Per-channel Quantization Level Allocation for Quantizing Convolutional Neural Networks
2020 IEEE International Conference on Consumer Electronics - Asia (ICCE-Asia)(2020)
摘要
Deep convolutional neural network such as ResNet18/50 for ImageNet classification can be quantized into 3-bit or higher precision with the accuracy of full precision (FP32) baseline, yet 2-bit quantization incurs significant accuracy losses. In this work, we report, given that ranges of per-channel activation distributions vary across activation channels, 2-bit (4-level) quantization loses most of the information in the activation channel with a small range. To minimize the loss of information of the activation channel with a small range, we propose a novel quantization method called Per-channel Quantization Level Allocation (PCQLA), which quantizes activation into 2-bit precision with per-channel quantization clipping value according to the range of per-channel activation distribution. We also apply PCQLA mothed with outlier-aware quantization. On the ImageNet classification dataset, our method offers sufficient accuracies, comparable to the full precision baseline, with 2-bit activation quantization on ResNet-18/50.
更多查看译文
关键词
Convolutional Neural Network,Neural Network Compression,Quantization
AI 理解论文
溯源树
样例
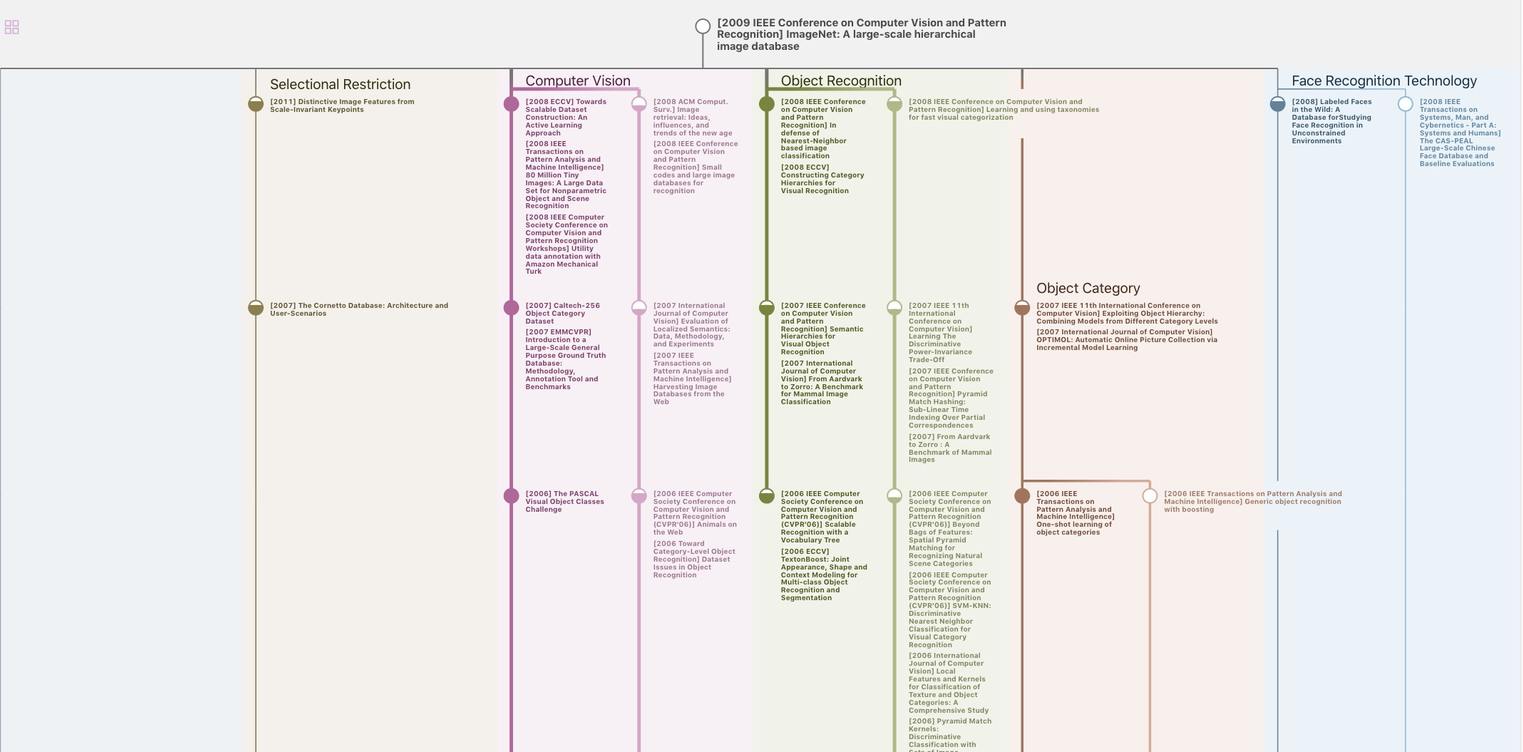
生成溯源树,研究论文发展脉络
Chat Paper
正在生成论文摘要