Practical Verification of Neural Network Enabled State Estimation System for Robotics.
IROS(2020)
摘要
We study for the first time the verification problem on learning-enabled state estimation systems for robotics, which use Bayes filter for localisation, and use deep neural network to process sensory input into observations for the Bayes filter. Specifically, we are interested in a robustness property of the systems: given a certain ability to an adversary for it to attack the neural network without being noticed, whether or not the state estimation system is able to function with only minor loss of localisation precision? For verification purposes, we reduce the state estimation systems to a novel class of labelled transition systems with payoffs and partial order relations, and formally express the robustness property as a constrained optimisation objective. Based on this, practical verification algorithms are developed. As a major case study, we work with a real-world dynamic tracking system that uses a Kalman filter (a special case of the Bayes filter) to localise and track a ground vehicle. Its perception system, based on convolutional neural networks, processes a high-resolution Wide Area Motion Imagery (WAMI) data stream. Experimental results show that our algorithms can not only verify the robustness of the WAMI tracking system but also provide useful counterexamples.
更多查看译文
关键词
neural network enabled state estimation system,robotics,verification problem,learning-enabled state estimation systems,Bayes filter,deep neural network,robustness property,localisation precision,verification purposes,labelled transition systems,practical verification algorithms,real-world dynamic tracking system,Kalman filter,perception system,convolutional neural networks,WAMI tracking system
AI 理解论文
溯源树
样例
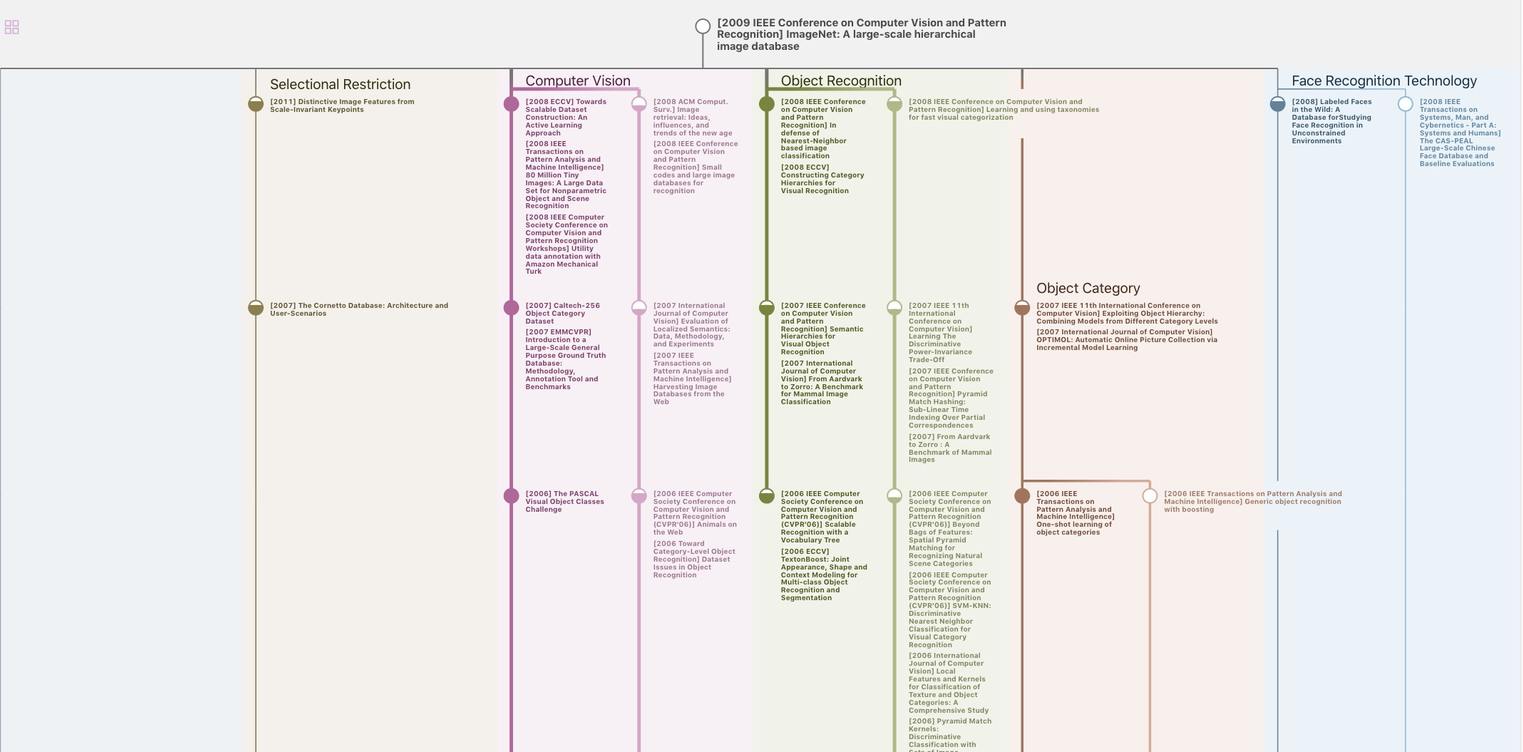
生成溯源树,研究论文发展脉络
Chat Paper
正在生成论文摘要