Confidently Comparing Estimators with the c-value
arxiv(2022)
摘要
Modern statistics provides an ever-expanding toolkit for estimating unknown parameters. Consequently, applied statisticians frequently face a difficult decision: retain a parameter estimate from a familiar method or replace it with an estimate from a newer or more complex one. While it is traditional to compare estimates using risk, such comparisons are rarely conclusive in realistic settings. In response, we propose the "c-value" as a measure of confidence that a new estimate achieves smaller loss than an old estimate on a given dataset. We show that it is unlikely that a large c-value coincides with a larger loss for the new estimate. Therefore, just as a small p-value supports rejecting a null hypothesis, a large c-value supports using a new estimate in place of the old. For a wide class of problems and estimates, we show how to compute a c-value by first constructing a data-dependent high-probability lower bound on the difference in loss. The c-value is frequentist in nature, but we show that it can provide validation of shrinkage estimates derived from Bayesian models in real data applications involving hierarchical models and Gaussian processes.
更多查看译文
关键词
Decision theory,Empirical Bayes,Model selection,Normal means,Shrinkage
AI 理解论文
溯源树
样例
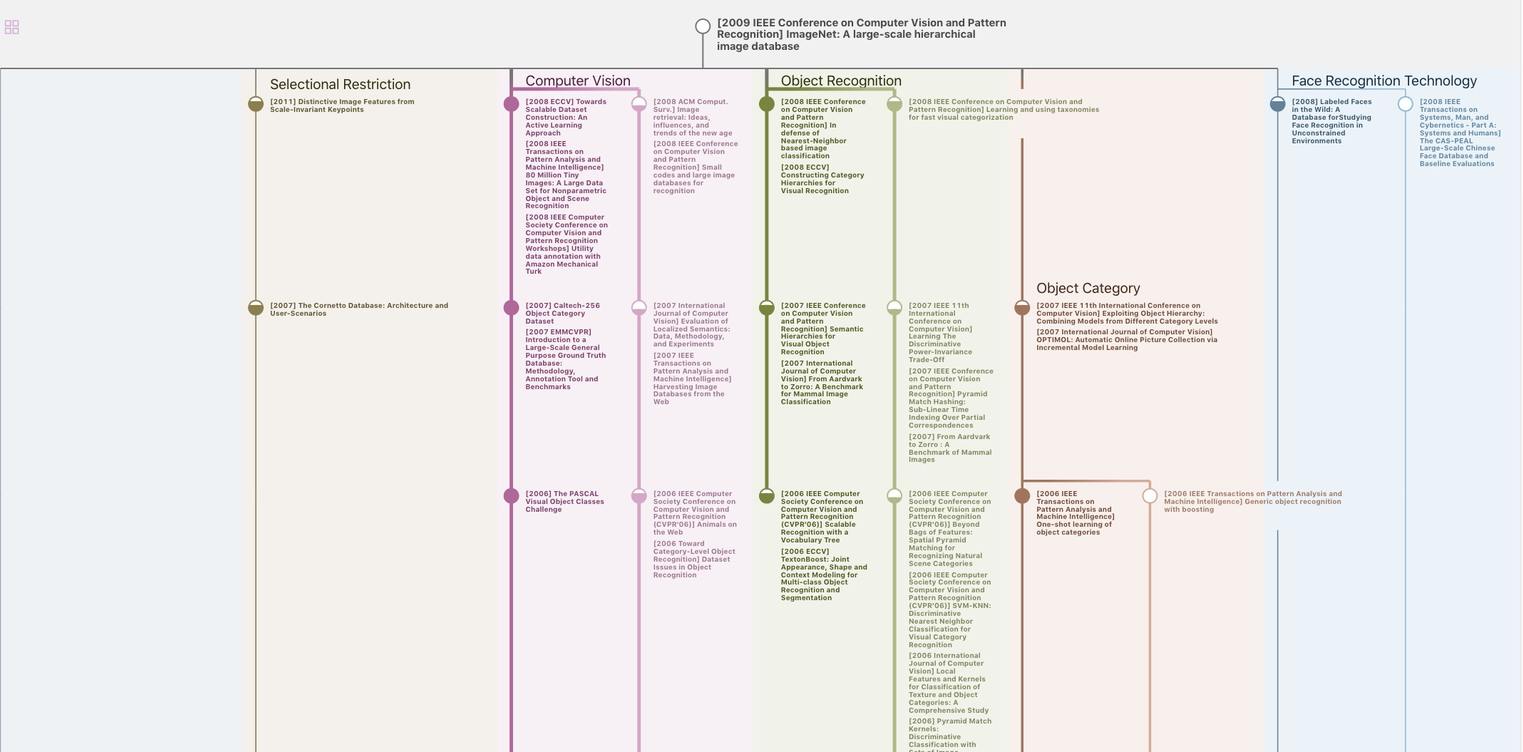
生成溯源树,研究论文发展脉络
Chat Paper
正在生成论文摘要