Deep Unfolding With Weighted ℓ ₂ Minimization for Compressive Sensing
IEEE Internet of Things Journal(2021)
摘要
Compressive sensing (CS) aims to accurately reconstruct high-dimensional signals from a small number of measurements by exploiting signal sparsity and structural priors. However, signal priors utilized in existing CS reconstruction algorithms rely mainly on hand-crafted design, which often cannot offer the best sparsity-undersampling tradeoff because high-order structural priors of signals are hard to be captured in this manner. In this article, a new recovery guarantee of the unified CS reconstruction model-weighted ℓ
1
minimization (WL1M) is derived, which indicates universal priors could hardly lead to the optimal selection of the weights. Motivated by the analysis, we propose a deep unfolding network for the general WL1M model. The proposed deep unfolding-based WL1M (D-WL1M) integrates universal priors with learning capability so that all of the parameters, including the crucial weights, can be learned from training data. We demonstrate the proposed D-WL1M outperforms several state-of-the-art CS-based methods and deep learning-based methods by a large margin via the experiments on the Caltech-256 image data set.
更多查看译文
关键词
Compressive sensing (CS),iterative soft-thresholding algorithm (ISTA),recurrent neural network (RNN),sequential sparse recovery,weighted ℓ₂ minimization (WL1M)
AI 理解论文
溯源树
样例
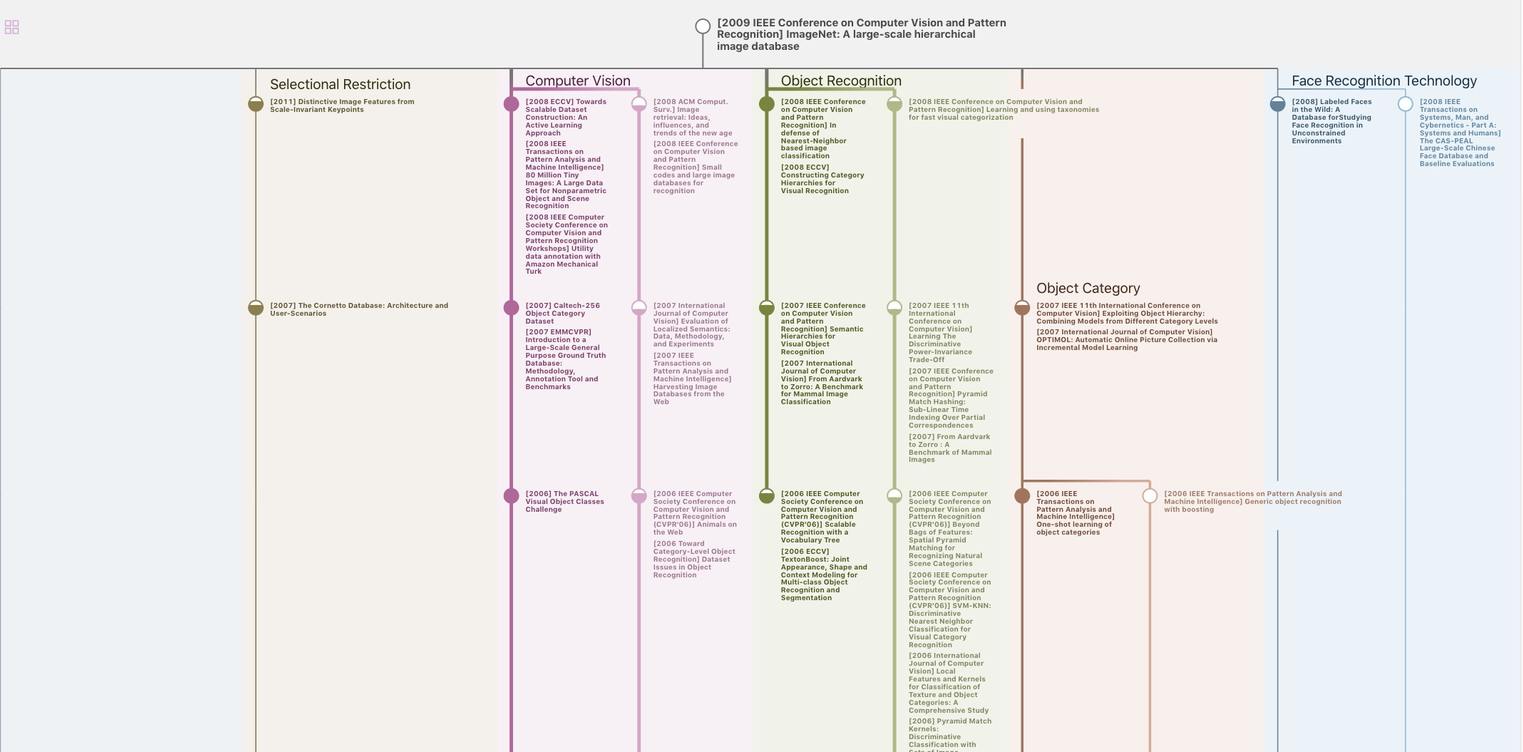
生成溯源树,研究论文发展脉络
Chat Paper
正在生成论文摘要