Approximation Bounds for Sparse Programs.
SIAM Journal on Mathematics of Data Science(2022)
摘要
We show that sparsity constrained optimization problems over low dimensional spaces tend to have a small duality gap. We use the Shapley-Folkman theorem to derive both data-driven bounds on the duality gap, and an efficient primalization procedure to recover feasible points satisfying these bounds. These error bounds are proportional to the rate of growth of the objective with the target cardinality, which means in particular that the relaxation is nearly tight as soon as the target cardinality is large enough so that only uninformative features are added.
更多查看译文
关键词
convex relaxation, sparsity, duality gap, Shapley-Folkman theorem
AI 理解论文
溯源树
样例
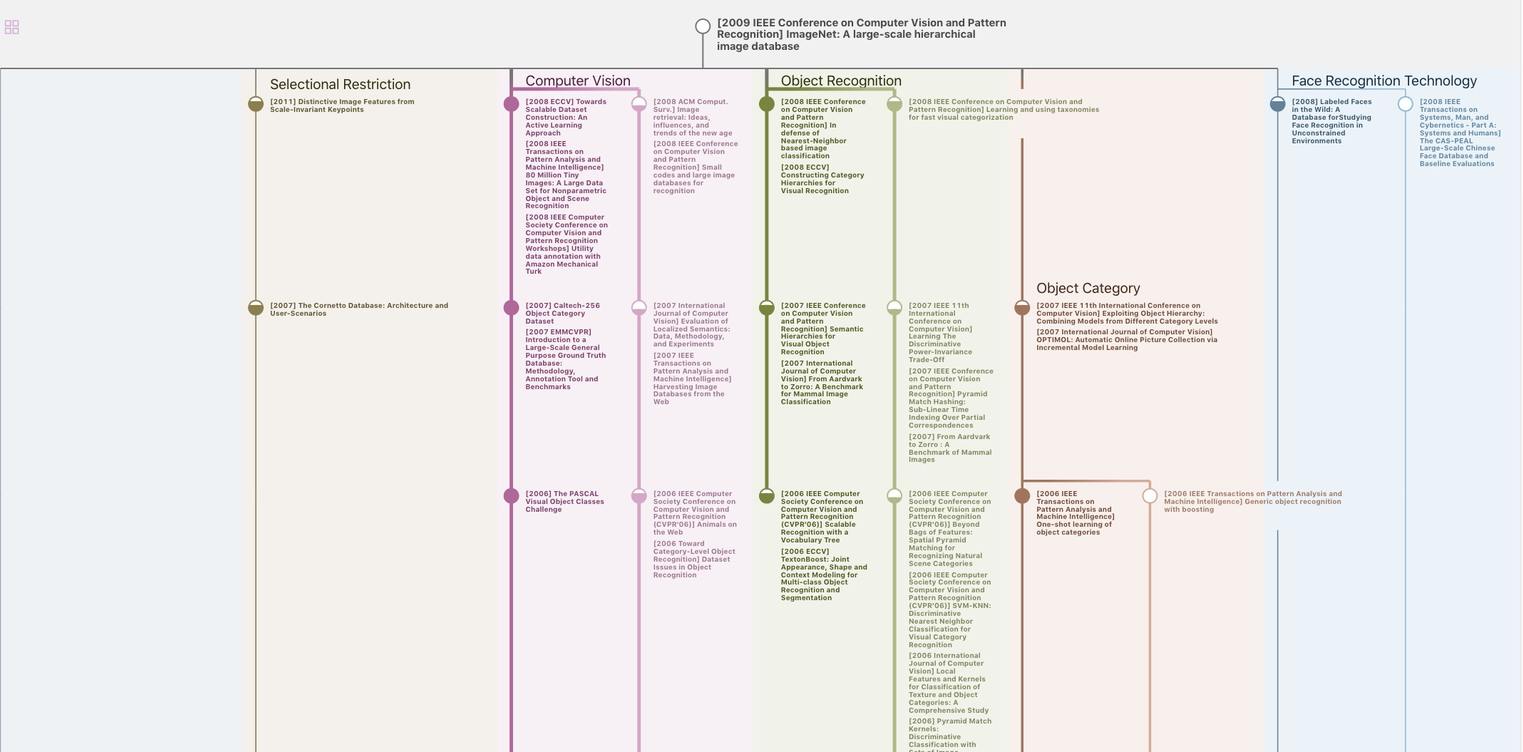
生成溯源树,研究论文发展脉络
Chat Paper
正在生成论文摘要