Exploiting low-rank covariance structures for computing high-dimensional normal and Student- t probabilities
STATISTICS AND COMPUTING(2021)
摘要
We present a preconditioned Monte Carlo method for computing high-dimensional multivariate normal and Student- t probabilities arising in spatial statistics. The approach combines a tile-low-rank representation of covariance matrices with a block-reordering scheme for efficient quasi-Monte Carlo simulation. The tile-low-rank representation decomposes the high-dimensional problem into many diagonal-block-size problems and low-rank connections. The block-reordering scheme reorders between and within the diagonal blocks to reduce the impact of integration variables from right to left, thus improving the Monte Carlo convergence rate. Simulations up to dimension 65,536 suggest that the new method can improve the run time by an order of magnitude compared with the hierarchical quasi-Monte Carlo method and two orders of magnitude compared with the dense quasi-Monte Carlo method. Our method also forms a strong substitute for the approximate conditioning methods as a more robust estimation with error guarantees. An application study to wind stochastic generators is provided to illustrate that the new computational method makes the maximum likelihood estimation feasible for high-dimensional skew-normal random fields.
更多查看译文
关键词
Block reordering, Hierarchical matrix, Skew-normal random field, Tile-low-rank matrix
AI 理解论文
溯源树
样例
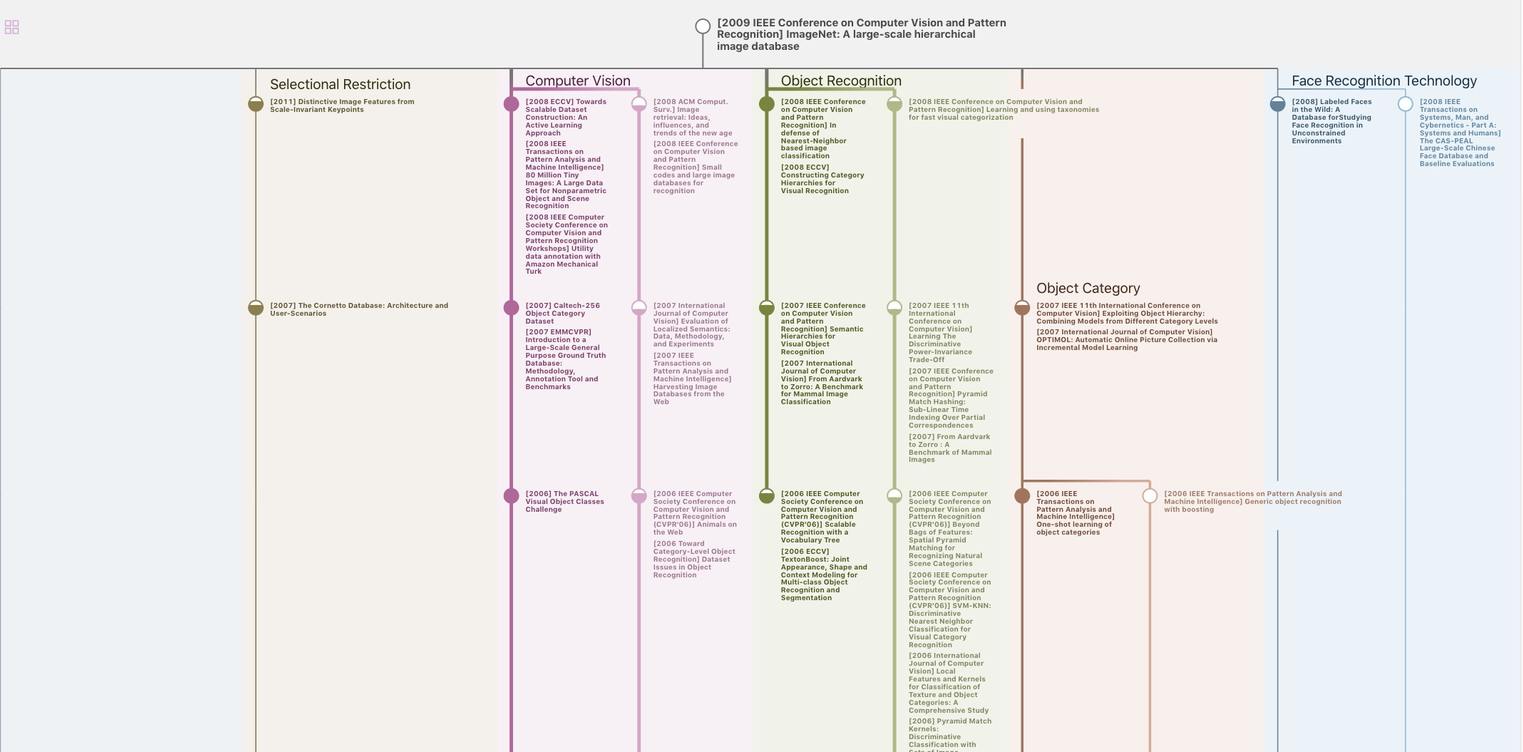
生成溯源树,研究论文发展脉络
Chat Paper
正在生成论文摘要