state-of-the-art results in many areas as computer vision or speech processing. For sequential data, especially for Natural Language Processing (NLP) tasks, Recurrent Neural Networks (RNNs) and their extensions, the Long Short Term Memory (LSTM) network and the Gated Recurrent Unit (GRU), are among the most used models, having a "term-to-term" sequence processing. However, if many works create extensions and improvements of the RNN, few have focused on developing other ways for sequential data processing with neural networks in a "term-to-term" way. This paper proposes the original Hidden Neural Markov Chain (HNMC) framework, a new family of sequential neural models. They are not based on the RNN but on the Hidden Markov Model (HMM), a probabilistic graphical model. This neural extension is possible thanks to the recent Entropic Forward-Backward algorithm for HMM restoration. We propose three different models: the classic HNMC, the HNMC2, and the HNMC-CN. After describing our models' whole construction, we compare them with classic RNN and Bidirectional RNN (BiRNN) models for some sequence labeling tasks: Chunking, Part-Of-Speech Tagging, and Named Entity Recognition. For every experiment, whatever the architecture or the embedding method used, one of our proposed models has the best results. It shows this new neural sequential framework's potential, which can open the way to new models, and might eventually compete with the prevalent BiLSTM and BiGRU.
更多查看译文
关键词
Hidden Markov Model, Entropic Forward-Backward, Recurrent Neural Network, Sequence Labeling, Hidden Neural Markov Chain
AI 理解论文
溯源树
样例
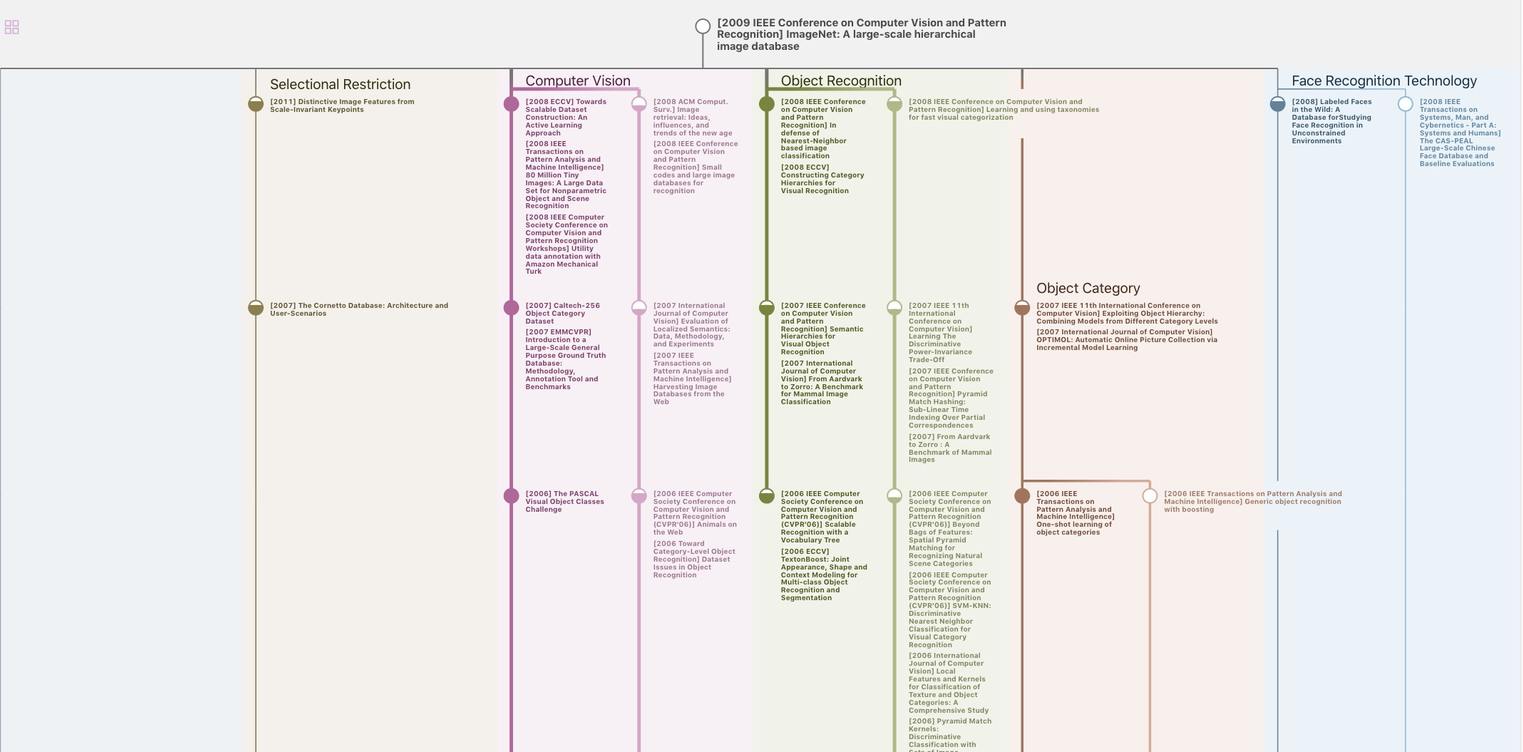
生成溯源树,研究论文发展脉络
Chat Paper
正在生成论文摘要