SEFEE: lightweight storage error forecasting in large-scale enterprise storage systems
The International Conference for High Performance Computing, Networking, Storage, and Analysis(2020)
摘要
ABSTRACTWith the rapid growth in scale and complexity, today's enterprise storage systems need to deal with significant amounts of errors. Existing proactive methods mainly focus on machine learning techniques trained using SMART measurements. However, such methods are usually expensive to use in practice and can only be applied to a limited types of errors with a limited scale. We collected more than 23-million storage events from 87 deployed NetApp-ONTAP systems managing 14,371 disks for two years and propose a lightweight training-free storage error forecasting method SEFEE. SEFEE employs Tensor Decomposition to directly analyze storage error-event logs and perform online error prediction for all error types in all storage nodes. SEFEE explores hidden spatio-temporal information that is deeply embedded in the global scale of storage systems to achieve record breaking error forecasting accuracy with minimal prediction overhead.
更多查看译文
关键词
Storage failures,error prediction,lightweight forecasting,training-free prediction,tensor decomposition
AI 理解论文
溯源树
样例
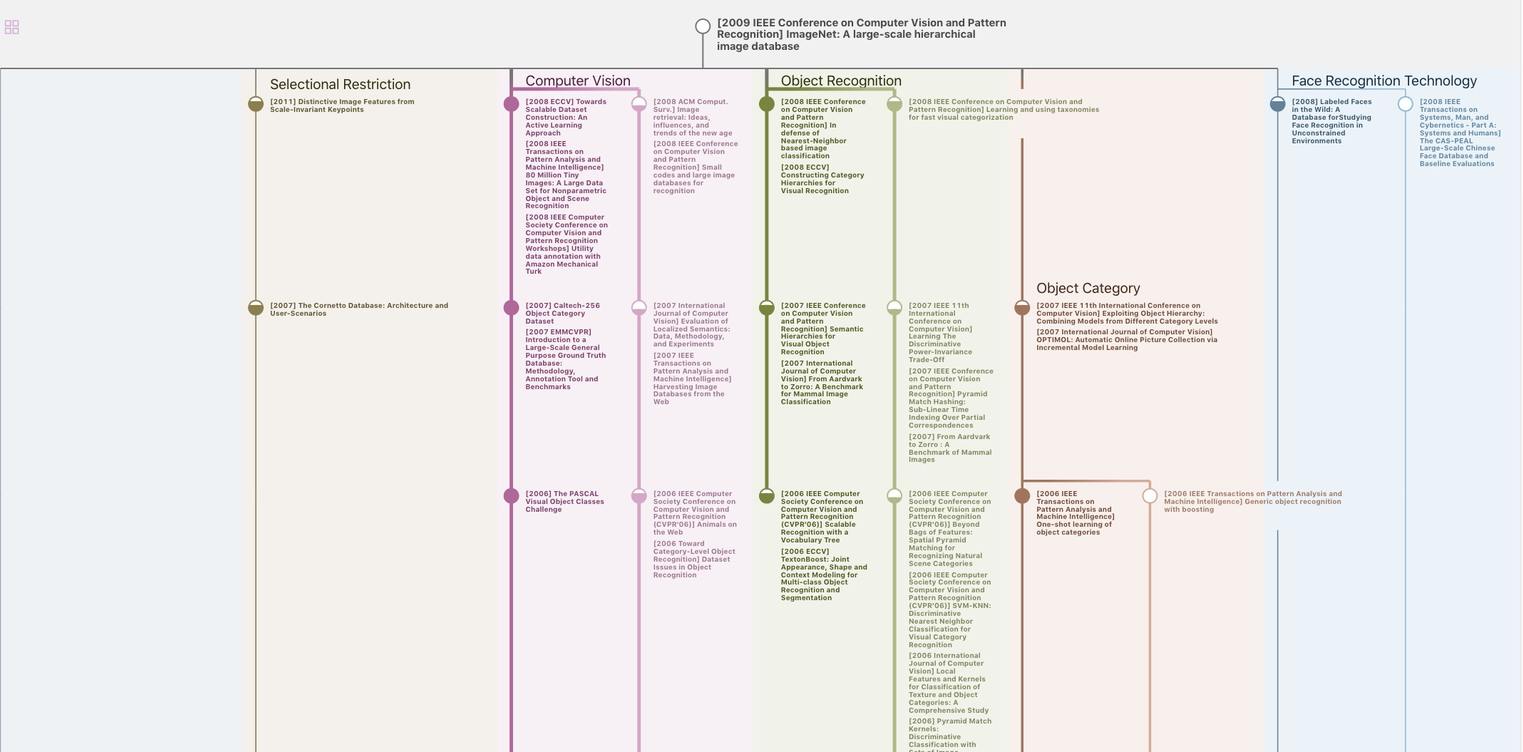
生成溯源树,研究论文发展脉络
Chat Paper
正在生成论文摘要