Stragglers Are Not Disasters: A Hybrid Federated Learning Framework with Delayed Gradients.
arxiv(2022)
摘要
Federated learning (FL) is a new machine learning framework that trains a joint model across a large number of decentralized computing devices. Existing methods, e.g., Federated Averaging (FedAvg), are able to provide an optimization guarantee by synchronously training the joint model, but usually suffer from stragglers, i.e., IoT devices with low computing power or communication bandwidth, especially on optimization problems with non-i.i.d distributed training data. To mitigate the influence of stragglers, this paper presents a novel FL framework, namely Hybrid Federated Learning (HFL), which consists of two major components: synchronous kernel and asynchronous updater that correspondingly addresses the normal devices and stragglers. Unlike traditional synchronous FL frameworks, HFL introduces the asynchronous updater which actively pulls unsynchronized and delayed local updates from stragglers to improve the efficiency and effectiveness of FL. Particularly, we propose a novel algorithm, delayed federated-SGD (DF-SGD), to merge the delayed local updates into the joint model. The results of comprehensive experiments under the HFL framework show that DF-SGD outperforms current FL methods.
更多查看译文
关键词
Federated Learning, Optimization, Large-scaled Data, Distributed Network
AI 理解论文
溯源树
样例
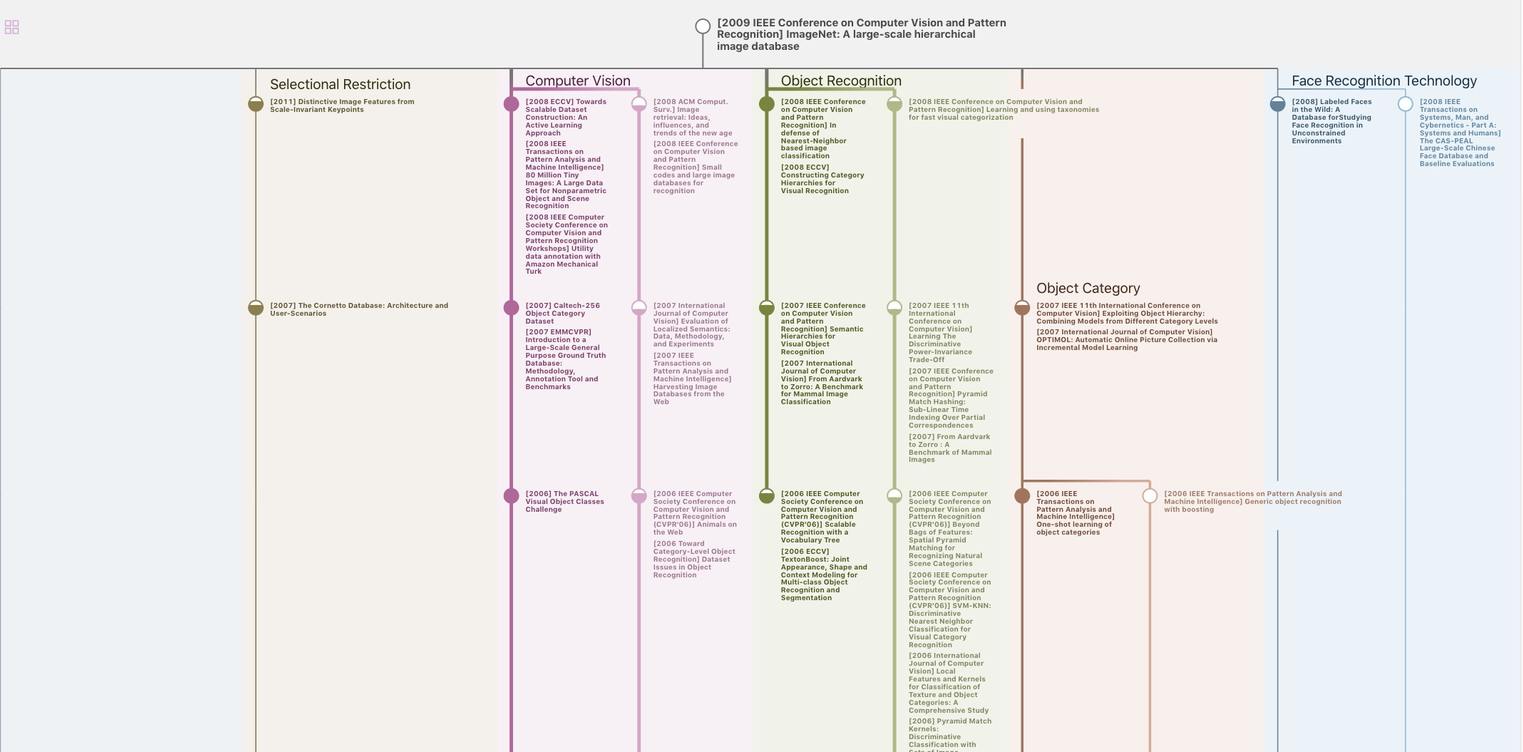
生成溯源树,研究论文发展脉络
Chat Paper
正在生成论文摘要