Sparse Causal Residual Neural Network for Linear and Nonlinear Concurrent Causal Inference and Root Cause Diagnosis
16TH IEEE INTERNATIONAL CONFERENCE ON CONTROL, AUTOMATION, ROBOTICS AND VISION (ICARCV 2020)(2020)
摘要
Reliable and effective fault diagnosis methods are necessary for complex industrial processes that consists of various units. After a process fault is detected, it remains a challenging task to locate the root cause unit and determine the propagation path of the fault. In this paper, a novel method, termed Sparse Causal Residual Neural Network (SCRNN), is proposed and applied for modern industrial root cause diagnosis. The advantage of SCRNN lies in that it can not only recognize linear and nonlinear causal relationships in parallel, but also automatically determine the causality lags and deduce the time delay of causal transmission. Besides, due to the specially designed sparse constraint and optimization algorithm, the SCRNN model can realize the function of key dependent variable selection, avoiding the high computational complexity and complicated procedure brought by pairwise comparison. The feasibility of the proposed method is illustrated through the benchmark TE process.
更多查看译文
关键词
reliable fault diagnosis methods,complex industrial processes,root cause unit,propagation path,nonlinear causal relationships,optimization algorithm,SCRNN model,benchmark TE process,sparse causal residual neural network,nonlinear concurrent causal inference,linear concurrent causal inference,industrial root cause diagnosis,key dependent variable selection
AI 理解论文
溯源树
样例
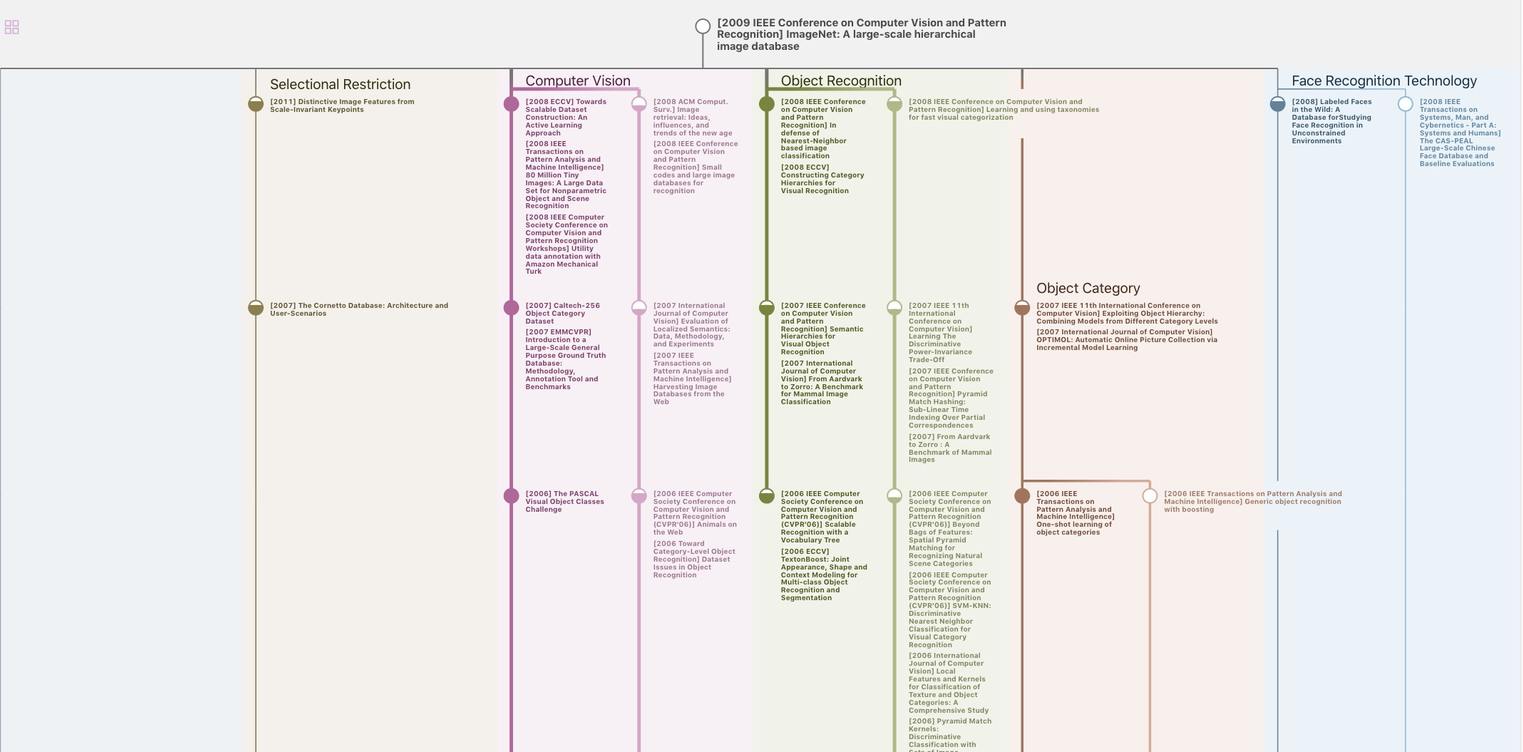
生成溯源树,研究论文发展脉络
Chat Paper
正在生成论文摘要