A cross-correction LiDAR SLAM method for high-accuracy 2D mapping of problematic scenario
ISPRS Journal of Photogrammetry and Remote Sensing(2021)
摘要
Highly accurate 2D maps can supply basic geospatial information for efficient and accurate indoor building modeling. However, problematic scenarios, which are characterized by few features, similar components and large scales, seriously influence data association and cumulative error elimination, and thus degrade simultaneous localization and mapping (SLAM)-based mapping quality. In this paper, a cross-correction LiDAR SLAM method is proposed for constructing high-accuracy 2D maps of problematic scenarios. The method comprises two models. The first model, namely, pose correction for rough mapping (PCRM), increases the data association capacity and generates a rough map with cumulative errors. In the PCRM model, a rough mapping module is developed against the scenario with few features for accurate data association. This module improves the robustness of the data association by using the initial poses from the local pose correction module, especially in similar-component scenarios. The other is a map correction for pose optimization (MCPO) model, which enhances cumulative error elimination capacity. Here, a block-based local map correction module is proposed that takes both map and pose into consideration to construct accurate constraints. The constraints are then added to the global pose optimization module to significantly reduce the cumulative error of the rough map and thus construct a high-accuracy 2D map. The results demonstrate the superiority of our method over 5 other state-of-the-art methods in problematic scenarios. The overall performance of our method in these two scenarios is approximately 1 cm and 0.2% in terms of the absolute and relative map errors, respectively. Moreover, the modeling results demonstrate that our method can be applied to the efficient and accurate indoor modeling.
更多查看译文
关键词
High-accuracy 2D map,Indoor modeling,Cross-correction SLAM,Mobile LiDAR,Problematic scenario
AI 理解论文
溯源树
样例
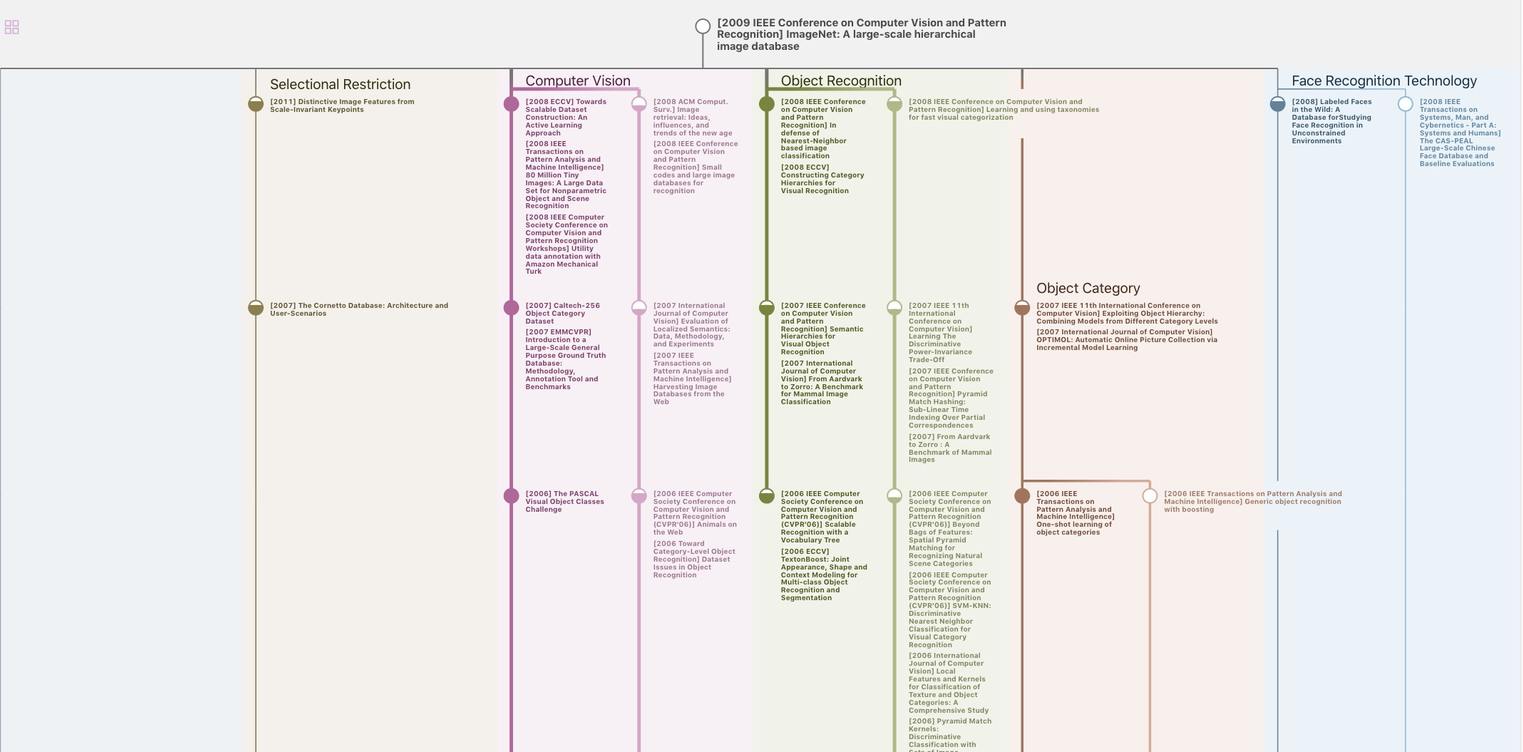
生成溯源树,研究论文发展脉络
Chat Paper
正在生成论文摘要