Towards Efficient Neural Network on Edge Devices via Statistical Weight Pruning.
GCCE(2020)
摘要
Convolutional neural network (CNN) becomes more and more popular as it has demonstrated great success in many visual content-oriented applications such as computer vision and image/video processing. Inevitably, massive computation resources and storage spaces are required for the hardware implementations of CNN. While “model compression” can significantly reduce parameters (e.g., weights) used in CNN so as corresponding computations by suitable pruning, this paper proposes a pruning approach based on statistical analysis of weight distribution. Experimental results show that substantial redundant weights of a demonstrated CNN were effectively removed through carefully tracing weight distributions for each layer. Meanwhile, saving weight is expected to save up to 30% of storage space and computation complexity.
更多查看译文
关键词
convolutional neural network (CNN),compression,storage space,pruning
AI 理解论文
溯源树
样例
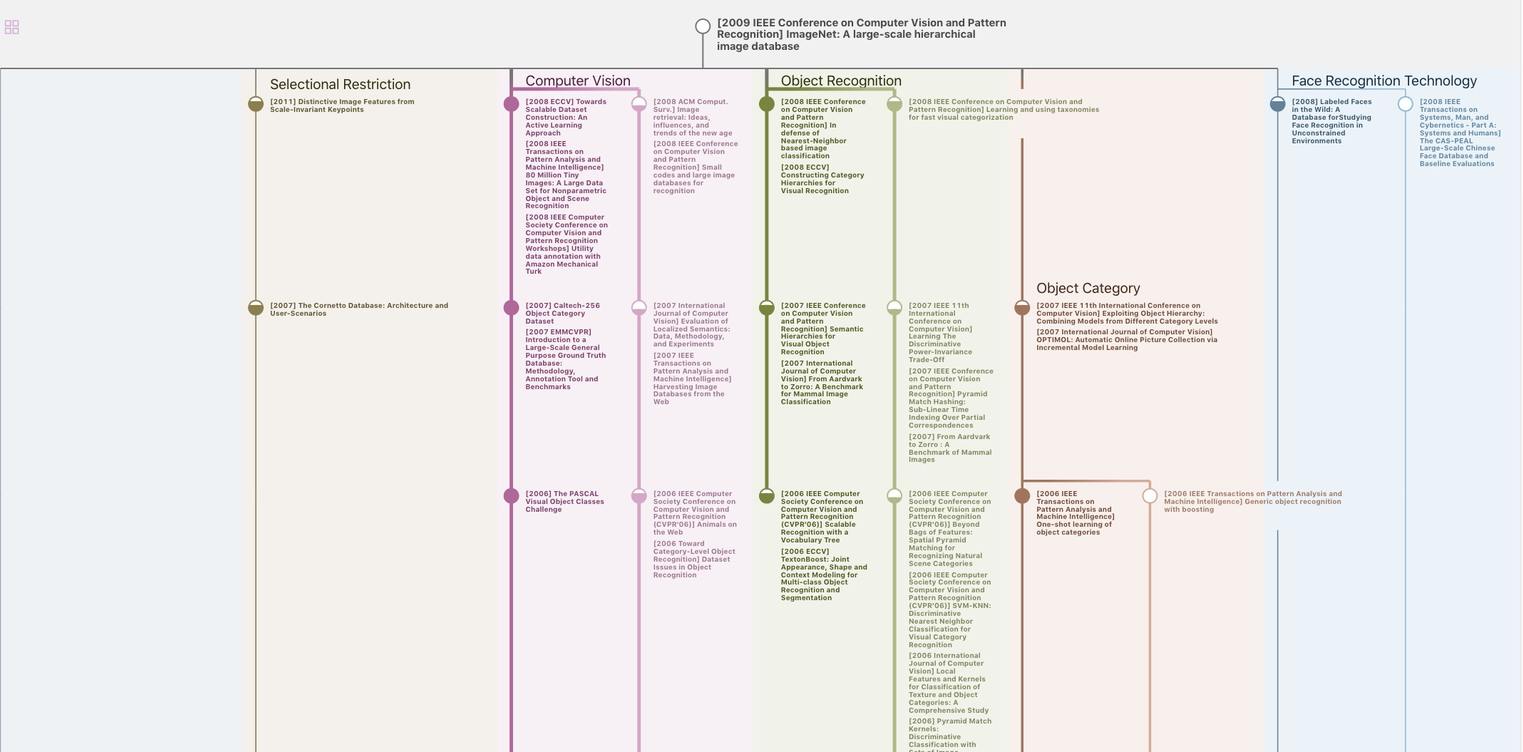
生成溯源树,研究论文发展脉络
Chat Paper
正在生成论文摘要