Optimal Resource Allocation for Crowdsourced Image Processing
2020 17th Annual IEEE International Conference on Sensing, Communication, and Networking (SECON)(2023)
摘要
Crowdsourced image processing has the potential to vastly impact response timeliness in various emergency situations. Because images can provide extremely important information regarding an event of interest (
hits
), sending the right images to an analyzer as soon as possible is of crucial importance. In this paper, we consider the problem of optimally assigning resources, both local (CPUs in phones) and remote (network-based GPUs) to mobile devices for processing images, ultimately sending those of interest to a centralized entity while also accounting for the energy consumption at the distributed nodes. To that end, we use the dual-path Network Utility Maximization (NUM) framework, coupled with a hit-ratio estimator and energy costs, to enable a distributed implementation of the system. We include analysis of different hit-ratio estimators using realistic trace data, first considering immediate and then delayed feedback. We address accuracy concerns when estimating the likelihood of future image
hits
and provide a window-based heuristic for scenarios when hit-ratio feedback is severely delayed. Our TCP-inspired window-method predicts both image
hit
likelihood and current wireless network congestion with great effectiveness. Results are validated using both synthetic simulations and real-life traces.
更多查看译文
关键词
Crowdsourcing,Optimization,Resource allocation
AI 理解论文
溯源树
样例
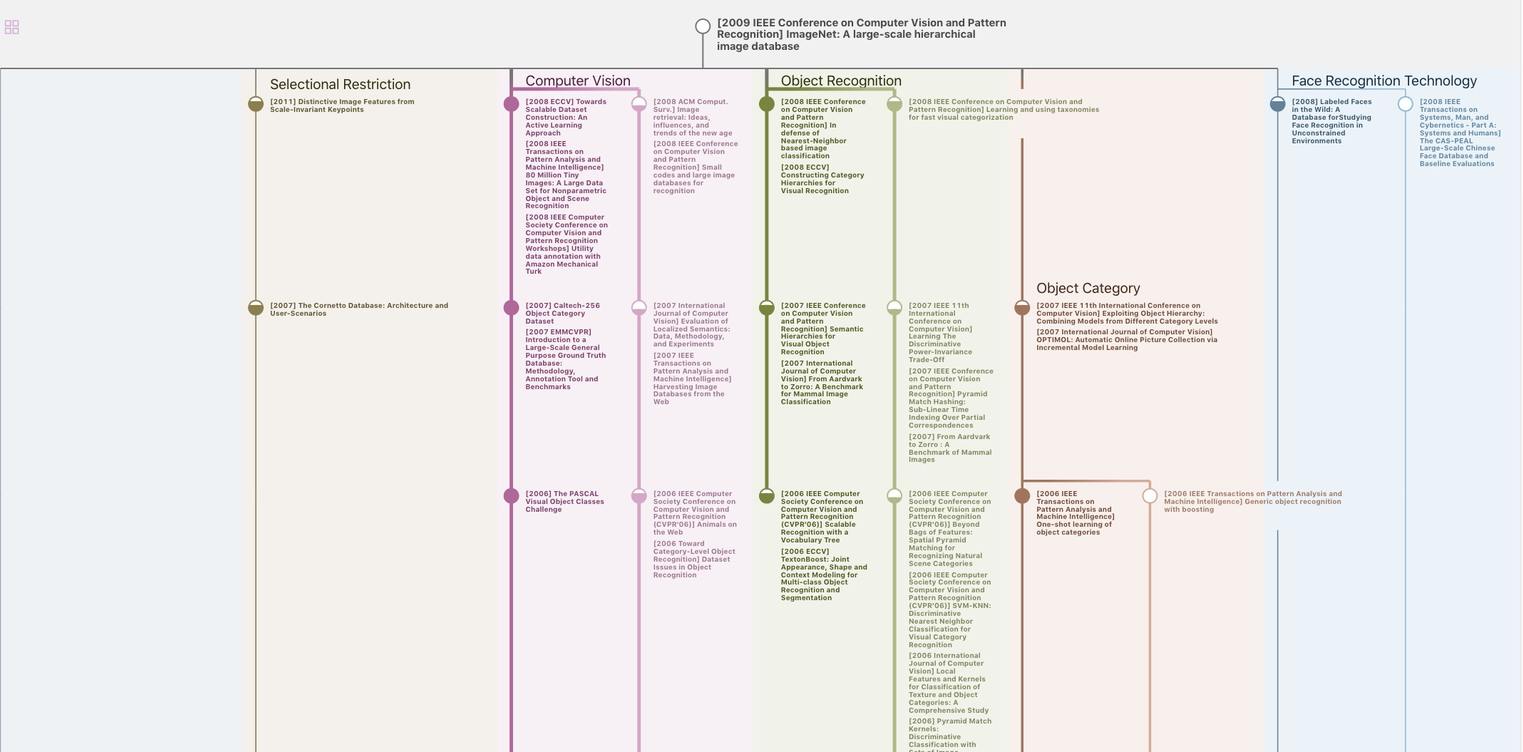
生成溯源树,研究论文发展脉络
Chat Paper
正在生成论文摘要