Bayesian Edge Regression in Undirected Graphical Models to Characterize Interpatient Heterogeneity in Cancer
JOURNAL OF THE AMERICAN STATISTICAL ASSOCIATION(2022)
摘要
It is well established that interpatient heterogeneity in cancer may significantly affect genomic data analyses and in particular, network topologies. Most existing graphical model methods estimate a single population-level graph for genomic or proteomic network. In many investigations, these networks depend on patient-specific indicators that characterize the heterogeneity of individual networks across subjects with respect to subject-level covariates. Examples include assessments of how the network varies with patient-specific prognostic scores or comparisons of tumor and normal graphs while accounting for tumor purity as a continuous predictor. In this article, we propose a novel edge regression model for undirected graphs, which estimates conditional dependencies as a function of subject-level covariates. We evaluate our model performance through simulation studies focused on comparing tumor and normal graphs while adjusting for tumor purity. In application to a dataset of proteomic measurements on plasma samples from patients with hepatocellular carcinoma (HCC), we ascertain how blood protein networks vary with disease severity, as measured by HepatoScore, a novel biomarker signature measuring disease severity. Our case study shows that the network connectivity increases with HepatoScore and a set of hub proteins as well as important protein connections are identified under different HepatoScore, which may provide important biological insights to the development of precision therapies for HCC.
更多查看译文
关键词
Bayesian adaptive shrinkage,Gene regulatory network,Nonstatic graph,Tumor heterogeneity,Undirected graphical models
AI 理解论文
溯源树
样例
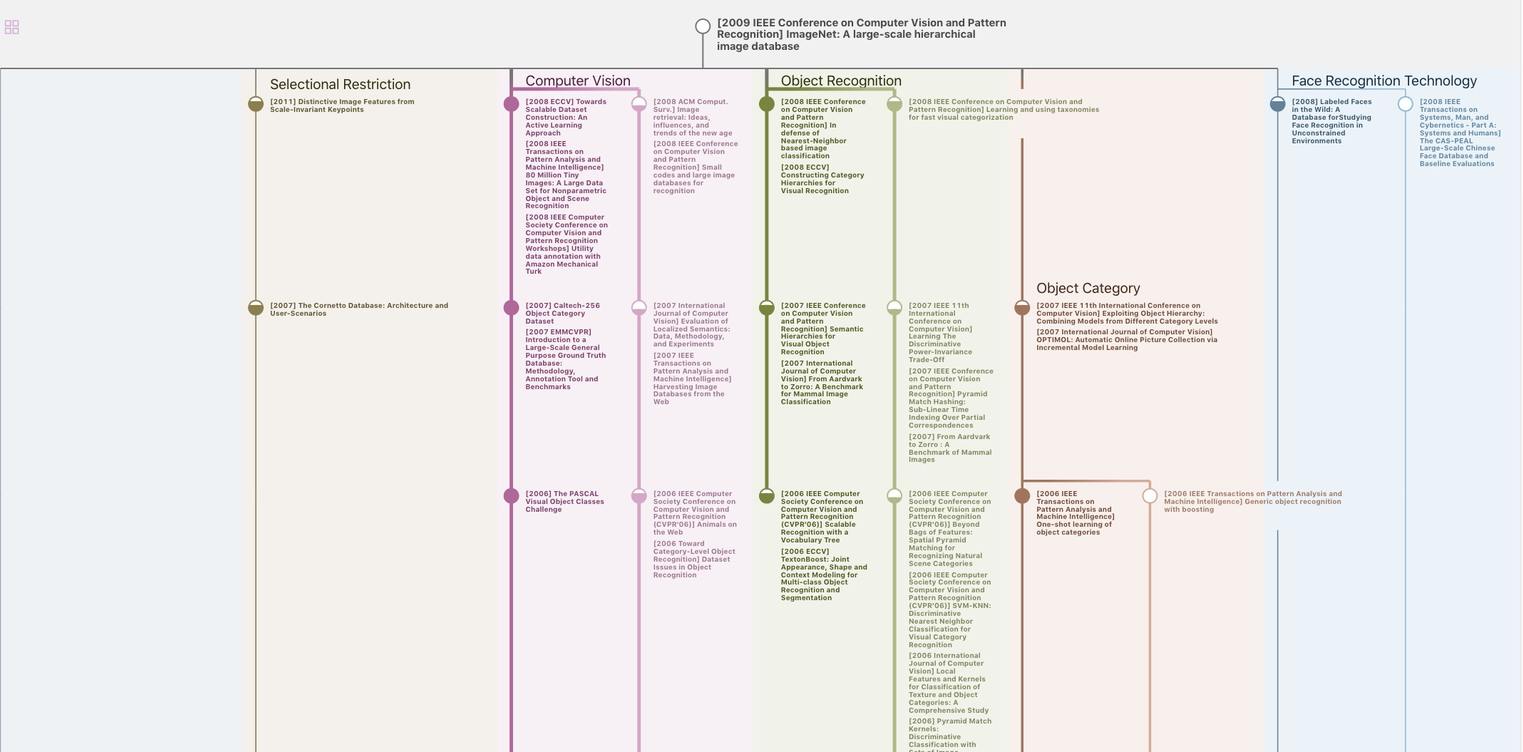
生成溯源树,研究论文发展脉络
Chat Paper
正在生成论文摘要