Enhanced Sampling of Nucleic Acids' Structures Using Deep-Learning-Derived Biasing Forces.
SSCI(2020)
摘要
The conformation spaces (CS) of macromolecules and their associated dynamics are of vital importance in the understanding of many biochemical functions as well as diseases and in the developments of drugs for curing or managing disease conditions. While the exploration of the CS is generally easier for molecules with fewer atoms (such as ligands and short peptides), achieving the same for larger molecules (such as nucleic acids and proteins) beyond a narrow local equilibrium is non-trivial and sometimes computationally prohibitive. In this work, we present Deep Enhanced Sampling of Nucleic Acids' Structures Using Deep-Learning-Derived Biasing Forces (DESNA, pronounced DES-na), that combines variational autoencoder, a special deep neural network (DNN), and molecular dynamics (MD) simulations to create a robust technique for enhanced sampling, in which DNN-learned latent space is used for inferring appropriate biasing potentials for guiding the MD simulations. The results obtained show that DESNA performs better than conventional MD simulations and efficiently samples wider CS than conventional MD simulations even when DESNA is allowed to run for as short as 10% of the length of conventional MD simulations. This suggests that DESNA is at least 10 times more efficient that conventional MD simulations in its sampling of CS of molecules.
更多查看译文
关键词
Deep neural network,variational autoencoder,conformation space,molecular dynamics,nucleic acids,DNA
AI 理解论文
溯源树
样例
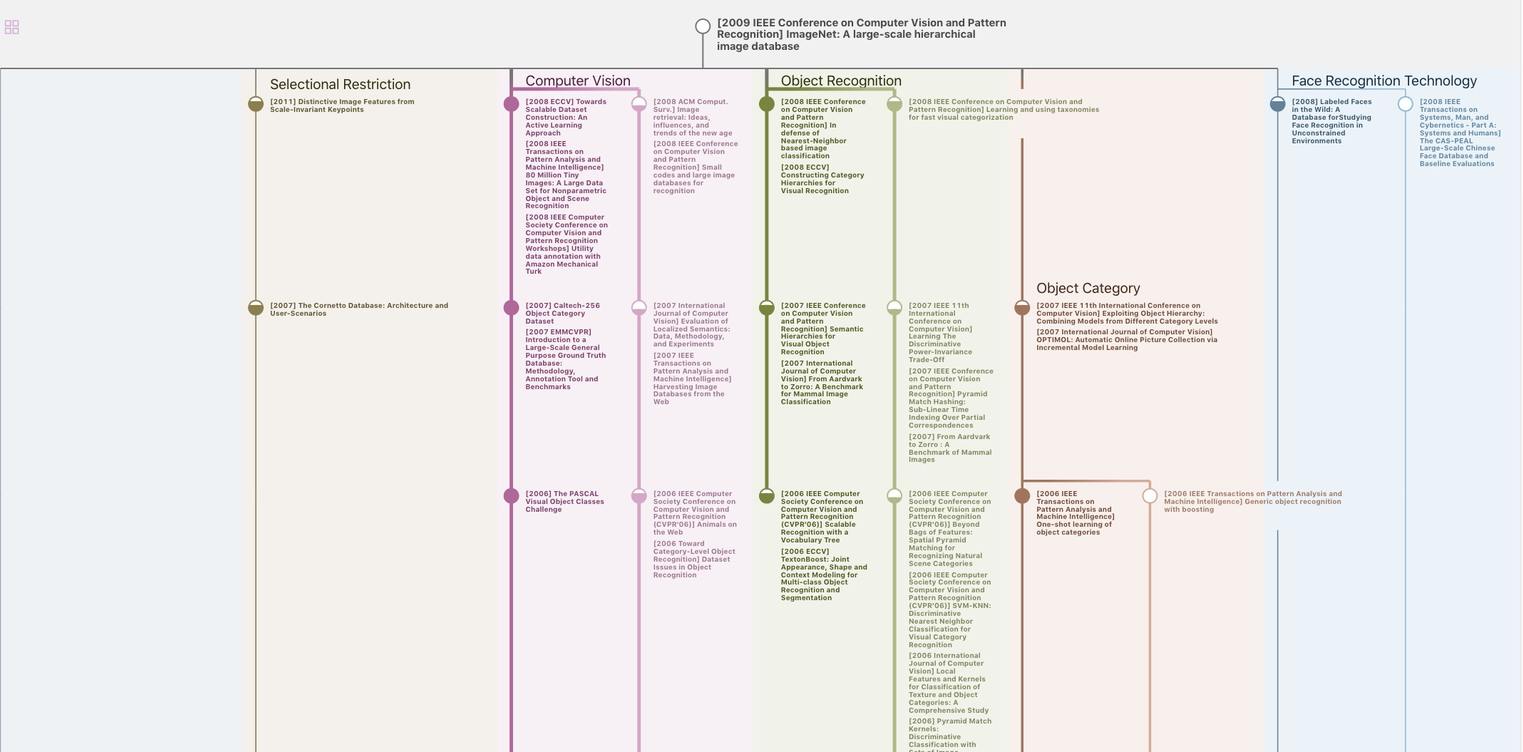
生成溯源树,研究论文发展脉络
Chat Paper
正在生成论文摘要