The Distracting Control Suite -- A Challenging Benchmark for Reinforcement Learning from Pixels
arxiv(2021)
摘要
Robots have to face challenging perceptual settings, including changes in viewpoint, lighting, and background. Current simulated reinforcement learning (RL) benchmarks such as DM Control provide visual input without such complexity, which limits the transfer of well-performing methods to the real world. In this paper, we extend DM Control with three kinds of visual distractions (variations in background, color, and camera pose) to produce a new challenging benchmark for vision-based control, and we analyze state of the art RL algorithms in these settings. Our experiments show that current RL methods for vision-based control perform poorly under distractions, and that their performance decreases with increasing distraction complexity, showing that new methods are needed to cope with the visual complexities of the real world. We also find that combinations of multiple distraction types are more difficult than a mere combination of their individual effects.
更多查看译文
关键词
distracting control suite,reinforcement learning,challenging benchmark
AI 理解论文
溯源树
样例
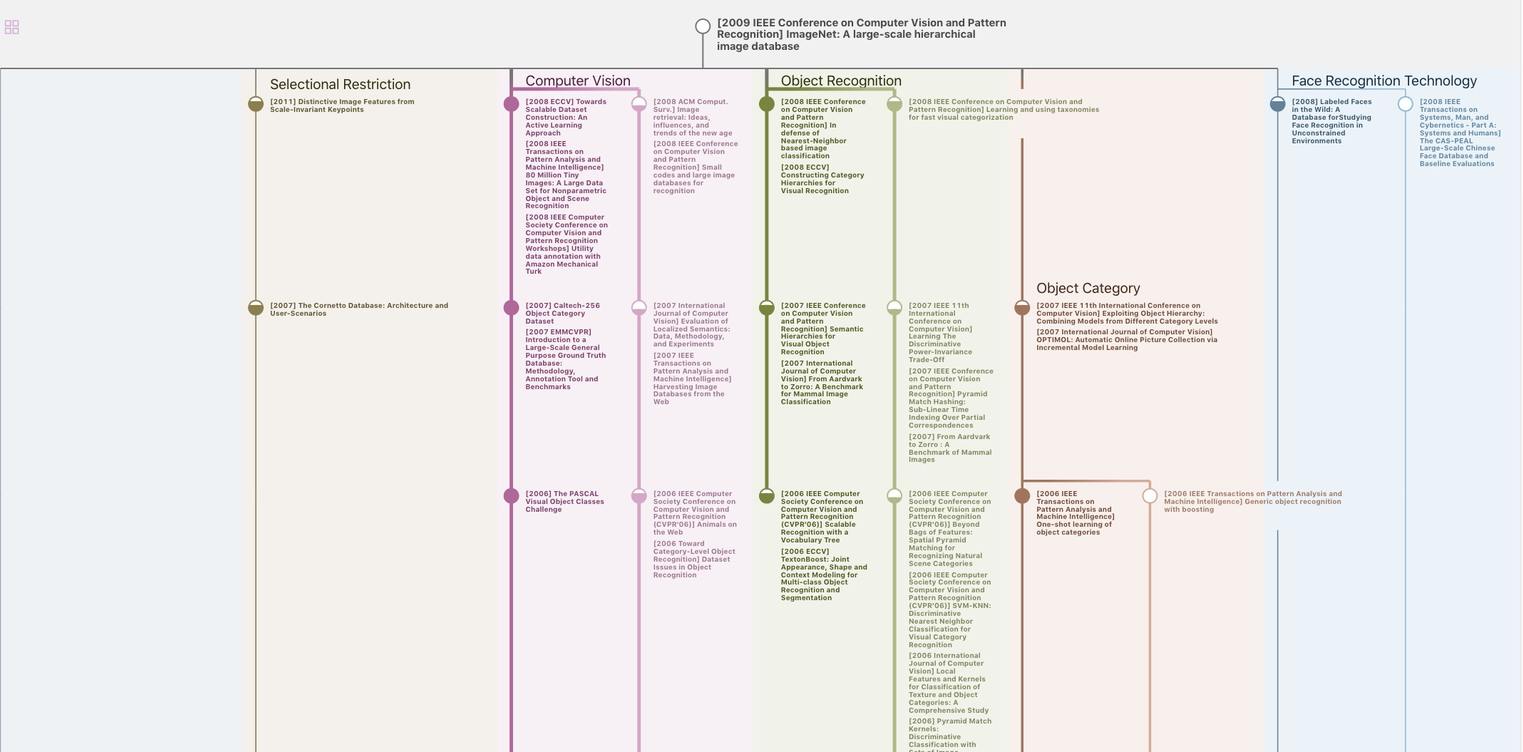
生成溯源树,研究论文发展脉络
Chat Paper
正在生成论文摘要