An Online Training Method for Augmenting MPC with Deep Reinforcement Learning
IROS(2020)
摘要
Recent breakthroughs both in reinforcement learning and trajectory optimization have made significant advances towards real world robotic system deployment. Reinforcement learning (RL) can be applied to many problems without needing any modeling or intuition about the system, at the cost of high sample complexity and the inability to prove any metrics about the learned policies. Trajectory optimization (TO) on the other hand allows for stability and robustness analyses on generated motions and trajectories, but is only as good as the often over-simplified derived model, and may have prohibitively expensive computation times for real-time control, for example in contact rich environments. This paper seeks to combine the benefits from these two areas while mitigating their drawbacks by (1) decreasing RL sample complexity by using existing knowledge of the problem with real-time optimal control, and (2) allowing online policy deployment at any point in the training process by using the TO (MPC) as a baseline or worst-case scenario action, while continuously improving the combined learned-optimized policy with deep RL. This method is evaluated on tasks of successively navigating a car model to a series of goal destinations over slippery terrains as fast as possible, in which drifting will allow the system to more quickly change directions while maintaining high speeds.
更多查看译文
关键词
online training method,MPC,deep reinforcement learning,trajectory optimization,stability,robustness analyses,motion generation,over-simplified derived model,real-time control,contact rich environments,RL sample complexity,real-time optimal control,online policy deployment,learned-optimized policy,car model,robotic system deployment,navigation
AI 理解论文
溯源树
样例
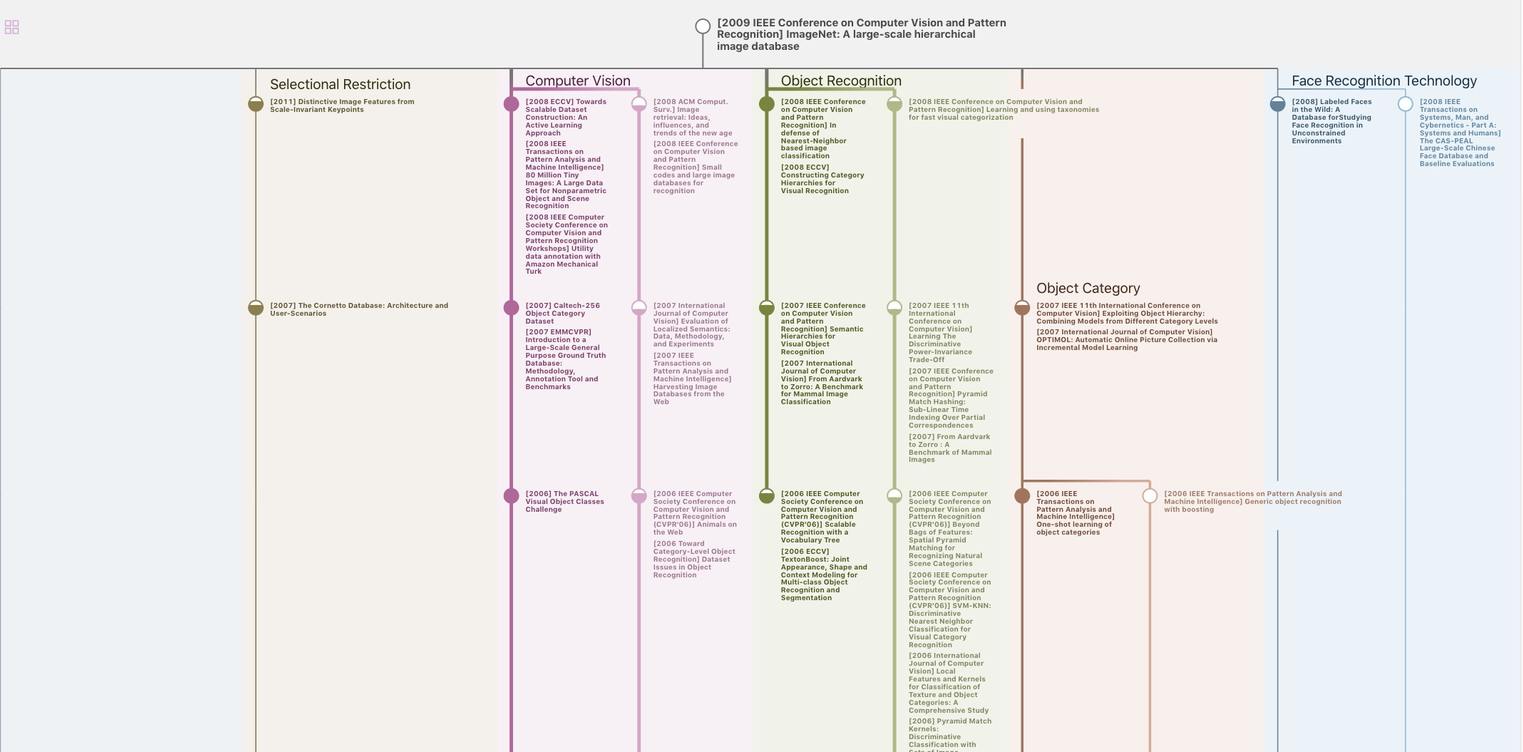
生成溯源树,研究论文发展脉络
Chat Paper
正在生成论文摘要