Ambient Pain Monitoring in Older Adults with Dementia to Improve Pain Management in Long-Term Care Facilities
ICMI-MLMI(2020)
摘要
ABSTRACTPainful conditions are prevalent in older adults, yet may go untreated, especially in people with severe dementia who often cannot verbally communicate their pain. Not addressing the pain can lead to the worsening of underlying conditions or lead to frustration and agitation. For older adults living in long-term care (LTC) facilities, timely assessment of pain remains a challenge. The main reasons for this are staff shortages at these facilities and/or insufficient expertise in cutting-edge pain assessment methods reliant on non-verbal cues. Ambient monitoring of non-verbal cues of pain, e.g. facial expressions, body movements, or vocalizations, is a promising avenue to improve pain management in LTC. Despite extensive existing research in computer vision algorithms for pain detection, the currently available techniques and models are not ready or directly applicable for use in LTC settings. Publicly available video datasets used for training and validating pain detection algorithms (e.g. the UNBC-McMaster Shoulder Pain Expression Archive Database and the BioVid Heat Pain Database) do not include older adults with dementia. Facial analysis models that are trained and validated on data from healthy and primarily young adults are known to under-perform, sometimes drastically, when tested on faces of older adults with dementia. As such, the performance of existing pain detection models on the dementia population remains to be validated. Furthermore, in existing datasets, participants are well-lit and face the camera; so the developed algorithm's performance may not transfer to a realistic ambient monitoring setting. In this work, we make three main contributions. First, we develop a fully-automated pain monitoring system (based on a convolutional neural network architecture) especially designed for and validated on a new dataset of over 162,000 video frames recorded unobtrusively from 95 older adults, of which 47 were community dwelling and cognitively healthy (age:~75.5~±~6.1), and 48 (age:~82.5~±~9.2) were individuals with severe dementia residing in LTC. Second, we introduce a data efficient pairwise training and inference method that calibrates to each individual face. Third, we introduce a contrastive training method and show that it significantly improves cross-dataset performance across UNBC-McMaster, cognitively healthy older adults, and older adults with dementia. We perform 5-fold (leave-subjects-out) cross-validation. Our algorithm achieves a Pearson correlation coefficient (PCC) of 0.48 for per-frame predictions of pain intensity and a PCC of 0.82 for predictions aggregated over 20 second windows for participants with dementia.
更多查看译文
关键词
Computer Vision, Dementia, Facial Expression, Older adults, Pain, Deep Learning, Contrastive Training
AI 理解论文
溯源树
样例
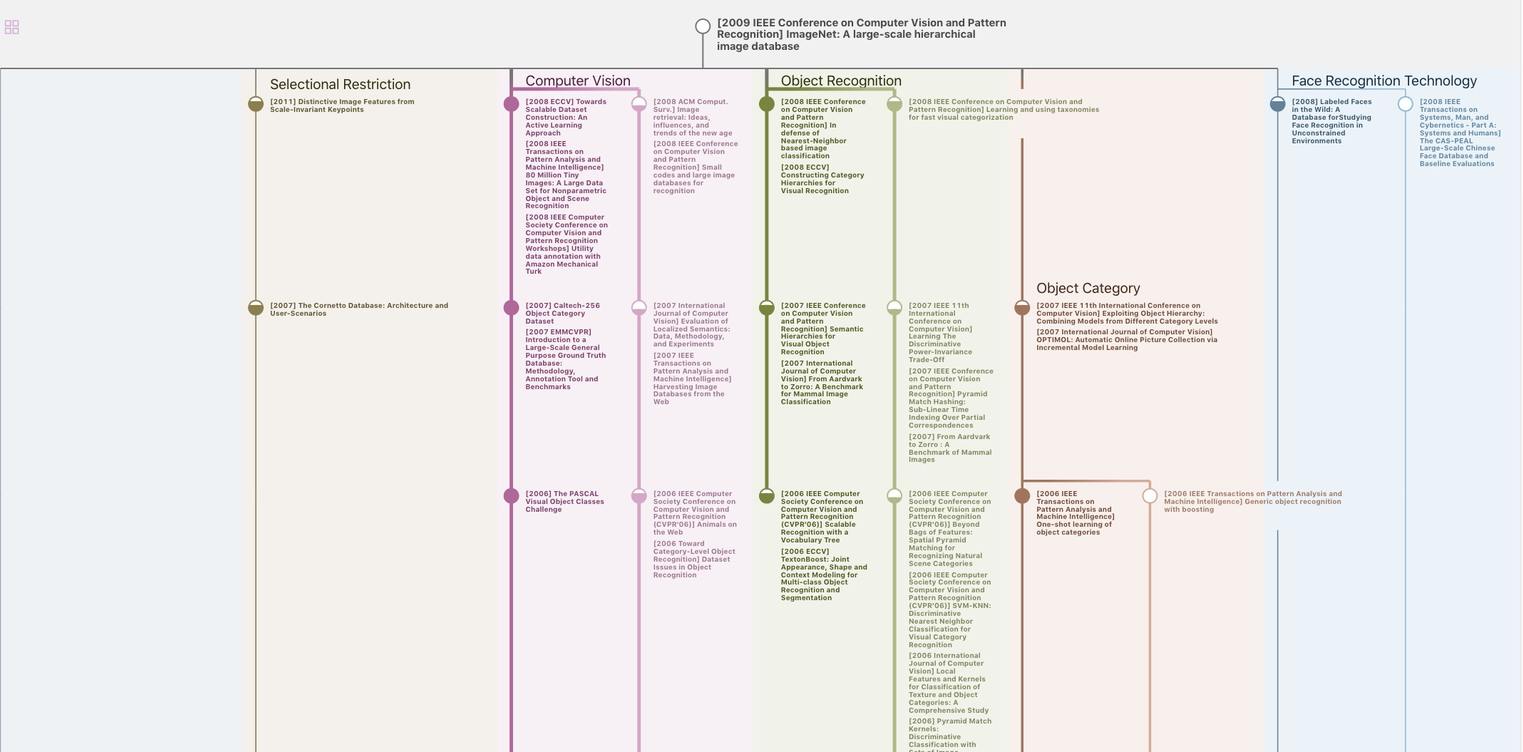
生成溯源树,研究论文发展脉络
Chat Paper
正在生成论文摘要