A Learning Approach for Optimizing Robot Behavior Selection Algorithm.
ICIRA(2020)
摘要
Algorithms are the heart of each robotics system. A specific class of algorithm embedded in robotics systems is the so-called behavior - or action - selection algorithm. These algorithms select an action a robot should take, when performing a specific task. The action selection is determined by the parameters of the algorithm. However, manually choosing a proper configuration within the high-dimensional parameter space of the behavior selection algorithm is a non-trivial and demanding task. In this paper, we show how this problem can be addressed with supervised learning techniques. Our method starts by learning the algorithm behavior from the parameter space according to environment features, then bootstrap itself into a more robust framework capable of self-adjusting robot parameters in real-time. We demonstrate our concept on a set of examples, including simulations and real world experiments.
更多查看译文
关键词
Robot learning, Behavior selection, Parameter optimization
AI 理解论文
溯源树
样例
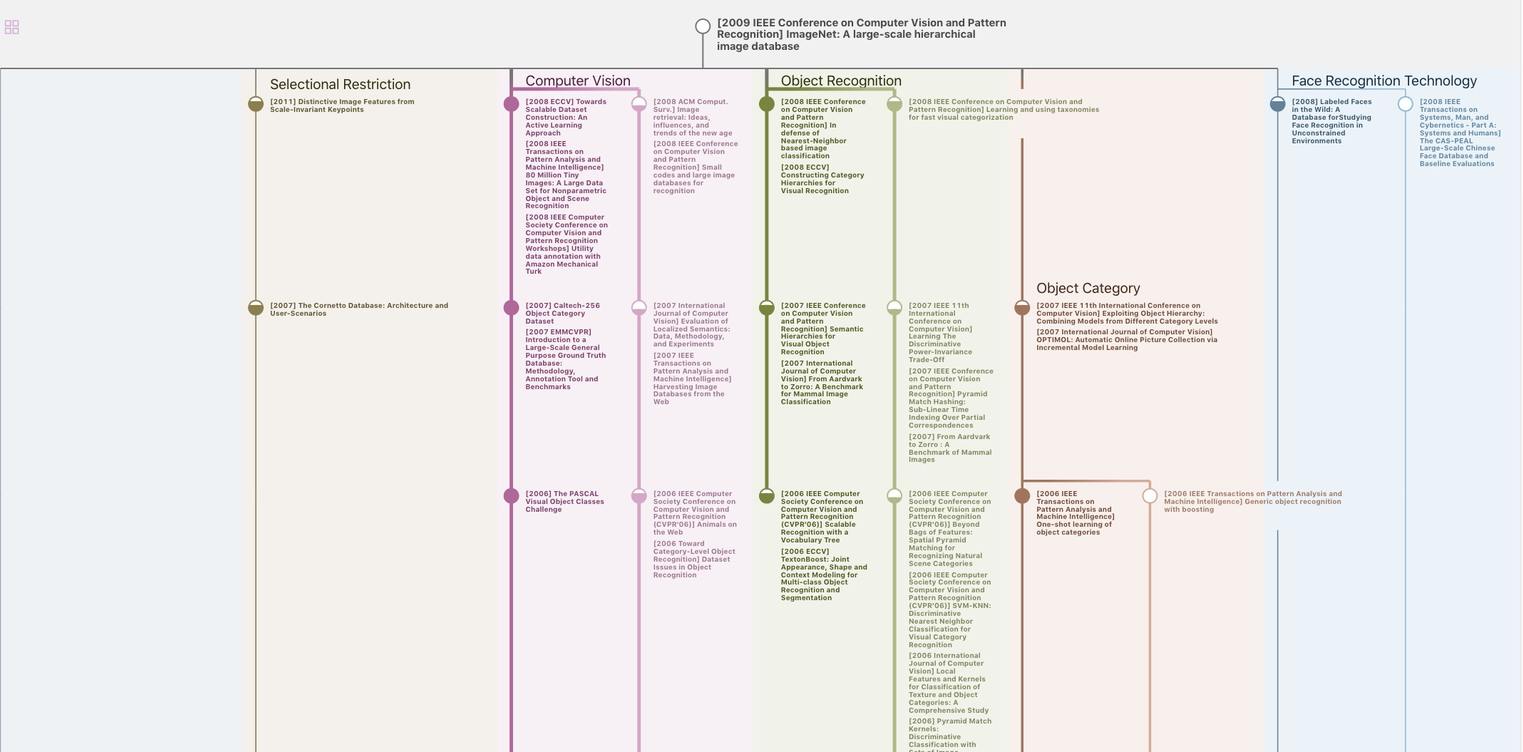
生成溯源树,研究论文发展脉络
Chat Paper
正在生成论文摘要