Robust Pedestrian Detection In Thermal Imagery Using Synthesized Images
2020 25TH INTERNATIONAL CONFERENCE ON PATTERN RECOGNITION (ICPR)(2020)
摘要
In this paper we propose a method for improving pedestrian detection in the thermal domain using two stages: first, a generative data augmentation approach is used, then a domain adaptation method using generated data adapts an RGB pedestrian detector. Our model, based on the Least-Squares Generative Adversarial Network, is trained to synthesize realistic thermal versions of input RGB images which are then used to augment the limited amount of labeled thermal pedestrian images available for training. We apply our generative data augmentation strategy in order to adapt a pretrained YOLOv3 pedestrian detector to detection in the thermal-only domain. Experimental results demonstrate the effectiveness of our approach: using less than 50% of available real thermal training data, and relying on synthesized data generated by our model in the domain adaptation phase, our detector achieves state-of-the-art results on the KAIST Multispectral Pedestrian Detection Benchmark; even if more real thermal data is available adding GAN generated images to the training data results in improved performance, thus showing that these images act as an effective form of data augmentation. To the best of our knowledge, our detector achieves the best single-modality detection results on KAIST with respect to the state-of-the-art.
更多查看译文
关键词
robust pedestrian detection,thermal imagery
AI 理解论文
溯源树
样例
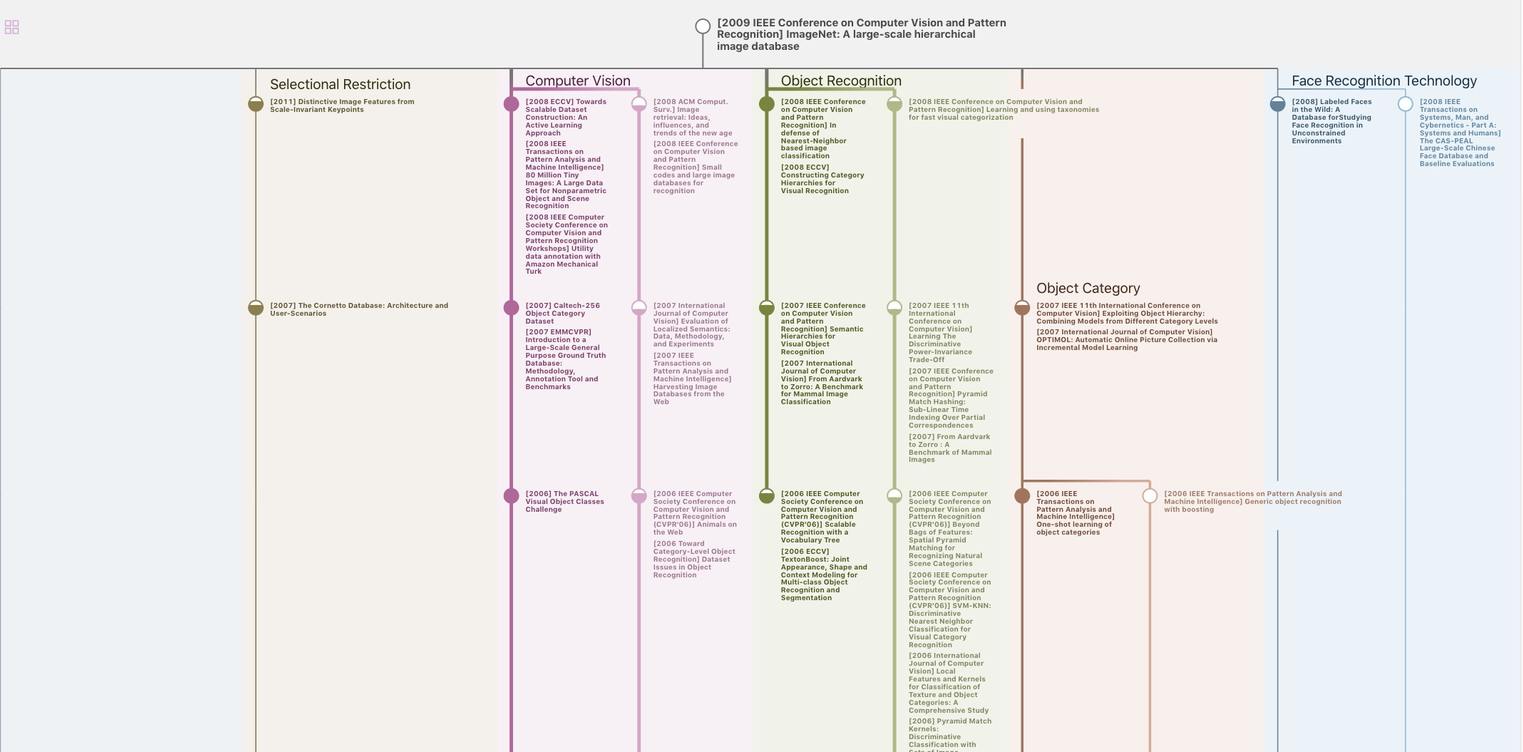
生成溯源树,研究论文发展脉络
Chat Paper
正在生成论文摘要