MoNet: Impressionism As A Defense Against Adversarial Examples
2020 Second IEEE International Conference on Trust, Privacy and Security in Intelligent Systems and Applications (TPS-ISA)(2020)
摘要
While image classifiers based on Convolutional Neural Networks (CNN) are extremely successful, they often suffer from adversarial examples. To address this problem, we explore the combination of using secret randomness and Floyd-Steinberg dithering. More specifically, each CNN is trained with a secret, random key. Input images are first processed using Floyd-Steinberg dithering to reduce the color depth, and then each pixel is encrypted using the AES block cipher. Processed images are then fed into a standard CNN. We call our approach MoNet, because the processed images are reminiscent of impressionistic paintings. Classifiers trained with MoNet showed significant improvements in model stability and robustness against adversarial inputs. MoNet significantly improves the resilience against transferability of adversarial examples, at the cost of a small drop in prediction accuracy.
更多查看译文
关键词
Adversarial Examples,Neural Network,MoNet,Floyd-Steinberg Dithering
AI 理解论文
溯源树
样例
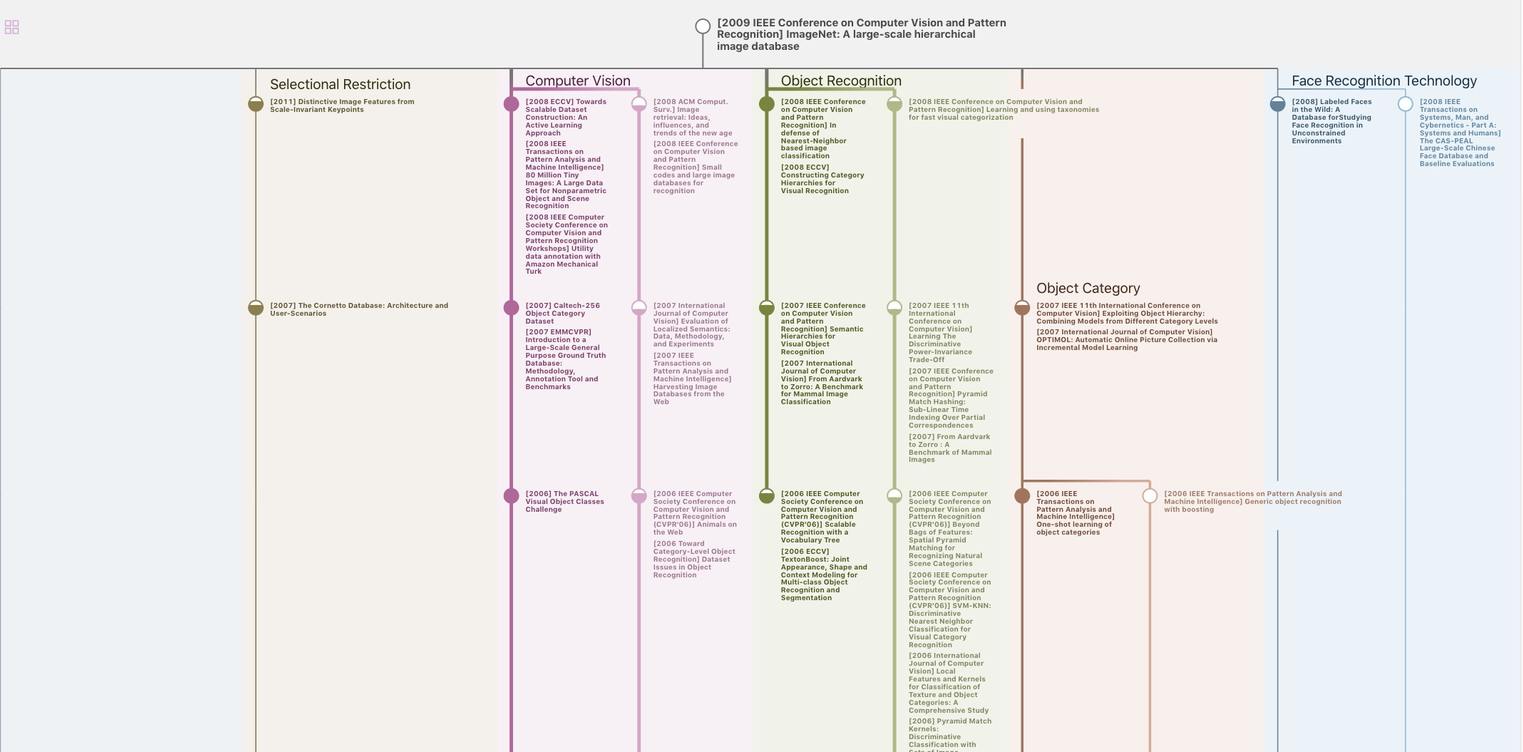
生成溯源树,研究论文发展脉络
Chat Paper
正在生成论文摘要