Interactive Anomaly Detection Based on Clustering and Online Mirror Descent
IoT Streams/ITEM@PKDD/ECML(2020)
摘要
In several applications, when anomalies are detected, human experts have to investigate or verify them one by one. As they investigate, they unwittingly produce a label - true positive (TP) or false positive (FP). In this paper, we propose a method (called OMD-Clustering) that exploits this label feedback to minimize the FP rate and detect more relevant anomalies, while minimizing the expert effort required to investigate them. The OMD-Clustering method iteratively suggests the top-1 anomalous instance to a human expert and receives feedback. Before suggesting the next anomaly, the method re-ranks instances so that the top anomalous instances are similar to the TP instances and dissimilar to the FP instances. This is achieved by learning to score anomalies differently in various regions of the feature space. An experimental evaluation on several real-world datasets is conducted. The results show that OMD-Clustering achieves significant improvement in both detection precision and expert effort compared to state-of-the-art interactive anomaly detection methods.
更多查看译文
关键词
anomaly detection,clustering
AI 理解论文
溯源树
样例
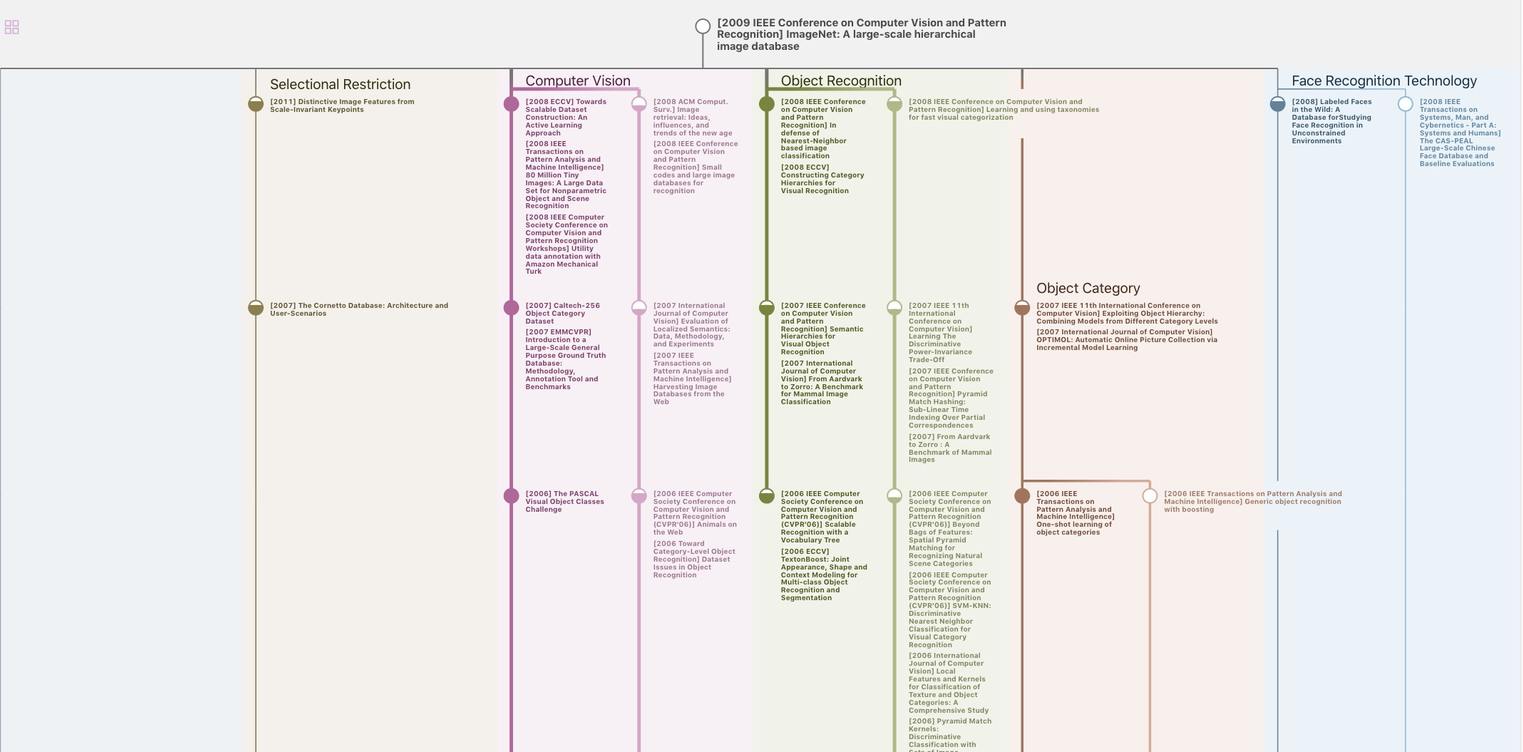
生成溯源树,研究论文发展脉络
Chat Paper
正在生成论文摘要