Attention-Based Sequence Learning Model For Travel Time Estimation
IEEE ACCESS(2020)
摘要
Travel time estimation (TTE) on a specific route is a challenging task since the complex road network structure and hard-captured temporal patterns. Many excellent methods have been proposed to address the aforementioned problems. Some approaches well designed heuristically in a non-learning based way have the advantage of a quick response to the query for travel time estimation. However, these methods are largely affected by the noise of traffic data since they are limited to a single feature. Existing road segment based methods are generally considered intuitive but are not accurate enough for they fail to model complex factors, like delay and direction of intersections. In this paper, we propose a novel attention based sequence learning model for travel time estimation of a path (ASTTE), that not only considers the real-world road network topology as multi-relational data but also refine the problem to the road segment and intersection direction aspects. Besides, we integrate the traffic information as local and neighbor dependency, which helps to monitor dynamic traffic conditions during the trip. The use of the attention mechanism allows the model to focus on significant elements among the path comprises road segments and intersections. High-quality experiments on two real-world datasets have demonstrated the effectiveness and robustness of our framework.
更多查看译文
关键词
Roads, Estimation, Data models, Trajectory, Task analysis, Predictive models, Global Positioning System, Travel time estimation, road network topology, multi-relational data
AI 理解论文
溯源树
样例
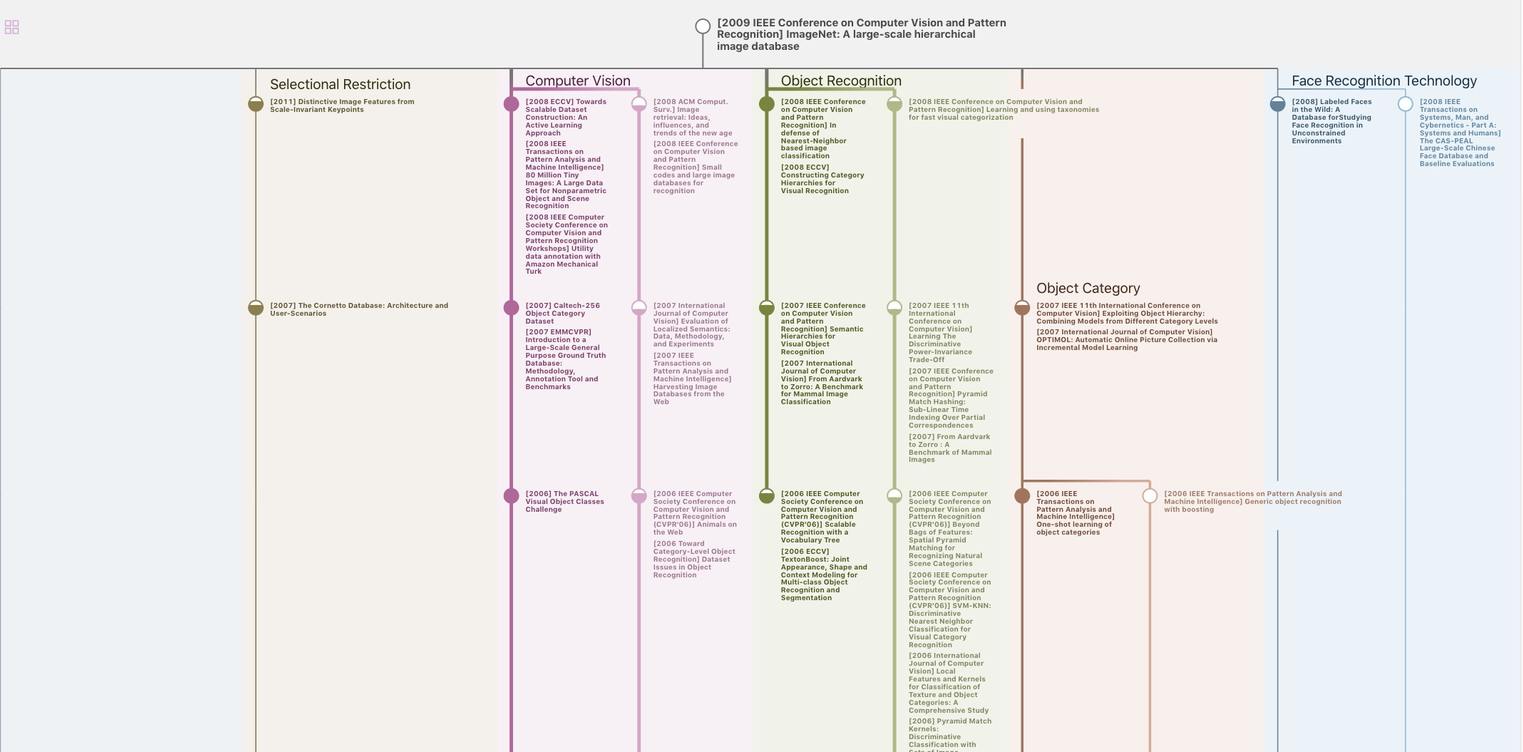
生成溯源树,研究论文发展脉络
Chat Paper
正在生成论文摘要