Exploring Multi-Task Multi-Lingual Learning of Transformer Models for Hate Speech and Offensive Speech Identification in Social Media
SN Comput. Sci.(2021)
摘要
Hate Speech has become a major content moderation issue for online social media platforms. Given the volume and velocity of online content production, it is impossible to manually moderate hate speech related content on any platform. In this paper we utilize a multi-task and multi-lingual approach based on recently proposed Transformer Neural Networks to solve three sub-tasks for hate speech. These sub-tasks were part of the 2019 shared task on hate speech and offensive content (HASOC) identification in Indo-European languages. We expand on our submission to that competition by utilizing multi-task models which are trained using three approaches, (a) multi-task learning with separate task heads, (b) back-translation, and (c) multi-lingual training. Finally, we investigate the performance of various models and identify instances where the Transformer based models perform differently and better. We show that it is possible to to utilize different combined approaches to obtain models that can generalize easily on different languages and tasks, while trading off slight accuracy (in some cases) for a much reduced inference time compute cost. We open source an updated version of our HASOC 2019 code with the new improvements at https://github.com/socialmediaie/MTML_HateSpeech .
更多查看译文
关键词
Hate speech,Offensive content,Transformer models,BERT,Language models,Neural networks,Multi-lingual,Multi-Task learning,Social media,Natural language processing,Machine learning,Deep learning,Open source
AI 理解论文
溯源树
样例
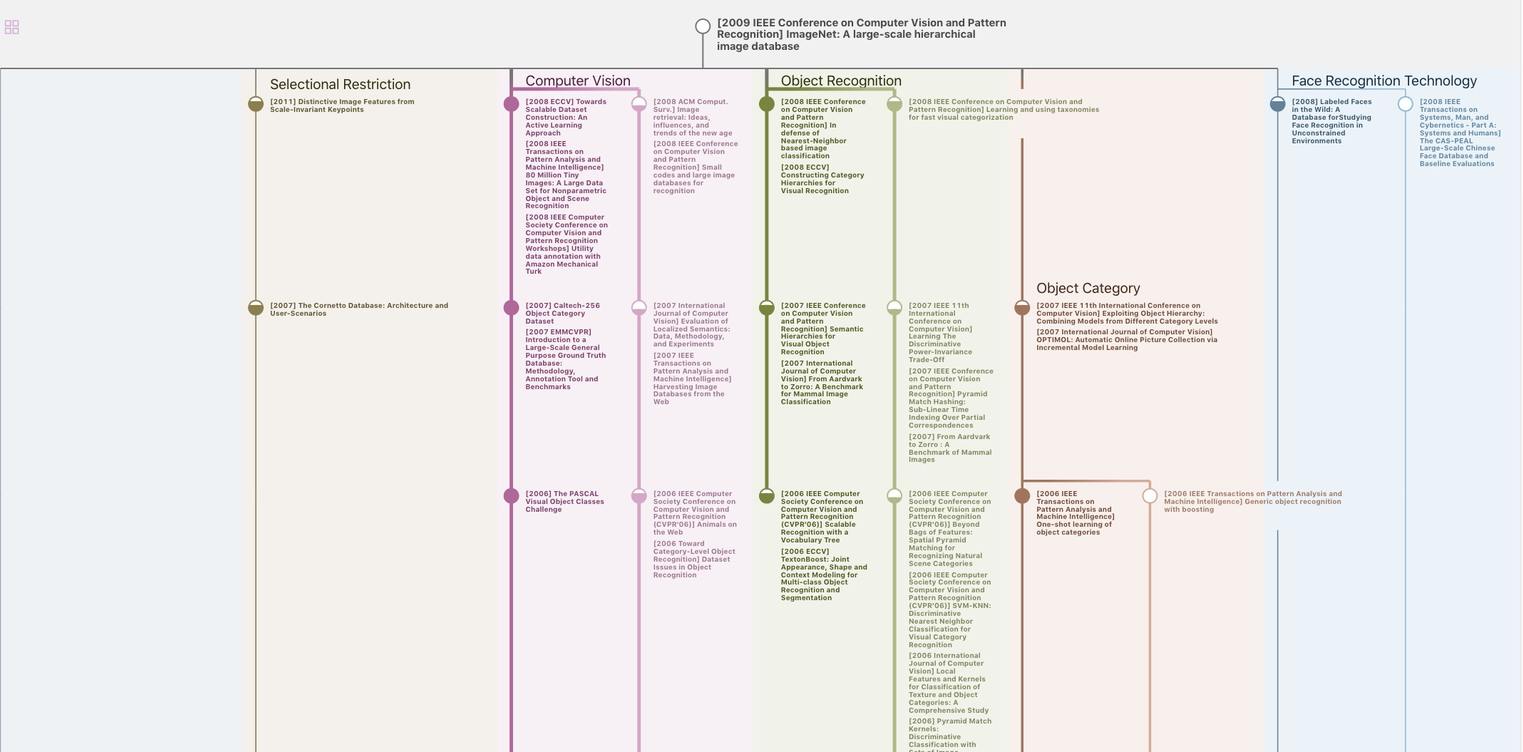
生成溯源树,研究论文发展脉络
Chat Paper
正在生成论文摘要