Improved Phishing Detection Algorithms using Adversarial Autoencoder Synthesized Data
2020 IEEE 45th Conference on Local Computer Networks (LCN)(2020)
摘要
Malicious actors often use phishing attacks to compromise legitimate users’ credentials. Machine learning is a promising approach for phishing detection. While the accuracy of machine learning algorithms is often dependent on the training data, very little attack data for training is available. We propose an approach for augmenting existing datasets that can be used by machine learning algorithms. We use an Adversarial Autoencoder (AAE) to generate samples that mimic the phishing websites and provide metrics to assess the quality of the generated samples. We test these samples against models trained with real-world data. Some of generated samples are able to evade existing detection model. We then use a portion of these samples in training. The new machine learning models are more robust and have higher accuracy. In other words, real-world phishing site data augmented with AAE synthesized data used for training the model is more effective for phishing detection.
更多查看译文
关键词
phishing detection,malicious actors,phishing attacks,attack data,Web sites,real-world data,machine learning,real-world phishing site data,AAE synthesized data,adversarial autoencoder synthesized data
AI 理解论文
溯源树
样例
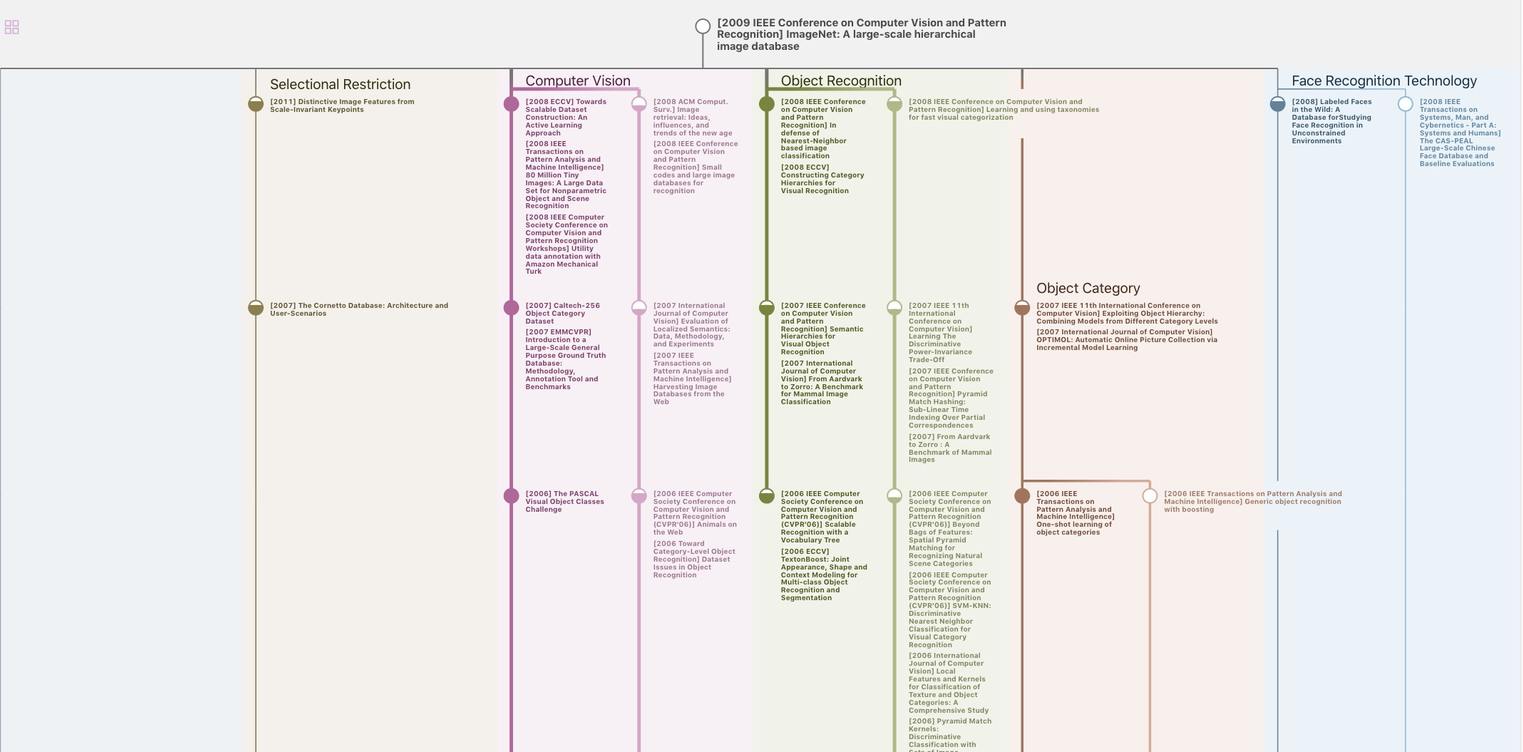
生成溯源树,研究论文发展脉络
Chat Paper
正在生成论文摘要