Towards a Smart Opponent for Board Games - Learning beyond Simulations.
SMC(2020)
摘要
Reinforcement learning algorithms have been successfully trained for games like GO, Atari, and Chess in simulated environments. However, in cue sport-based games like Carrom, real world is unpredictable unlike in Chess and GO due to the stochastic nature of the gameplay as well as the effect of external factors such as friction combined with multiple collisions. Hence, solely training in a simulated platform for games like Billiard and Carrom, which need precise execution of a shot, would not be ideal in actual gameplay. This paper presents a real-time vision based efficient robotic system to play Carrom against a proficient human opponent. We demonstrate the challenges of adopting a reinforcement learning algorithm beyond simulations in implementing a strategic gameplay for the robotic system. We currently achieve an overall shot accuracy of 70.6% by combining heuristic and reinforcement learning algorithms. Analysis of the overall results suggests the possibility of adopting a real-world training for board games which need precise mechanical actuation beyond simulations.
更多查看译文
关键词
Robotic systems,Machine vision,Image processing,Machine learning,Heuristic algorithms
AI 理解论文
溯源树
样例
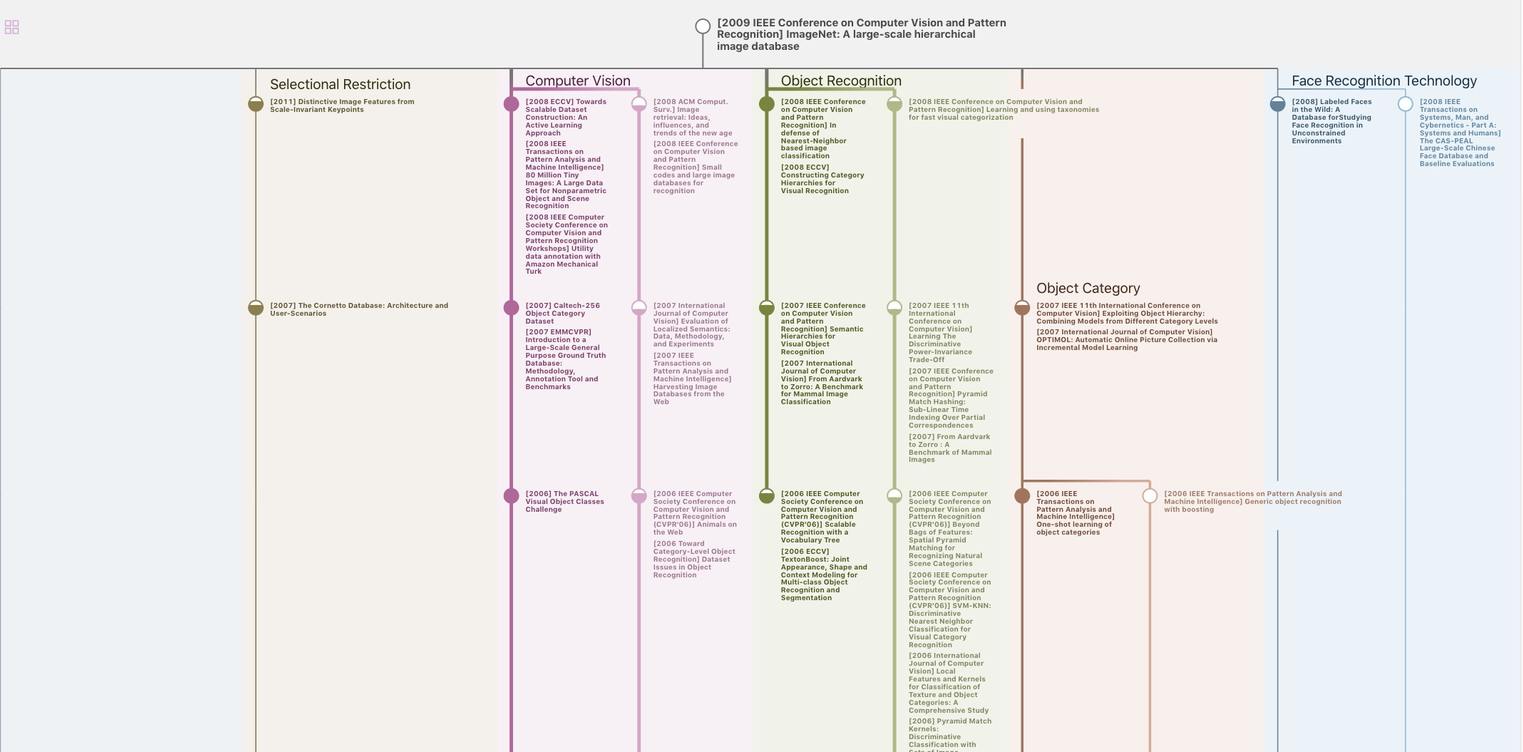
生成溯源树,研究论文发展脉络
Chat Paper
正在生成论文摘要