Effective Linear Policy Gradient Search Through Primal-Dual Approximation
2020 INTERNATIONAL JOINT CONFERENCE ON NEURAL NETWORKS (IJCNN)(2020)
摘要
Recent research discovered that Reinforcement Learning (RL) algorithms with simple linear policies can achieve competitive performance as many state-of-the-art RL algorithms designed to train policies in the form of multi-layer neural networks. However, high learning performance is only achieved so far when policies are trained by jointly using multiple episodes of samples. An important research question remains as to whether linear policies can achieve cutting-edge performance when they are trained in a step-wise fashion (i.e., policies are iteratively updated based on every newly obtained sample). This paper presents a confirmatory answer to this question by developing a new RL algorithm called Primal-Dual Regular-gradient Actor-Critic (PD-RAC) as a generalization of RAC, which is a popular step-wise RL technique. Experiments on six benchmark control problems show that PD-RAC can achieve leading performance, in comparison to several recently developed baseline algorithms.
更多查看译文
关键词
Reinforcement Learning, Actor Critic, Episodic Learning, Step-wise Learning, Primal-Dual Approximation
AI 理解论文
溯源树
样例
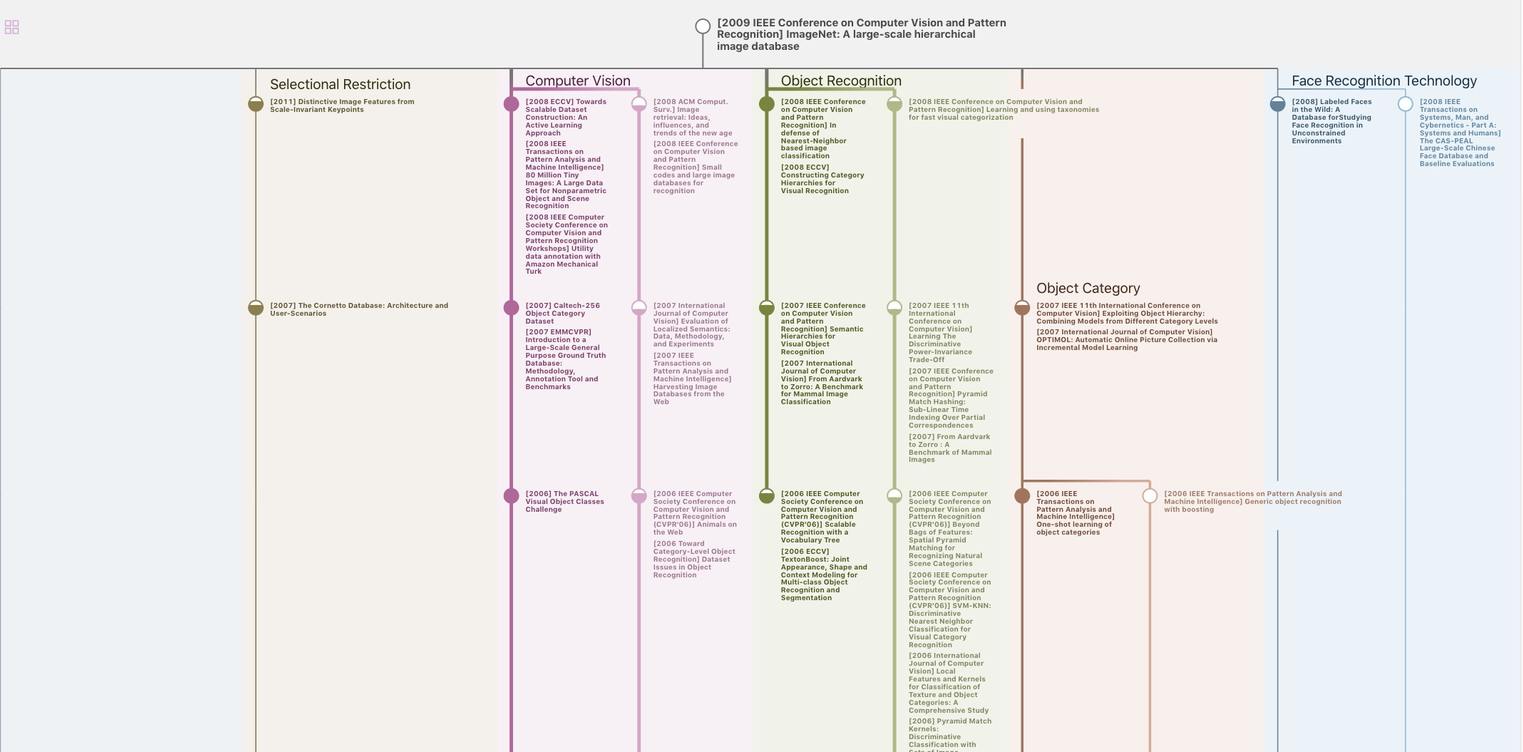
生成溯源树,研究论文发展脉络
Chat Paper
正在生成论文摘要