Interpretable Spatiotemporal Deep Learning Model For Traffic Flow Prediction Based On Potential Energy Fields
20TH IEEE INTERNATIONAL CONFERENCE ON DATA MINING (ICDM 2020)(2020)
摘要
Traffic flow prediction is of great importance in traffic management and public safety, but is challenging due to the complex spatial-temporal dependencies as well as temporal dynamics. Existing work either focuses on traditional statistical models, which have limited prediction accuracy, or relies on black-box deep learning models, which have superior prediction accuracy but are hard to interpret. In contrast, we propose a novel interpretable spatiotemporal deep learning model for traffic flow prediction. Our main idea is to model the physics of traffic flow through a number of latent Spatio-Temporal Potential Energy Fields (ST-PEFs), similar to water flow driven by the gravity field. We develop a Wind field Decomposition (WD) algorithm to decompose traffic flow into poly-tree components so that ST-PEFs can be established. We then design a spatiotemporal deep learning model for the ST-PEFs, which consists of a temporal component (modeling the temporal correlation) and a spatial component (modeling the spatial dependencies). To the best of our knowledge, this is the first work that make traffic flow prediction based on ST-PEFs. Experimental results on real-world traffic datasets show the effectiveness of our model compared to the existing methods. A case study confirms our model interpretability.
更多查看译文
关键词
Potential Energy Fields, Spatiotemporal Model, Interpretable Prediction, Deep Learning
AI 理解论文
溯源树
样例
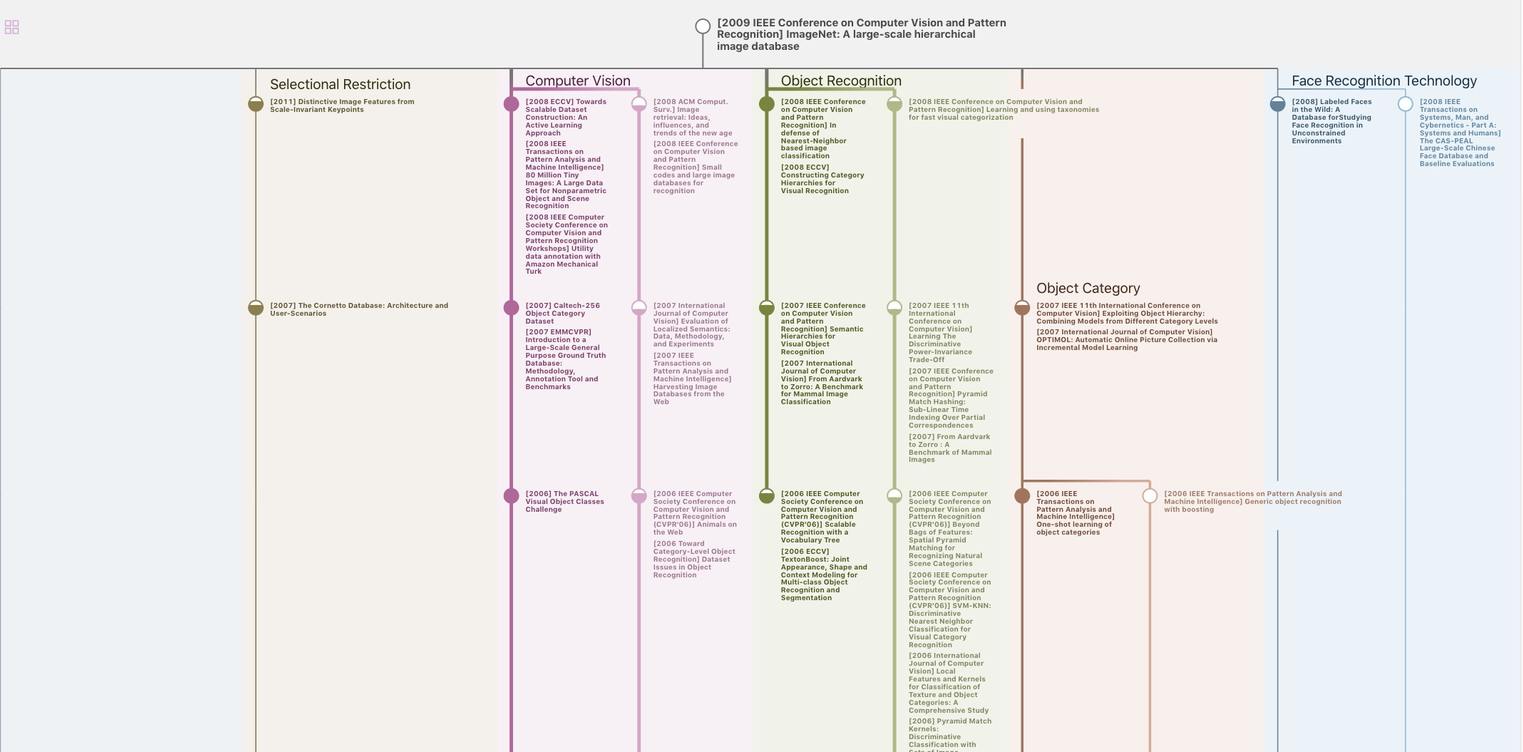
生成溯源树,研究论文发展脉络
Chat Paper
正在生成论文摘要