B-Spline Parameterized Joint Optimization of Reconstruction and K-Space Trajectories (BJORK) for Accelerated 2D MRI
IEEE Transactions on Medical Imaging(2022)
摘要
Optimizing k-space sampling trajectories is a promising yet challenging topic for fast magnetic resonance imaging (MRI). This work proposes to optimize a reconstruction method and sampling trajectories jointly concerning image reconstruction quality in a supervised learning manner. We parameterize trajectories with quadratic B-spline kernels to reduce the number of parameters and apply multi-scale optimization, which may help to avoid sub-optimal local minima. The algorithm includes an efficient non-Cartesian unrolled neural network-based reconstruction and an accurate approximation for backpropagation through the non-uniform fast Fourier transform (NUFFT) operator to accurately reconstruct and back-propagate multi-coil non-Cartesian data. Penalties on slew rate and gradient amplitude enforce hardware constraints. Sampling and reconstruction are trained jointly using large public datasets. To correct for possible eddy-current effects introduced by the curved trajectory, we use a pencil-beam trajectory mapping technique. In both simulations and in- vivo experiments, the learned trajectory demonstrates significantly improved image quality compared to previous model-based and learning-based trajectory optimization methods for
$10\times $
acceleration factors. Though trained with neural network-based reconstruction, the proposed trajectory also leads to improved image quality with compressed sensing-based reconstruction.
更多查看译文
关键词
Algorithms,Brain,Fourier Analysis,Image Processing, Computer-Assisted,Magnetic Resonance Imaging
AI 理解论文
溯源树
样例
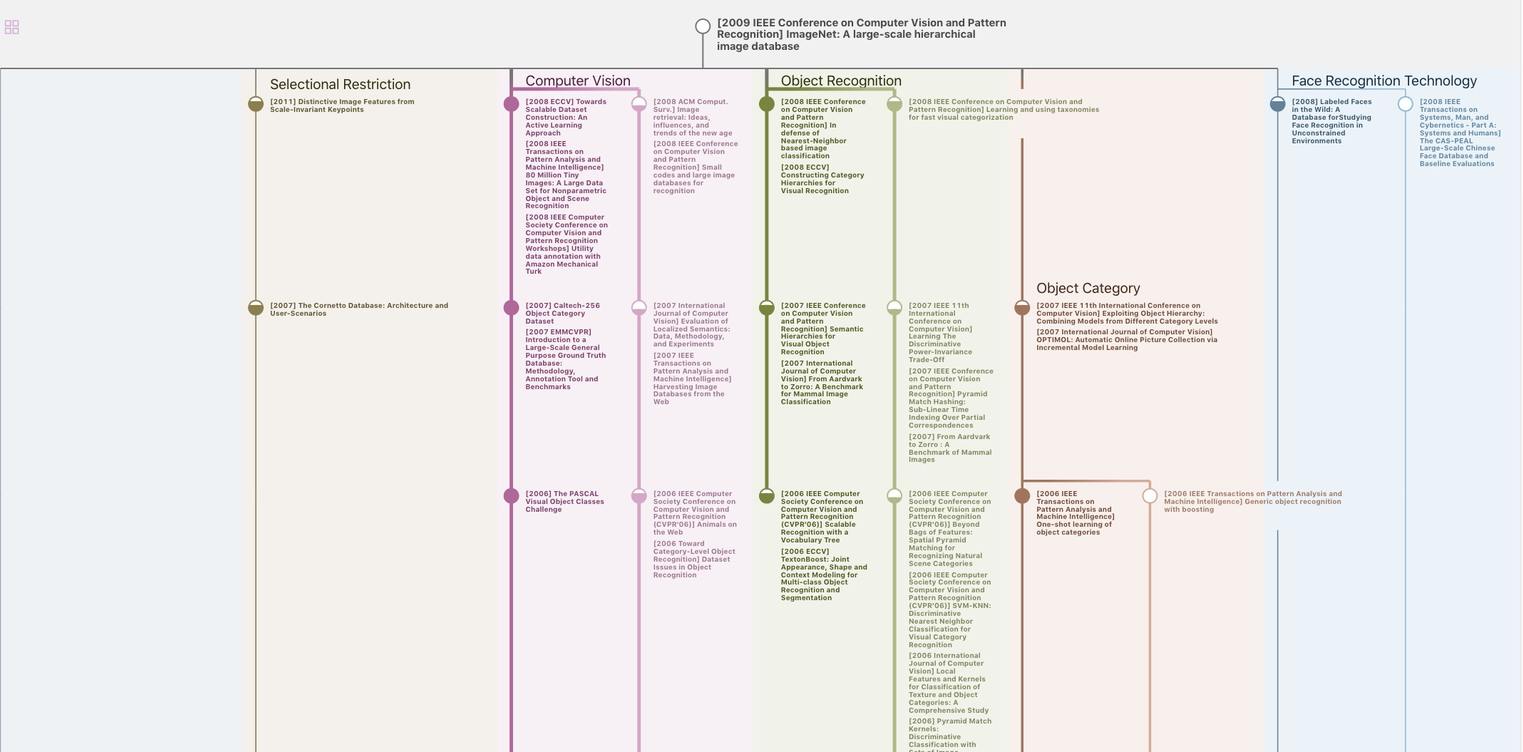
生成溯源树,研究论文发展脉络
Chat Paper
正在生成论文摘要