Hidden Markov Model Based Data-Driven Calibration Of Non-Dispersive Infrared Gas Sensor
28TH EUROPEAN SIGNAL PROCESSING CONFERENCE (EUSIPCO 2020)(2021)
摘要
Non-dispersive infrared gas sensing is one of the best gas measurement method for air quality monitoring. However, sensors drift over time due to sensor aging and environmental factors, which makes calibration necessary. In this paper, we propose a hidden Markov model approach for sensor self-calibration, which builds on the physical model of gas sensors based on the Beer-Lambert law. We focus on the statistical dependency between a calibration coefficient and the temperature change. Supervised and unsupervised learning algorithms to learn the stochastic parameters of the hidden Markov model are derived and numerically tested. The true calibration coefficient at each time instant is estimated using the Viterbi algorithm. The numerical experiments using CO2 sensor data show excellent initial results which confirms that data-driven calibration of non-dispersive infrared gas sensors is possible. Meanwhile, the challenge in the practical design is to find an appropriate quantization scheme to keep the computation burden reasonable while achieving good performance.
更多查看译文
关键词
Non-dispersive infrared gas sensor, drift, self calibration, data-driven modeling, hidden Markov model, statistical inference
AI 理解论文
溯源树
样例
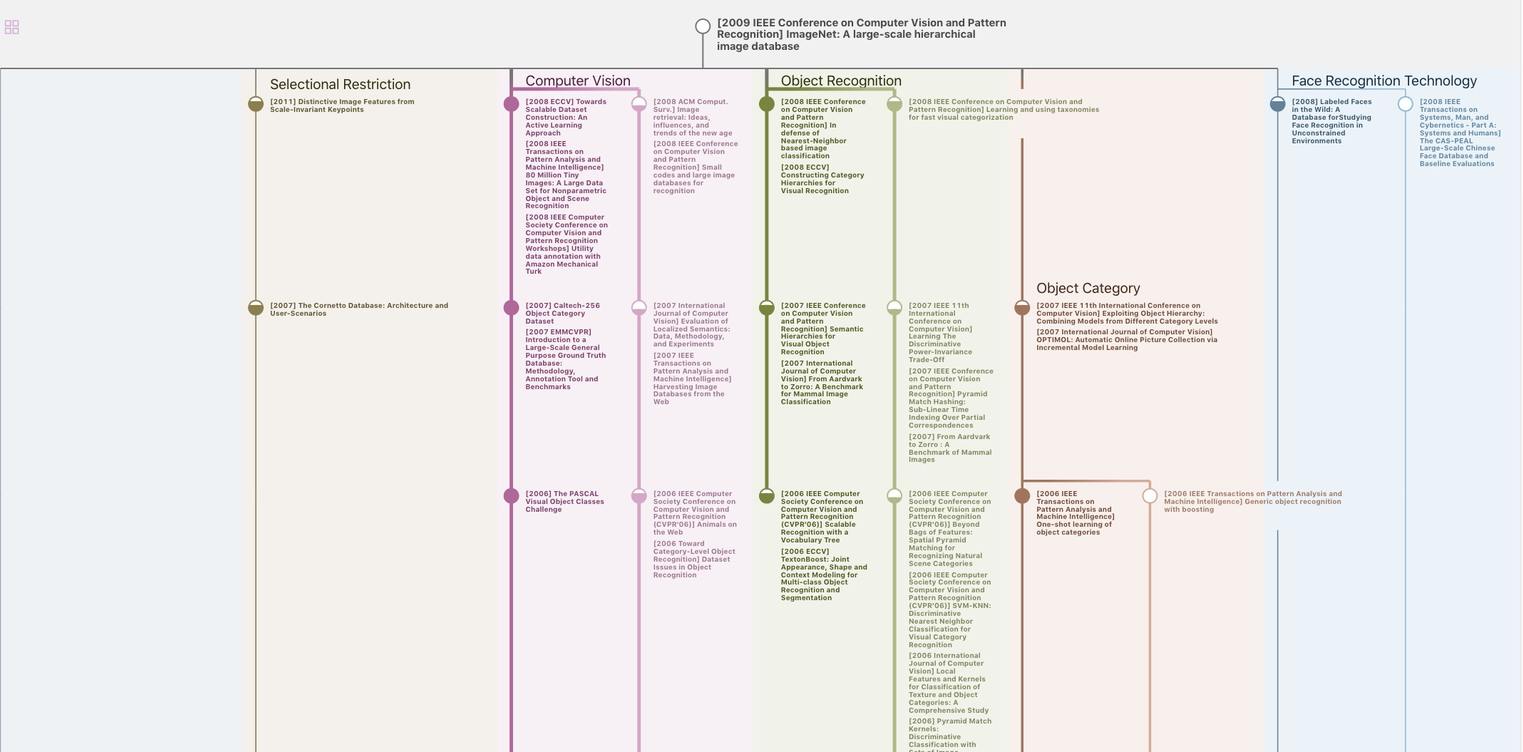
生成溯源树,研究论文发展脉络
Chat Paper
正在生成论文摘要