Fairness for Unobserved Characteristics: Insights from Technological Impacts on Queer Communities
AIES '21: PROCEEDINGS OF THE 2021 AAAI/ACM CONFERENCE ON AI, ETHICS, AND SOCIETY(2021)
摘要
Advances in algorithmic fairness have largely omitted sexual orientation and gender identity. We explore queer concerns in privacy, censorship, language, online safety, health and employment to study the positive and negative effects of artificial intelligence on queer communities. These issues underscore the need for new directions in fairness research that take into account a multiplicity of considerations, from privacy preservation, context sensitivity and process fairness, to an awareness of sociotechnical impact and the increasingly important role of inclusive and participatory research processes. Most current approaches for algorithmic fairness assume that the target characteristics for fairness-frequently, race and legal gender-can be observed or recorded. Sexual orientation and gender identity are prototypical instances of unobserved characteristics, which are frequently missing, unknown or fundamentally unmeasurable. This paper highlights the importance of developing new approaches for algorithmic fairness that break away from the prevailing assumption of observed characteristics.
更多查看译文
关键词
algorithmic fairness, queer communities, sexual orientation, gender identity, machine learning, marginalised groups
AI 理解论文
溯源树
样例
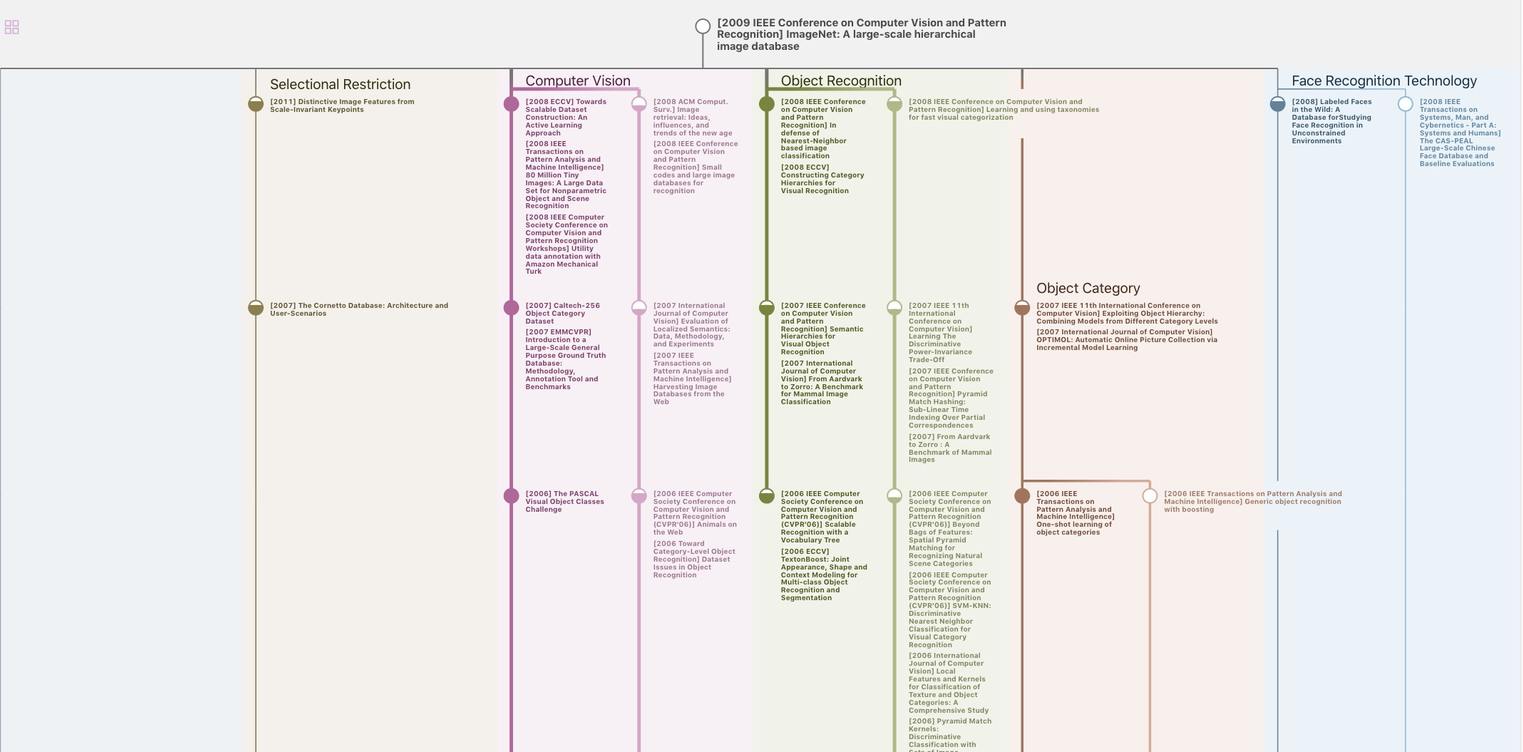
生成溯源树,研究论文发展脉络
Chat Paper
正在生成论文摘要