Modelling VM Latent Characteristics and Predicting Application Performance using Semi-supervised Non-negative Matrix Factorization
2020 IEEE 13th International Conference on Cloud Computing (CLOUD)(2020)
摘要
Selecting a suitable VM instance type for an application can be a difficult task because of the number of options and the variety of application requirements. Recent research takes a data-driven approach to model VM performance, but this requires carefully choosing a small set of relevant benchmarks as input. We propose a semi-supervised matrix-factorization-based latent variable approach to predict the performance of an unknown new application. This method allows to take a large set of benchmarks as input for VM performance modelling, and it uses the model and the performance measure of the new application on some of the target VMs to predict the performance on the rest of all VMs. We ran experiments with 373 micro-benchmarks from stress-ng and 37 AWS EC2 VMs to predict the scores of Geekbench accurately. Our initial results showed that the RMSE and STD of the predicted scores are 6.7 and 4.5 when sampling Geekbench on 5 VMs, and 10.0 and 2.8 when sampling 10.
更多查看译文
关键词
Cloud Computing,Matrix Factorization,Latent Variable Model,Micro-Benchmarks
AI 理解论文
溯源树
样例
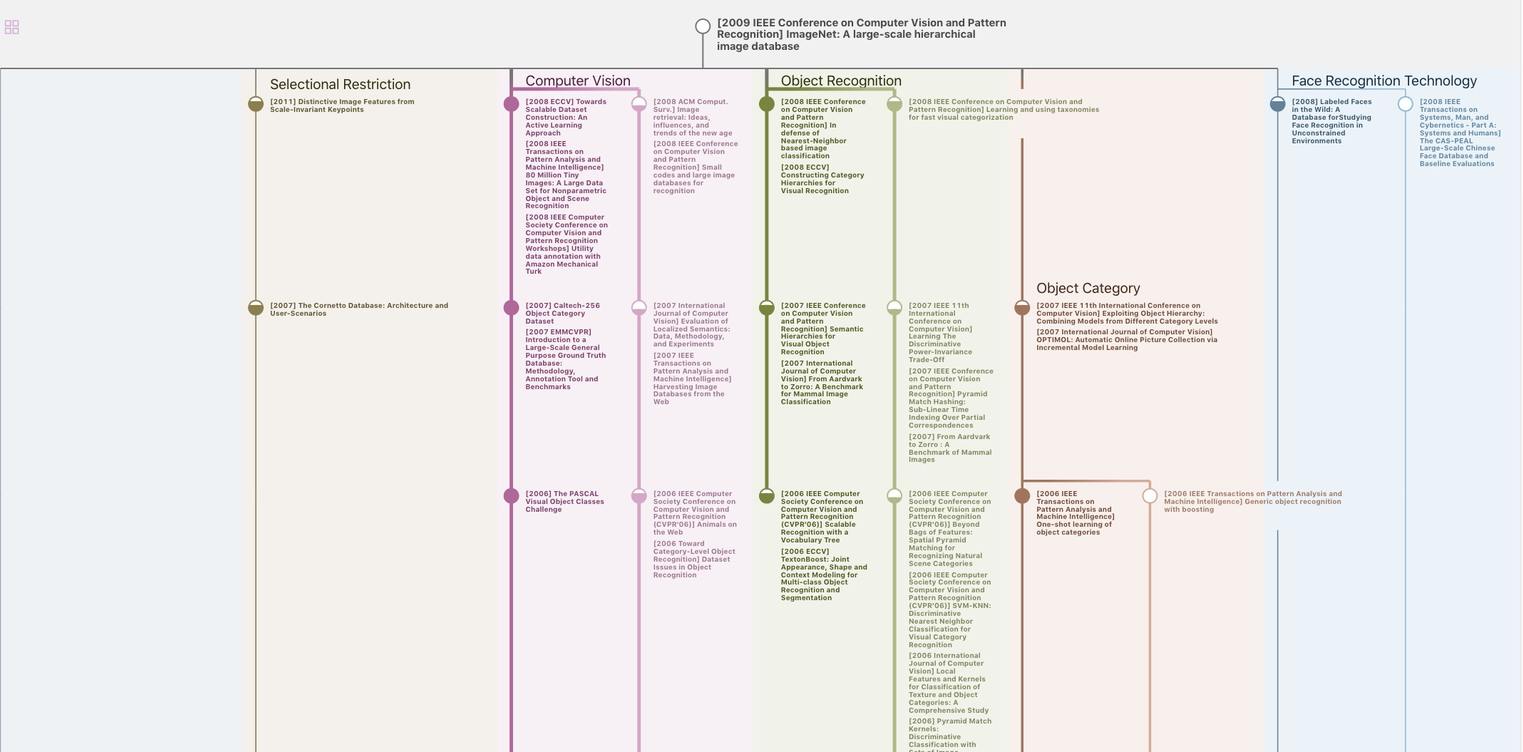
生成溯源树,研究论文发展脉络
Chat Paper
正在生成论文摘要