Automatic Curation of Large-Scale Datasets for Audio-Visual Representation Learning
arxiv(2021)
摘要
Large-scale datasets are the cornerstone of self-supervised representation learning. Existing algorithms extract learning signals by making certain assumptions about the data, e.g., spatio-temporal continuity and multimodal correspondence. Unfortunately, finding a large amount of data that satisfies such assumptions is sometimes not straightforward. This restricts the community to rely on datasets that require laborious annotation and/or manual filtering processes. In this paper, we describe a subset optimization approach for automatic dataset curation. Focusing on the scenario of audio-visual representation learning, we pose the problem as finding a subset that maximizes the mutual information between audio and visual channels in videos. We demonstrate that our approach finds videos with high audio-visual correspondence and show that self-supervised models trained on our data, despite being automatically constructed, achieve similar downstream performances to existing video datasets with similar scales. The most significant benefit of our approach is scalability. We release the largest video dataset for audio-visual research collected automatically using our approach.
更多查看译文
关键词
Datasets and evaluation,Representation learning,Video analysis and understanding
AI 理解论文
溯源树
样例
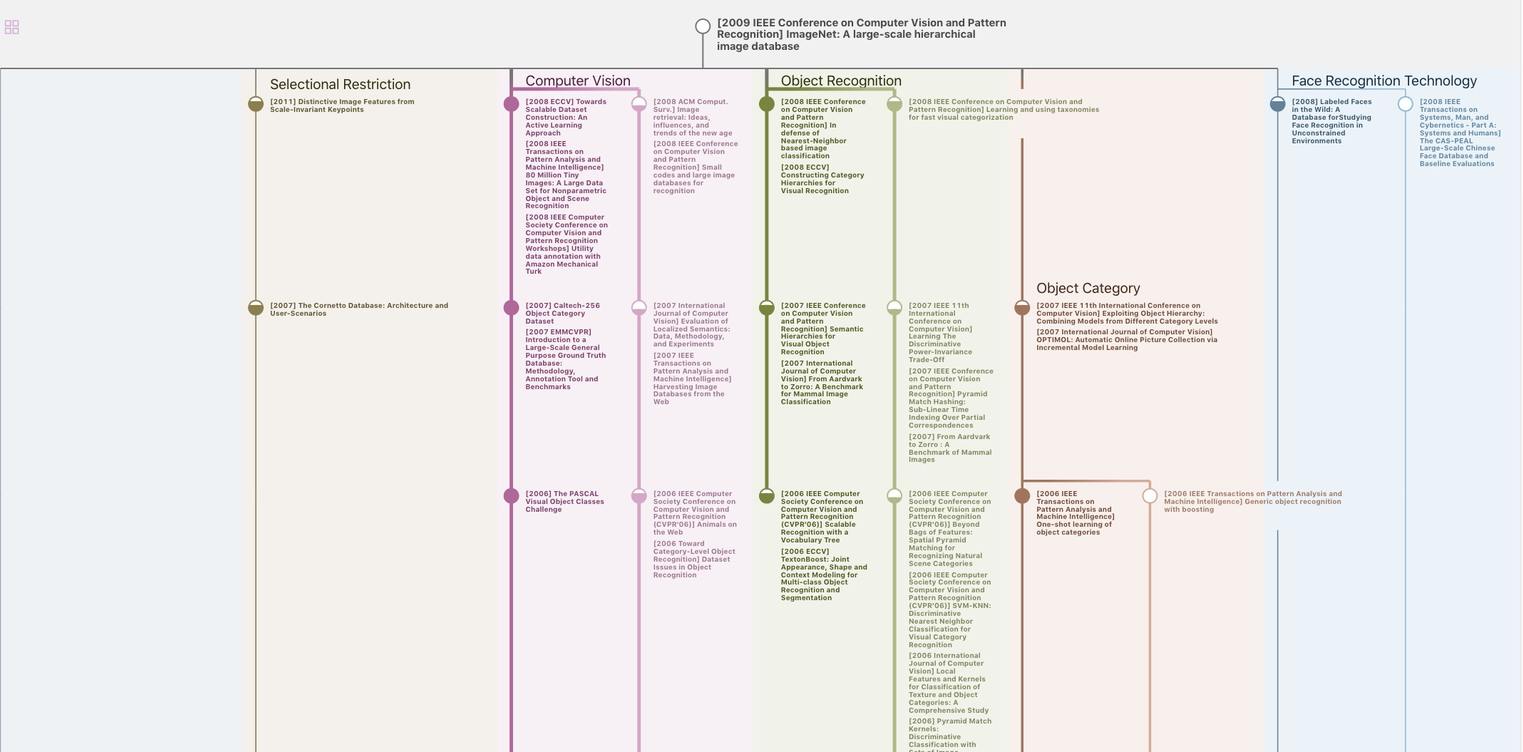
生成溯源树,研究论文发展脉络
Chat Paper
正在生成论文摘要