Harnessing Tensor Structures—Multi-Mode Reservoir Computing and Its Application in Massive MIMO
IEEE Transactions on Wireless Communications(2022)
摘要
In this paper, we introduce a new neural network (NN) structure, multi-mode reservoir computing (Multi-Mode RC). It inherits the dynamic mechanism of RC and processes the forward path and loss optimization of the NN using tensor as the underlying data format. Multi-Mode RC exhibits less complexity compared to conventional RC structures (e.g. single-mode RC), and offers comparable generalization performance to its single-mode counterpart. Furthermore, we introduce an alternating least square-based learning algorithm as well as the associated theoretical analysis for Multi-Mode RC. The result can be utilized to guide the configuration of NN parameters to sufficiently circumvent over-fitting issues. As a key application, we consider the symbol detection task in multiple-input-multiple-output (MIMO) orthogonal-frequency-division-multiplexing (OFDM) systems with massive MIMO employed at the base stations (BSs). Thanks to the tensor structure of massive MIMO-OFDM signals, our online learning-based symbol detection method generalizes well in terms of bit error rate even using a limited online training set. Evaluation results suggest that the Multi-Mode RC-based learning framework can efficiently and effectively combat practical constraints of wireless systems (i.e. channel state information (CSI) errors and hardware non-linearity) to enable robust and adaptive communications over the air.
更多查看译文
关键词
Reservoir computing,neural networks,online training,massive MIMO,5G,imperfect CSI,and non-linearity
AI 理解论文
溯源树
样例
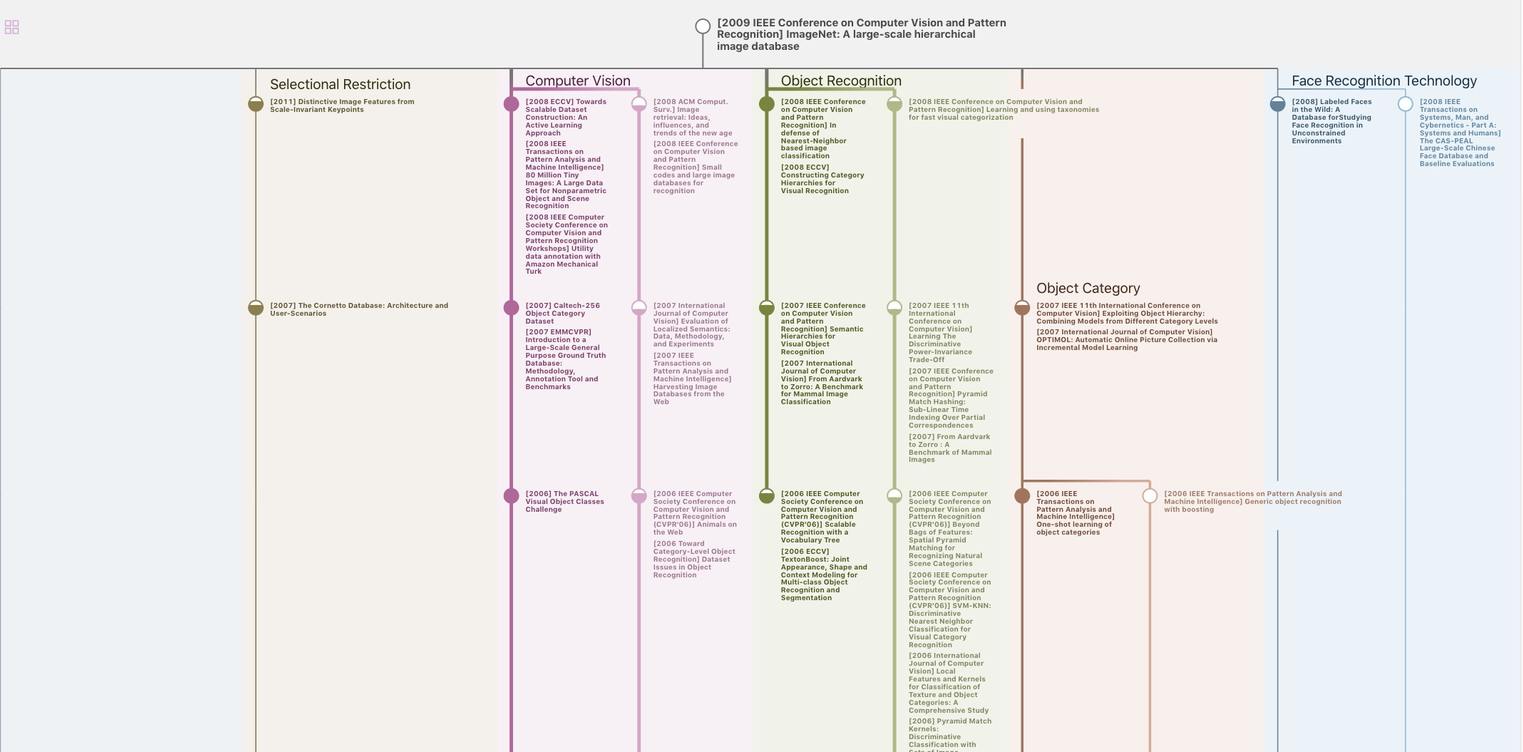
生成溯源树,研究论文发展脉络
Chat Paper
正在生成论文摘要