Extreme Phenotype Sampling Improves LASSO and Random Forest Marker Selection for Complex Traits.
BIBM(2020)
摘要
Most attempts to fit a supervised machine learning (ML) model in bioinformatics try to predict the full range of trait or response values. While such prediction tasks effectively capture the entire phenotypic range of the samples, they are cost prohibitive and can be statistically underpowered for detection of rare variants. In a study design known as extreme phenotype sampling (EPS), samples are selected from the two extremes of the phenotypic distribution. This approach is costcutting, by reducing genotyping/sequencing costs, as well as capable of increasing statistical power. Although combining EPS with ML algorithms has the potential to enhance association studies by improving their computational efficiency, EPS-ML approaches have seen limited use. In this paper we demonstrate an efficient and effective approach to leverage the EPS study design using LASSO regression and random forests, two commonly used ML algorithms within the broader bioinformatics community. We analyze two distinct data sets: leaf expression values generated from black cottonwood and malaria parasite transcriptome data collected from patients. We demonstrate that focusing only on the phenotypic extremes of these sample sets (by forming binary classes) can select more biologically meaningful features than using the full range. This approach will be useful to investigators when examining complex or novel traits. It is particularly well-suited to RNA-seq data where investigators often want to narrow attention to a small number of candidate transcripts out of a large initial pool. Our approach intentionally leverages existing software with efficient implementations to enable future applications of EPS-ML. 1
更多查看译文
关键词
random forest marker selection,lasso,phenotype
AI 理解论文
溯源树
样例
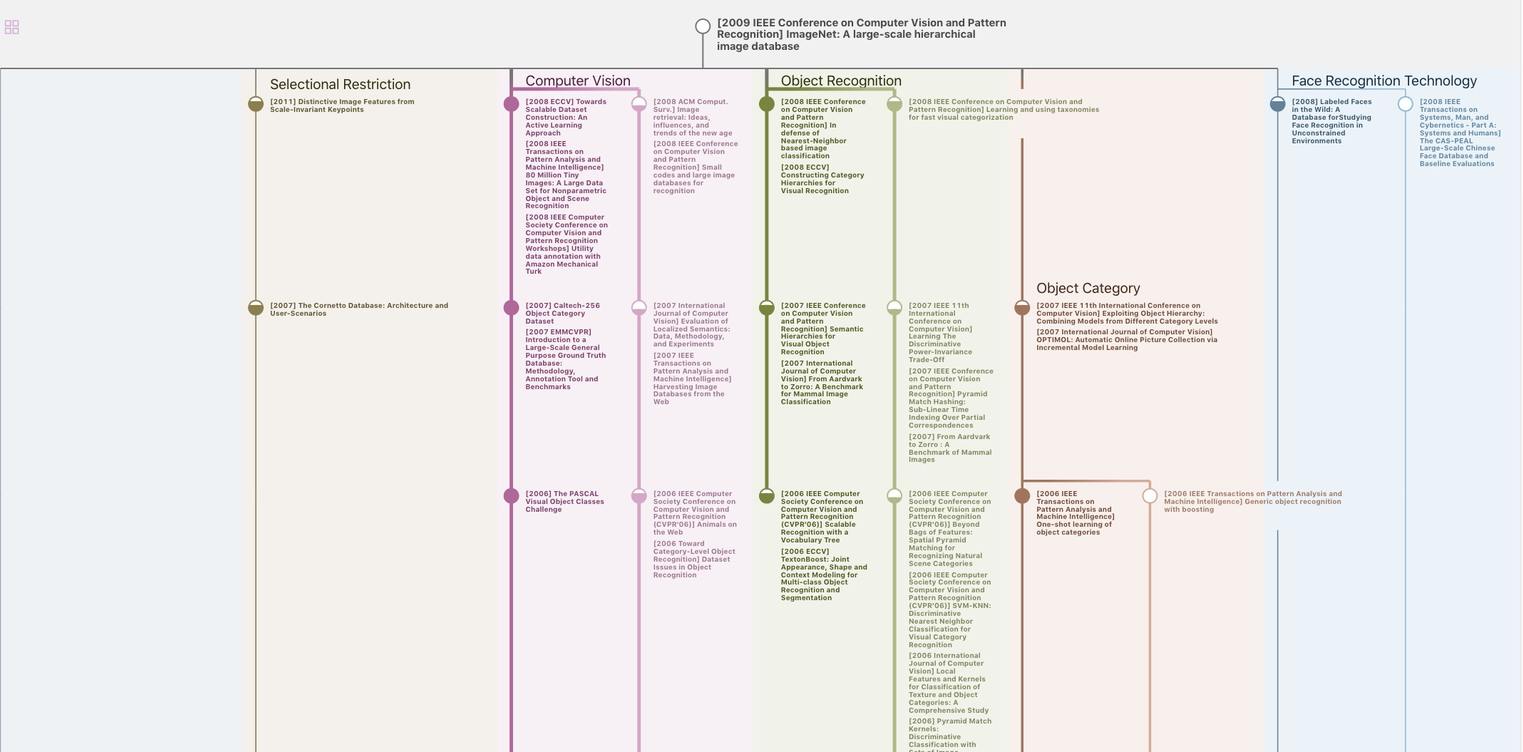
生成溯源树,研究论文发展脉络
Chat Paper
正在生成论文摘要