A Causal Convolutional Neural Network for Motion Modeling and Synthesis
arxiv(2021)
摘要
We propose a novel deep generative model based on causal convolutions for multi-subject motion modeling and synthesis, which is inspired by the success of WaveNet in multi-subject speech synthesis. However, it is nontrivial to adapt WaveNet to handle high-dimensional and physically constrained motion data. To this end, we add an encoder and a decoder to the WaveNet to translate the motion data into features and back to the predicted motions. We also add 1D convolution layers to take skeleton configuration as an input to model skeleton variations across different subjects. As a result, our network can scale up well to large-scale motion data sets across multiple subjects and support various applications, such as random and controllable motion synthesis, motion denoising, and motion completion, in a unified way. Complex motions, such as punching, kicking and, kicking while punching, are also well handled. Moreover, our network can synthesize motions for novel skeletons not in the training dataset. After fine-tuning the network with a few motion data of the novel skeleton, it is able to capture the personalized style implied in the motion and generate high-quality motions for the skeleton. Thus, it has the potential to be used as a pre-trained network in few-shot learning for motion modeling and synthesis. Experimental results show that our model can effectively handle the variation of skeleton configurations, and it runs fast to synthesize different types of motions on-line. We also perform user studies to verify that the quality of motions generated by our network is superior to the motions of state-of-the-art human motion synthesis methods.
更多查看译文
关键词
causal convolutional neural network,motion modeling,convolutional neural network
AI 理解论文
溯源树
样例
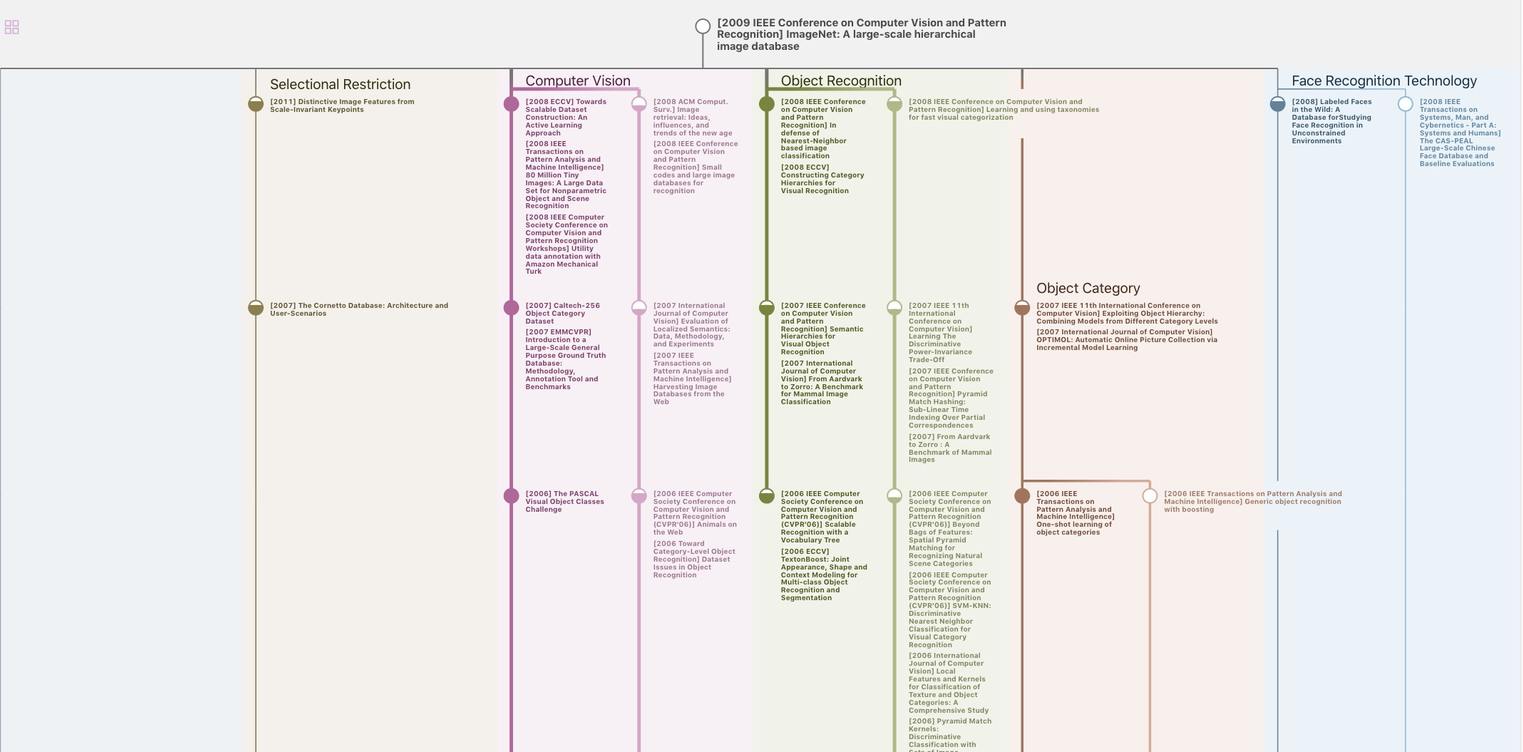
生成溯源树,研究论文发展脉络
Chat Paper
正在生成论文摘要