FedMax: Enabling a Highly-Efficient Federated Learning Framework
2020 IEEE 13th International Conference on Cloud Computing (CLOUD)(2020)
摘要
IoT devices produce a wealth of data desired for learning models to empower more intelligent applications. However, such data is often privacy sensitive making data owners reluctant upload their data to a central server for learning purposes. Federated learning provides a promising privacy-preserving learning approach, which decouples the model training from the need of accessing to the sensitive data. However, realizing a deployed, dependable federated learning system faces critical challenges, such as frequent dropouts of learning workers, heterogeneity of workers computation, and limited communication. In this paper, we focus on the systems aspects to advance federated learning and contribute a highly efficient and reliable distributed federated learning framework, FedMax, aiming to tackle these challenges. In designing FedMax, we contribute new techniques in light of the properties of a real federated learning setting, including a relaxed synchronization communication scheme and a similarity-based worker selection approach. We have implemented a prototype of FedMax and evaluated FedMax upon multiple popular machine learning models and datasets, showing that FedMax significantly increases the robustness of a federated learning system, speeds up the convergence rate by 25%, and increases the system efficiency by 50%, in comparison with state-of-the-art approaches.
更多查看译文
关键词
Federated learning,distributed systems,machine learning,privacy-preserving
AI 理解论文
溯源树
样例
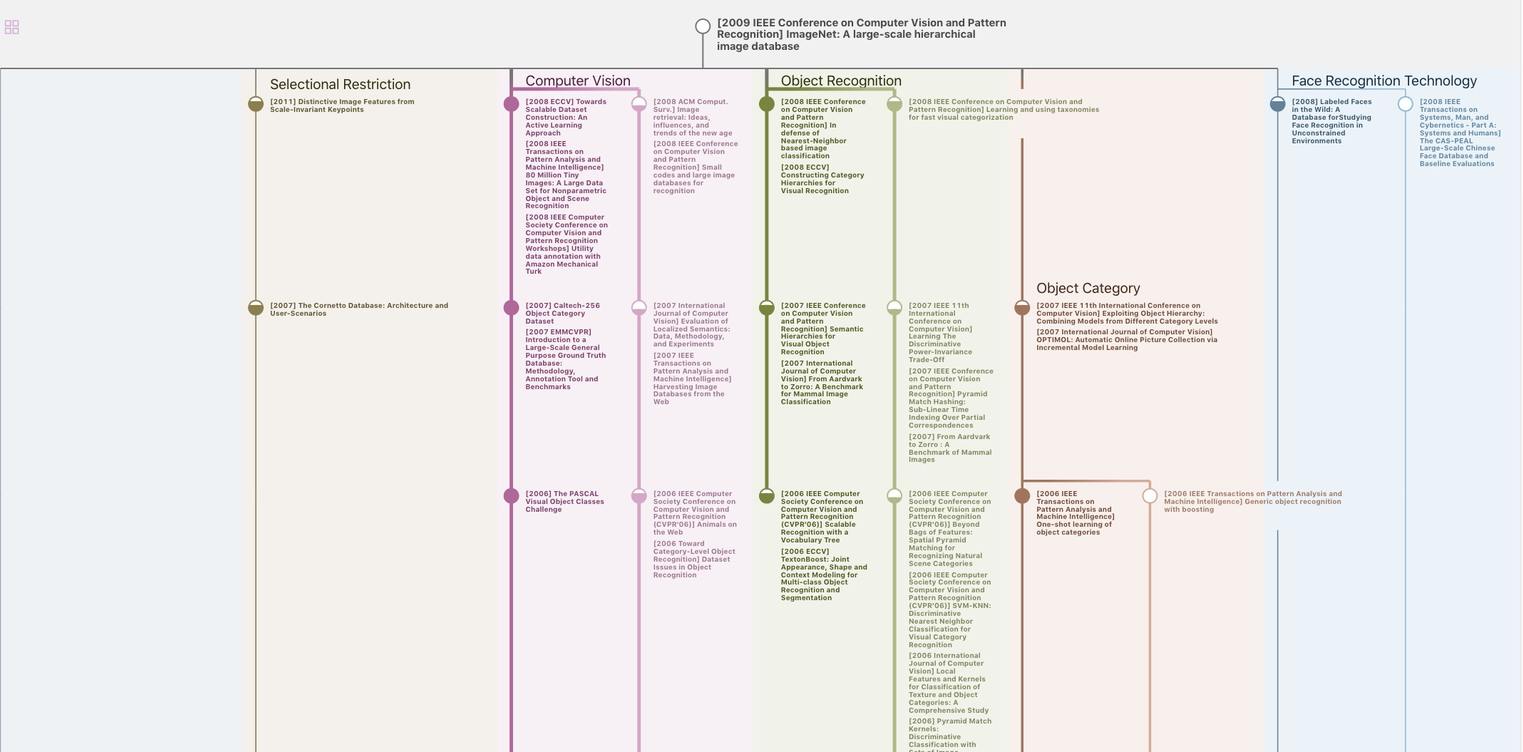
生成溯源树,研究论文发展脉络
Chat Paper
正在生成论文摘要