Temporal convolutional networks and transformers for classifying the sleep stage in awake or asleep using pulse oximetry signals
Journal of Computational Science(2022)
摘要
Sleep disorders are very widespread in the world population and suffer from a generalized underdiagnosis, given the complexity of their diagnostic methods. Therefore, there is an increasing interest in developing simpler screening methods. Pulse oximeter is an ideal device for sleep disorder screenings, since it is a portable, low-cost and accessible technology. This device can provide an estimation of the heart rate (HR), which can be useful to obtain information regarding the sleep stage. In this work, we developed a network architecture in order to classify the sleep stage in awake or asleep using only HR signals from a pulse oximeter. The proposed architecture has two fundamental parts. The first part has the aim of obtaining a representation of the HR by using temporal convolutional networks. Then, the obtained representation is used to feed the second part, which is based on transformers, a model built solely with attention mechanisms. Transformers are able to model the sequence, learning the transition rules between sleep stages. The performance of the proposed method was evaluated on the Sleep Heart Health Study dataset, composed of 5000 healthy and pathological subjects. The dataset was split into three subsets: 2500 for training, 1250 for validating and 1250 for testing. The overall accuracy, specificity, sensitivity and Cohen’s Kappa coefficient were 90.0%, 94.9%, 78.1%, and 0.73.
更多查看译文
关键词
Automatic sleep staging,Heart rate,Attention models,Transformers
AI 理解论文
溯源树
样例
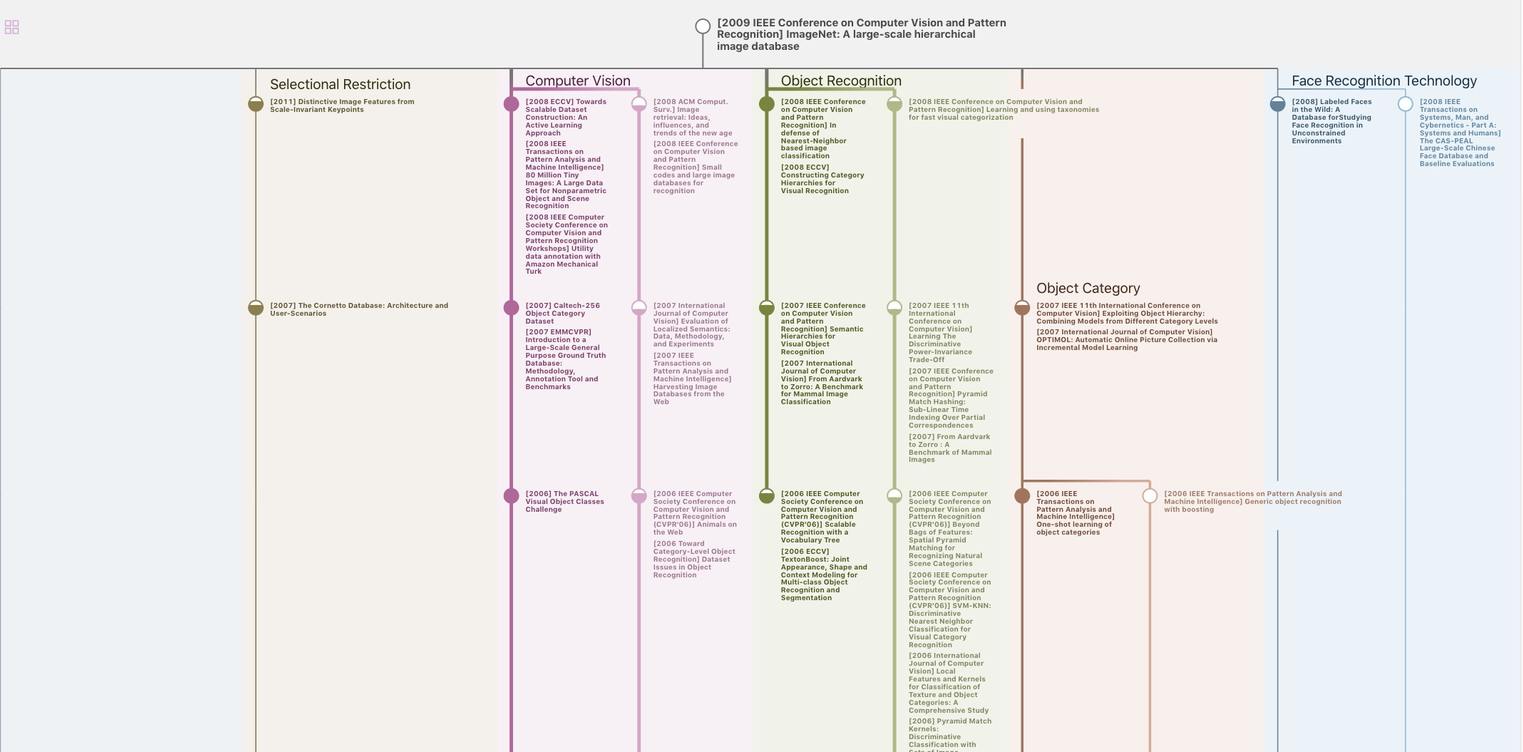
生成溯源树,研究论文发展脉络
Chat Paper
正在生成论文摘要