Finite-Sample Analysis Of Off-Policy Natural Actor-Critic Algorithm
INTERNATIONAL CONFERENCE ON MACHINE LEARNING, VOL 139(2021)
摘要
In this paper, we provide finite-sample convergence guarantees for an off-policy variant of the natural actor-critic (NAC) algorithm based on Importance Sampling. In particular, we show that the algorithm converges to a global optimal policy with a sample complexity of O(epsilon(-3) log(2) (1/epsilon)) under an appropriate choice of stepsizes. In order to overcome the issue of large variance due to Importance Sampling, we propose the Q-trace algorithm for the critic, which is inspired by the V-trace algorithm (Espeholt et al., 2018). This enables us to explicitly control the bias and variance, and characterize the trade-off between them. As an advantage of off-policy sampling, a major feature of our result is that we do not need any additional assumptions, beyond the ergodicity of the Markov chain induced by the behavior policy.
更多查看译文
关键词
finite-sample finite-sample analysis,off-policy,actor-critic
AI 理解论文
溯源树
样例
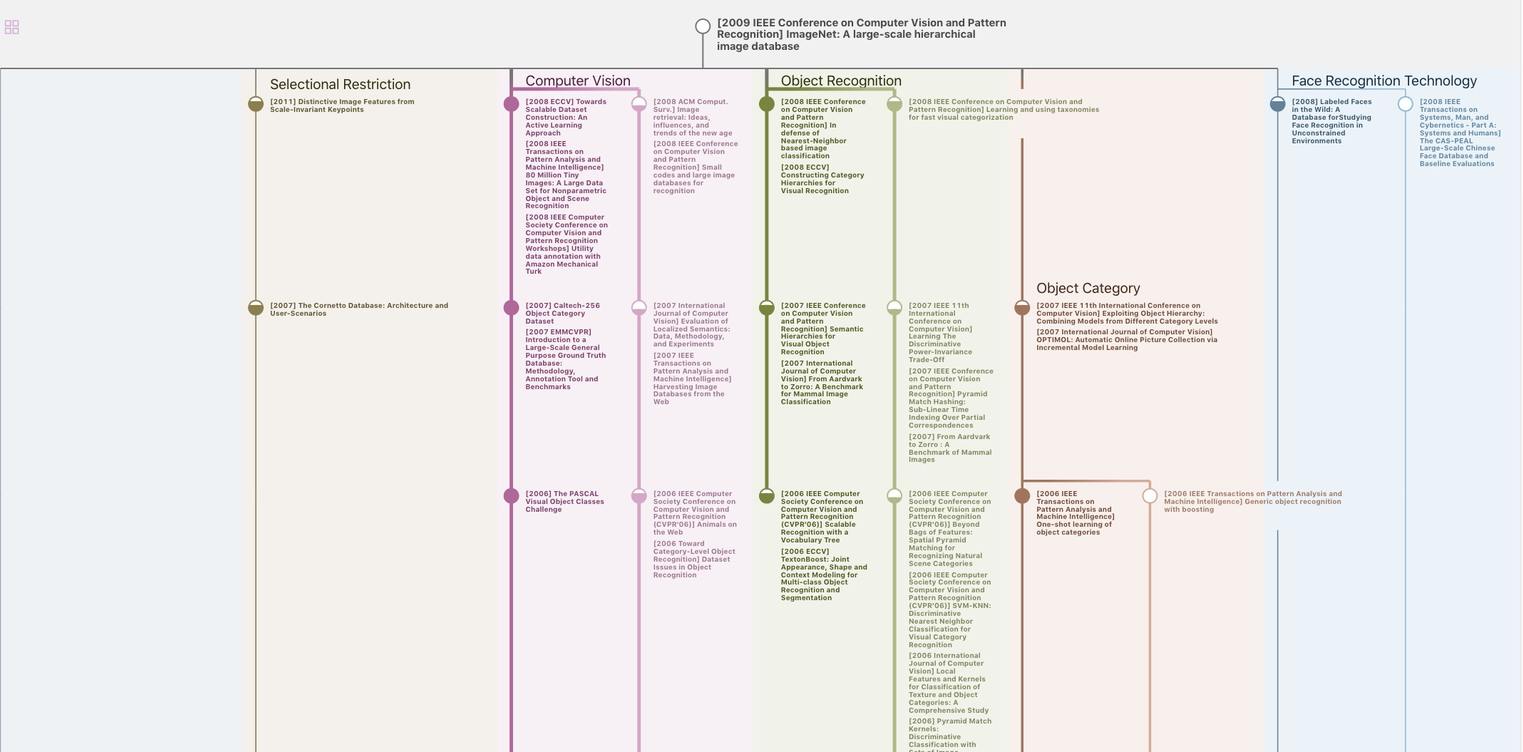
生成溯源树,研究论文发展脉络
Chat Paper
正在生成论文摘要