Statistical Tests Ensemble Drift Detector.
SSCI(2020)
摘要
Several classifiers use supervised inductive learning and, to improve accuracy, they are often combined with concept drift detectors. The ideal learning algorithm associates robustness to noise with sensitivity to concept drifts. Motivated by these statements, this paper proposes a concept drift detector aiming to validate empirically the idea of implementing a drift detection method based on the combination of statistical tests as a viable option to improve the classification. The Statistical Tests Ensemble Detector (STED) uses the results of Brown-Forsythe, O'Brien, and ANOVA statistical tests combined by two voting strategies. To signal warnings, the majority vote is used with the results of the three tests, and, to detect concept drifts, the "Early-find-early-report" rule is adopted with the results of the Brown-Forsythe and O'Brien tests only. The experiments' results using Hoeffding Tree (IIT) as base learner in 24 artificial and seven real-world datasets corroborates the efficiency of this proposal: STED achieved the best accuracies and was balanced in the detections of drifts, based on its second position in the evaluation using the Matthews Correlation Coefficient (MCC).
更多查看译文
关键词
Concept Drift, Ensemble of statistical tests, Drift detection, Data stream, Online Learning
AI 理解论文
溯源树
样例
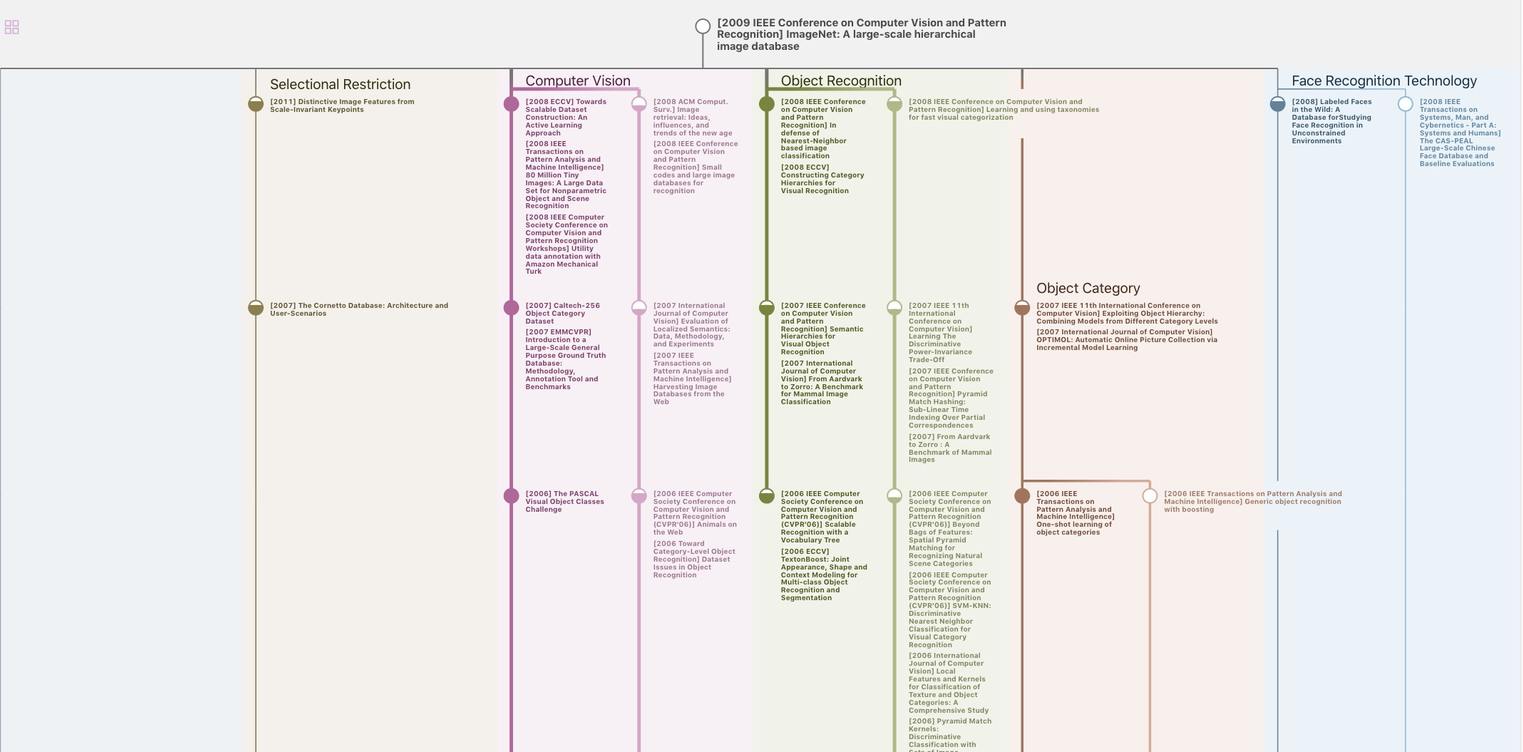
生成溯源树,研究论文发展脉络
Chat Paper
正在生成论文摘要