Optimising Long-Term Outcomes using Real-World Fluent Objectives: An Application to Football
AAMAS(2021)
摘要
In this paper, we present a novel approach for optimising long-term tactical and strategic decision-making in football (soccer) by encapsulating events in a league environment across a given time frame. We model the teams' objectives for a season and track how these evolve as games unfold to give a fluent objective that can aid in decision-making games. We develop Markov chain Monte Carlo and deep learning-based algorithms that make use of the fluent objectives in order to learn from prior games and other games in the environment and increase the teams' long-term performance. Simulations of our approach using real-world datasets from 760 matches shows that by using optimised tactics with our fluent objective and prior games, we can on average increase teams mean expected finishing distribution in the league by up to 35.6%.
更多查看译文
关键词
football,outcomes,long-term long-term,real-world
AI 理解论文
溯源树
样例
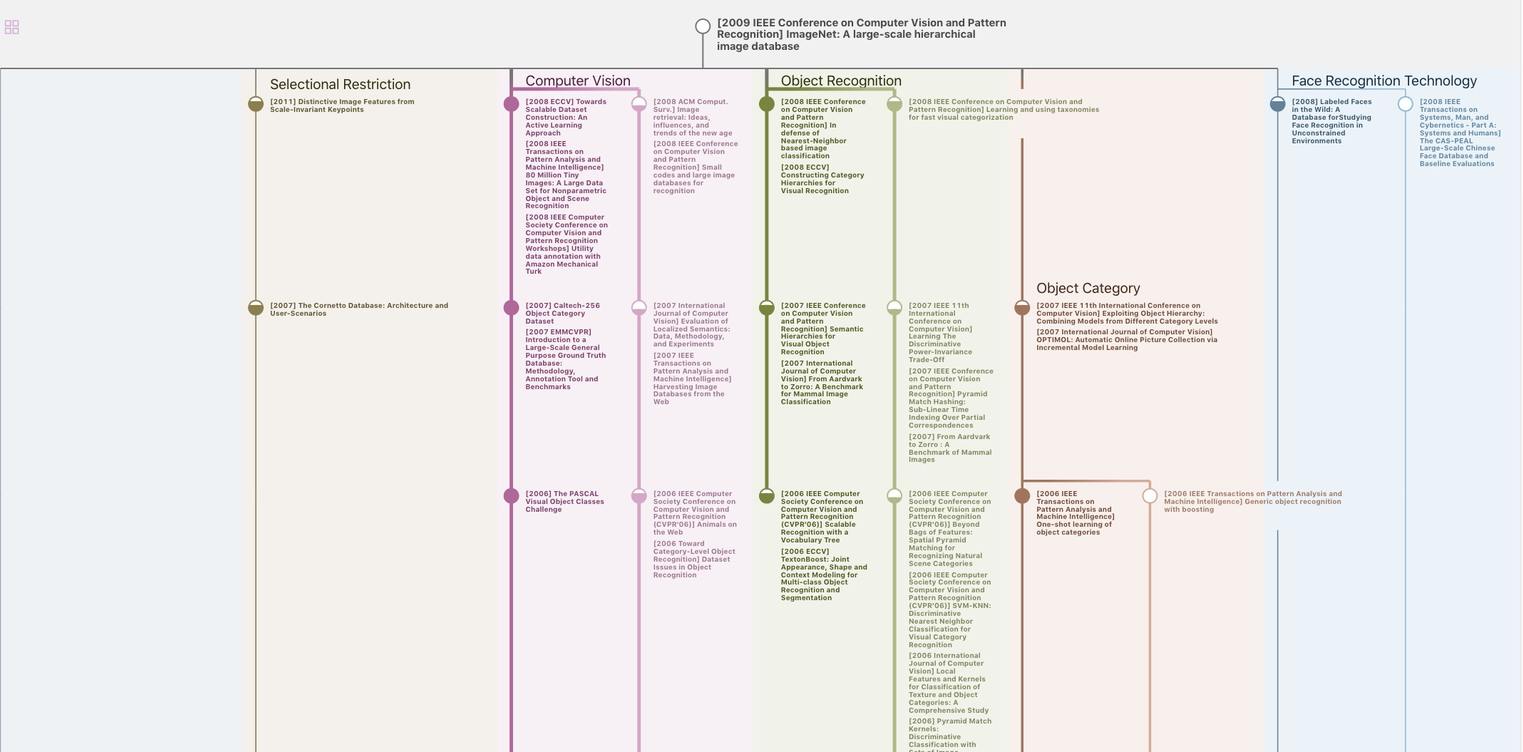
生成溯源树,研究论文发展脉络
Chat Paper
正在生成论文摘要