Electricity Load Forecasting with Collective Echo State Networks
2020 IEEE International Conference on Communications, Control, and Computing Technologies for Smart Grids (SmartGridComm)(2020)
摘要
Short-term load forecasting (STLF) is one of the most important tasks in power grid systems. Despite thorough research of STLF on community scales, predicting individual power consumption remains challenging. With the deployment of advanced metering infrastructure (AMI) technology, it is more convenient to access and operate load usage data on an individual scale. This work first investigates a novel deep learning method, Collective Long Short-term Memory (LSTM), which can fully utilize correlated meter information from AMI. Then, Collective Echo State Networks (ESN) are proposed as a simplified form of Collective LSTM using the reservoir method. Experimental results show that Collective ESN can achieve state-of-the-art prediction accuracy with extremely fast speed and fewer computational resources.
更多查看译文
关键词
electricity load forecasting,short-term load forecasting,STLF,power grid systems,community scales,individual power consumption,advanced metering infrastructure technology,AMI,load usage data,individual scale,deep learning method,correlated meter information,prediction accuracy,collective echo state networks,collective long short-term memory,collective LSTM,collective ESN
AI 理解论文
溯源树
样例
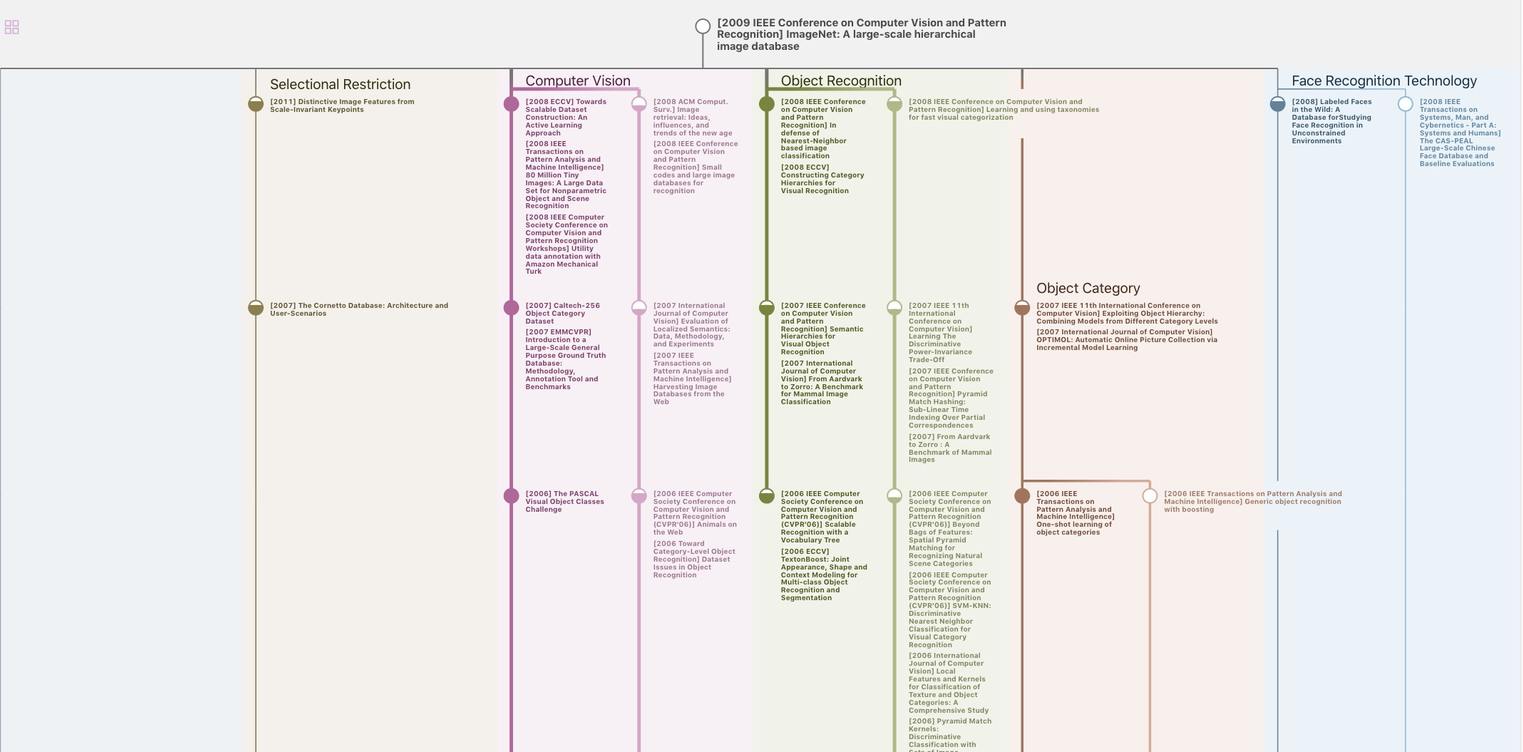
生成溯源树,研究论文发展脉络
Chat Paper
正在生成论文摘要