Comprehensive comparative study of multi-label classification methods
Expert Systems with Applications(2022)
摘要
Multi-label classification (MLC) has recently attracted increasing interest in the machine learning community. Several studies provide surveys of methods and datasets for MLC, and a few provide empirical comparisons of MLC methods. However, they are limited in the number of methods and datasets considered. This paper provides a comprehensive empirical investigation of a wide range of MLC methods on a wealth of datasets from different domains. More specifically, our study evaluates 26 methods on 42 benchmark datasets using 20 evaluation measures. The evaluation methodology used meets the highest literature standards for designing and conducting large-scale, time-limited experimental studies. First, the methods were selected based on their use in the community to ensure a balanced representation of methods across the MLC taxonomy of methods within the study. Second, the datasets cover a wide range of complexity and application domains. The selected evaluation measures assess the predictive performance and efficiency of the methods. The results of the analysis identify RFPCT, RFDTBR, ECCJ48, EBRJ48, and AdaBoost.MH as the best-performing methods across the spectrum of performance measures. Whenever a new method is introduced, it should be compared with different subsets of MLC methods selected according to relevant (and possibly different) evaluation criteria.
更多查看译文
关键词
Multi-label classification,Benchmarking machine learning methods,Performance estimation,Evaluation measures
AI 理解论文
溯源树
样例
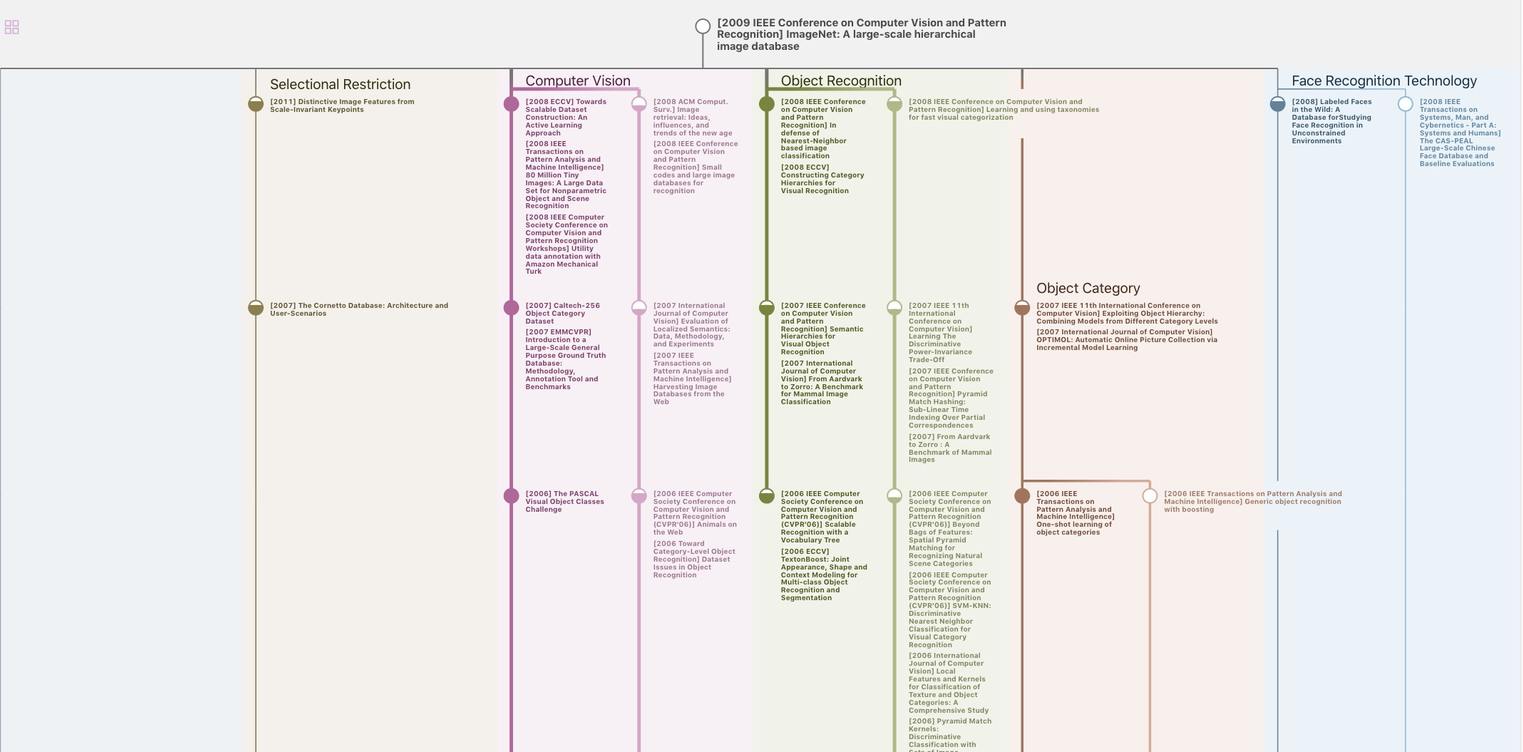
生成溯源树,研究论文发展脉络
Chat Paper
正在生成论文摘要