Theoretically Principled Deep Rl Acceleration Via Nearest Neighbor Function Approximation
THIRTY-FIFTH AAAI CONFERENCE ON ARTIFICIAL INTELLIGENCE, THIRTY-THIRD CONFERENCE ON INNOVATIVE APPLICATIONS OF ARTIFICIAL INTELLIGENCE AND THE ELEVENTH SYMPOSIUM ON EDUCATIONAL ADVANCES IN ARTIFICIAL INTELLIGENCE(2021)
摘要
Recently, deep reinforcement learning (RL) has achieved remarkable empirical success by integrating deep neural networks into RL frameworks. However, these algorithms often require a large number of training samples and admit little theoretical understanding. To mitigate these issues, we propose a theoretically principled nearest neighbor (NN) function approximator that can improve the value networks in deep RL methods. Inspired by human similarity judgments, the NN approximator estimates the action values using rollouts on past observations and can provably obtain a small regret bound that depends only on the intrinsic complexity of the environment. We present (1) Nearest Neighbor Actor-Critic (NNAC), an online policy gradient algorithm that demonstrates the practicality of combining function approximation with deep RL, and (2) a plug-and-play NN update module that aids the training of existing deep RL methods. Experiments on classical control and MuJoCo locomotion tasks show that the NN-accelerated agents achieve higher sample efficiency and stability than the baseline agents. Based on its theoretical benefits, we believe that the NN approximator can be further applied to other complex domains to speed-up learning.
更多查看译文
AI 理解论文
溯源树
样例
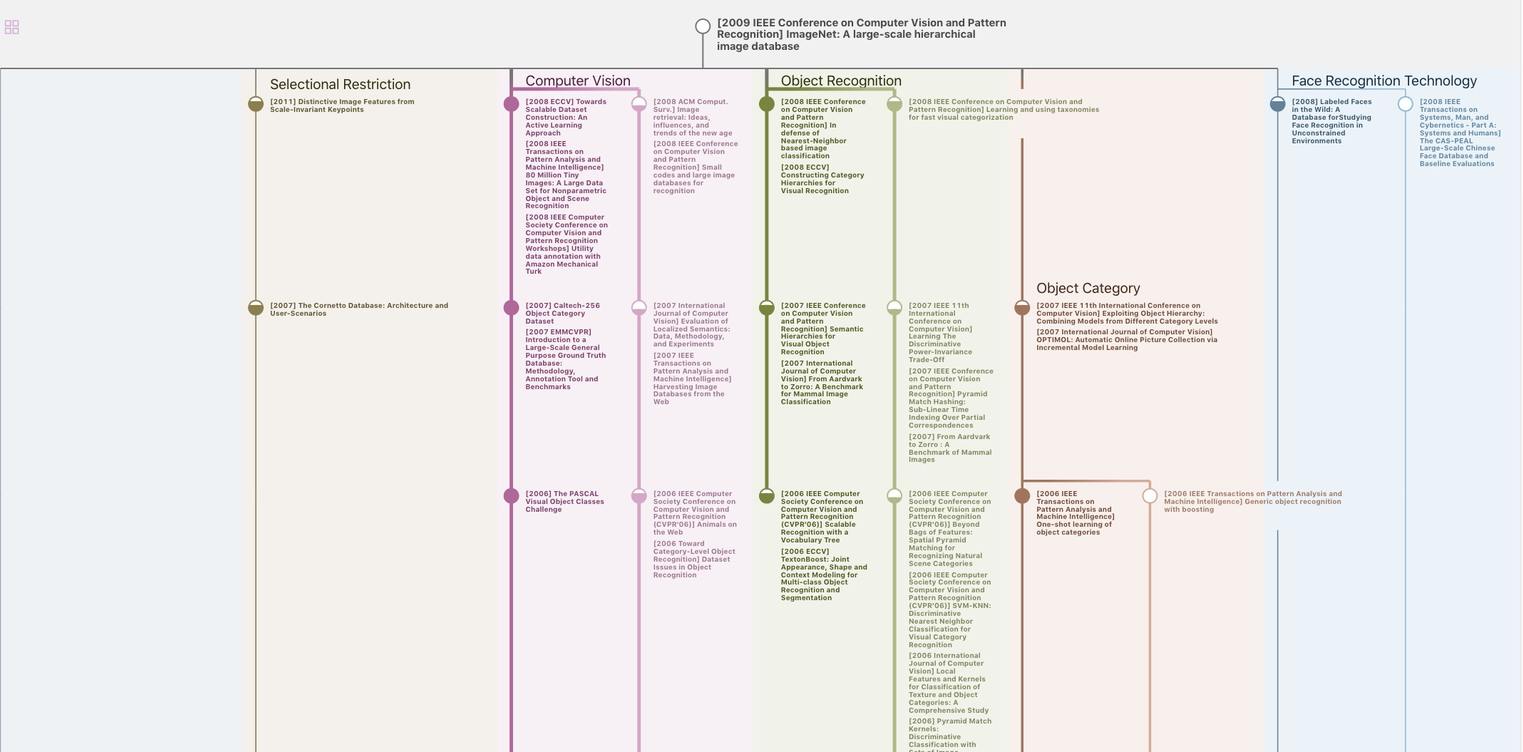
生成溯源树,研究论文发展脉络
Chat Paper
正在生成论文摘要