Near-Optimal Representation Learning For Linear Bandits And Linear Rl
INTERNATIONAL CONFERENCE ON MACHINE LEARNING, VOL 139(2021)
摘要
This paper studies representation learning for multi-task linear bandits and multi-task episodic RL with linear value function approximation. We first consider the setting where we play M linear bandits with dimension d concurrently, and these bandits share a common k-dimensional linear representation so that k << d and k << M. We propose a sample-efficient algorithm, MTLR-OFUL, which leverages the shared representation to achieve (O) over tilde (M root dkT+ d root kMT) regret, with T being the number of total steps. Our regret significantly improves upon the baseline (O) over tilde (Md root T) achieved by solving each task independently. We further develop a lower bound that shows our regret is near-optimal when d > M. Furthermore, we extend the algorithm and analysis to multi-task episodic RL with linear value function approximation under low inherent Bellman error (Zanette et al., 2020a). To the best of our knowledge, this is the first theoretical result that characterize the benefits of multi-task representation learning for exploration in RL with function approximation.
更多查看译文
AI 理解论文
溯源树
样例
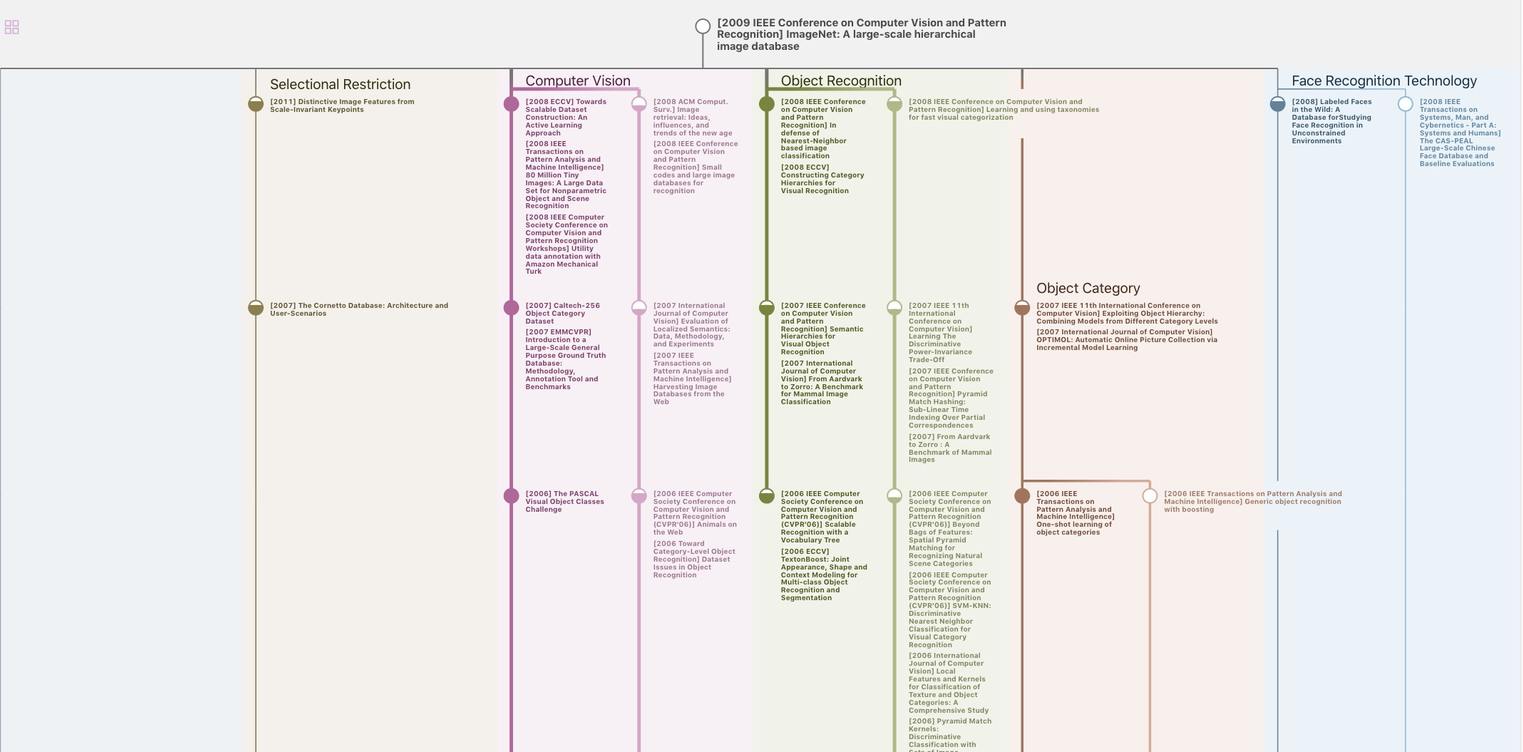
生成溯源树,研究论文发展脉络
Chat Paper
正在生成论文摘要