Selective Adaptation of End-to-End Speech Recognition using Hybrid CTC/Attention Architecture for Noise Robustness
2020 28th European Signal Processing Conference (EUSIPCO)(2021)
摘要
This paper investigates supervised adaptation of end-to-end speech recognition, which uses hybrid connectionist temporal classification (CTC)/Attention architecture, for noise robustness. The components of the architecture, namely the shared encoder, the attention decoder's long short-term memory (LSTM) layers, and the soft-max layers of the CTC part and attention part, are adapted separately or together using limited amount of adaptation data. When adapting the shared encoder, we propose to adapt only the connections of the memory cells in the memory blocks of bidirectional LSTM (BLSTM) layers to improve performance and reduce the time for adapting the models. In within-domain and cross-domain adaptation scenarios, experimental results show that adaptation of end-to-end speech recognition using the hybrid CTC/Attention architecture is effective even when the amount of adaptation data is limited. In cross-domain adaptation, substantial performance improvement can be achieved with only 2.4 minutes of adaptation data. In both adaptation scenarios, adapting only the memory cells of the BLSTM layers in the shared encoder yields comparable or slightly better performance while yielding smaller adaptation time than the adaptation of other components or the whole architecture, especially when the amount of adaptation data is less than or equal to 10 minutes.
更多查看译文
关键词
End-to-end speech recognition,noise robustness,adaptation,connectionist temporal classification,attention
AI 理解论文
溯源树
样例
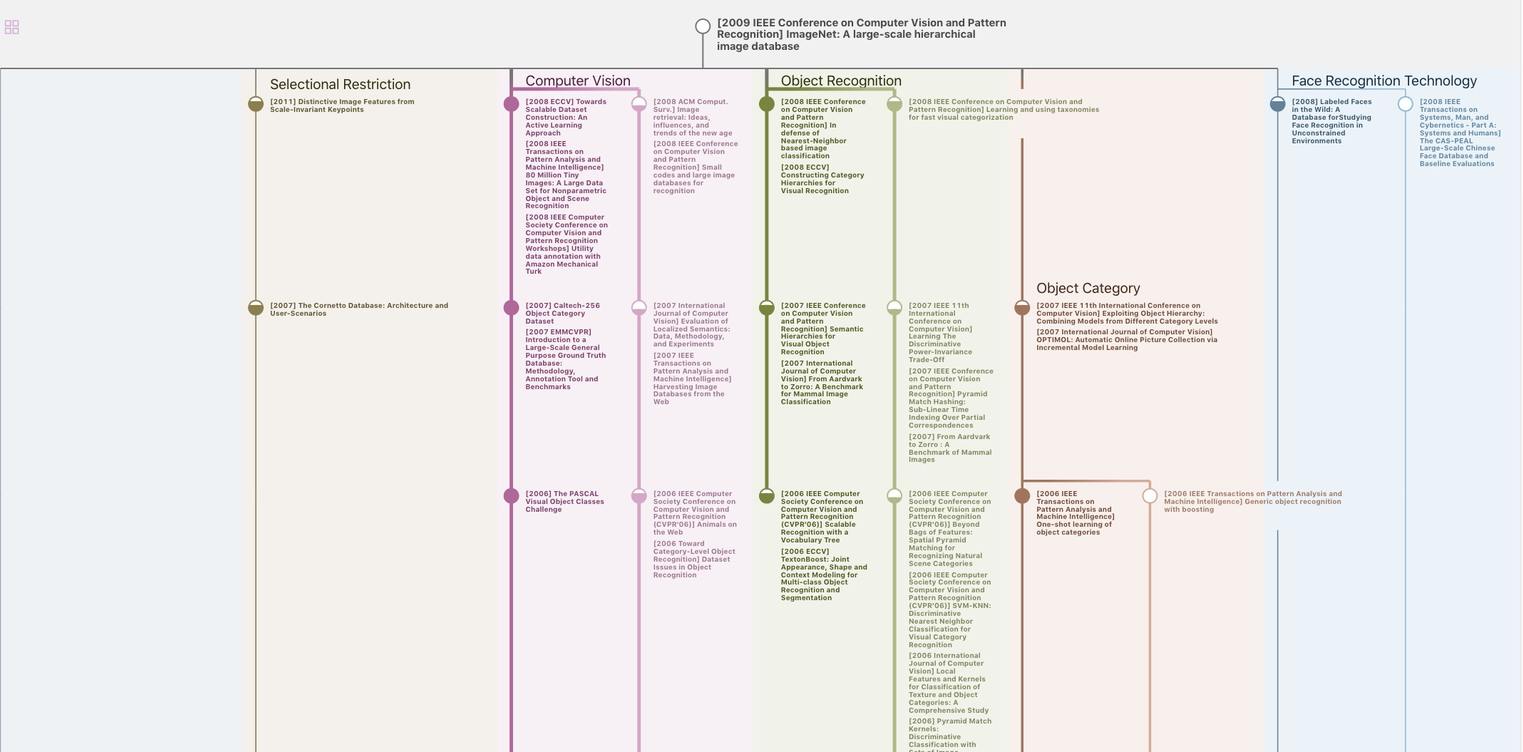
生成溯源树,研究论文发展脉络
Chat Paper
正在生成论文摘要